Stochastic filtering in jump systems with state dependent mode transitions
ACC'09 Proceedings of the 2009 conference on American Control Conference(2009)
摘要
We introduce a new methodology to construct a Gaussian mixture approximation to the true filter density in hybrid Markovian switching systems. We relax the assumption that the mode transition process is a Markov chain and allow it to depend on the actual and unobservable state of the system. The main feature of the method is that the Gaussian densities used in the approximation are selected as the solution of a convex programming problem which trades off sparsity of the solution with goodness of fit. A meaningful example shows that the proposed method can outperform the widely used interacting multiple model (IMM) filter in terms of accuracy at the expenses of an increase in computational time.
更多查看译文
关键词
main feature,state dependent mode transition,computational time,meaningful example,gaussian mixture approximation,mode transition process,jump system,markov chain,hybrid markovian,true filter density,convex programming problem,convex programming,goodness of fit,trajectory,nonlinear programming,matched filters,noise,clustering algorithms,kalman filter,approximation theory,gaussian processes,probability density function,estimation,markov processes,covariance matrix,kalman filtering,tracking,control systems,sampling methods,filtering,upper bound
AI 理解论文
溯源树
样例
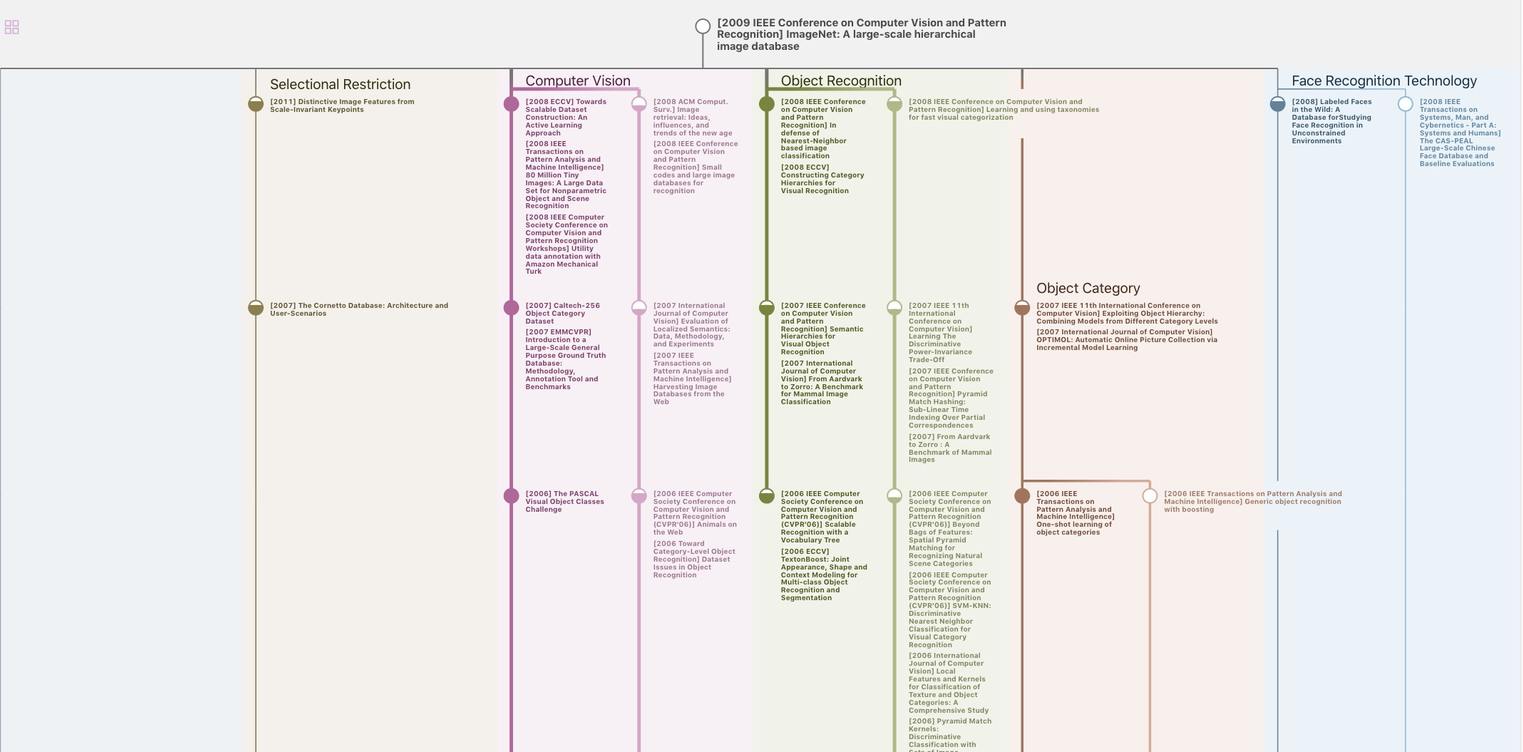
生成溯源树,研究论文发展脉络
Chat Paper
正在生成论文摘要