Classification of cancers by gene expression profiles from peripheral blood
msra(2003)
摘要
We have analyzed gene expression in peripheral blood lymphocytes from patients at early stages of solid tumors (not blood related) and normal controls. The samples from patients with solid tumors can be easily distinguished from controls by hierarchical clustering or supervised procedures including shrunken centroids or penalized discriminant analysis (PDA). In most cases, a single sample randomly picked from each group is enough to provide correct classification for the rest of the samples. In spite of the consistency of the cancer group, it has a substantially greater variance than the control group. Part of this variance stems from a variable degree of progressive failure of the immune response in cancer patients. We have estimated the disease progression by projecting each sample on the AverageControl-AverageCancer vector, by the shrunken distance to control centroid, and by crossvalidation with PDA. To identify the most significant genes we have used either the median distance from the control centroid, or discriminant loadings obtained from PDA performed on Control-Cancer groups after the outliers have been removed. Among the genes changed the most with disease progression we identified two main groups. The first group, which contains genes associated with cell growth, actin remodeling, energy production, and mitosis, was identified by the highest scores obtained with both methods described above. The second group was identified by selecting genes associated with NK cells and cytotoxic T-cells, and demonstrating that all of these genes are significantly downregulated. Materials and Methods Solid tumor patients comprised 10 non-small cell lung carcinoma, 2 sarcoma, 2 pancreatic carcinoma, and 1 each esophageal, ovarian, small cell, adrenal, and mesothelioma, together with 9 normal controls. Approximately 8000 genes had a complete set of expression values across all patients and controls and these values were used for further analysis. Results Analysis of the distance matrix generated using Manhattan or Euclidian distances shows that for most of the samples the most distant member of sample's own group is closer than the closest member of the opposite group (Fig. 1). The only outlier is a lung cancer sample that is positioned exactly halfway between the cancer centroid and control centroid. But this sample is an outlier also relative to the controls: its distance to the control centroid is larger than for any control sample. We confirmed our findings about the distinction between cancer and control groups using two discriminant methods. First, all samples were analyzed with a multiclass shrunken centroids algorithm (1). In this analysis all genes and four samples from each of the two solid tumor groups and control group were used for training and the remaining samples were used as a test set. There is no misclassification of any solid tumor as a control or vice versa. Second, crossvalidation using PDA between control and cancer groups is 100% accurate. To estimate the extent of changes in individual patient samples compared to the control group, we have used the following three metrics. 1) Normalized and shrunken distance to control centroid; 2) Predictive scores for each patient obtained with PDA crossvalidation; 3) Projection of each sample on the Average Control- Average Cancer vector (Fig.2). Metrics 2, and 3 gave similar results. We have used several methods to pick out the most informative genes. Although simple t-test between patients and controls identified a substantial number of differentially expressed genes, we did not use it as a primary metric because of the substantial variance in the patient group. Indeed, we observed that the average distance between cancer samples is much greater than the average distance between normal controls. The distribution of variance in gene expression is the lowest in the two sets of healthy controls, and the largest in lung adenocarcinomas and a group of "mixed cancers". Therefore we used only the variance in the control group to normalize the changes in gene expression and sorted genes by median Z score in the patient group, relative to the control centroid. Alternatively we have used discriminant loadings from PDA classification of Controls vs Patients. To reduce the variance in the most informative genes, we have removed the patient samples that were least advanced in the disease progression as described above. Finally, we have selected the genes known to be expressed exclusively in cytotoxic T-cells and NK cells and found them significantly downregulated.
更多查看译文
关键词
hierarchical clustering,distance matrix,cell growth,gene expression,discriminant analysis,immune response,energy production,control group,cytotoxic t cells
AI 理解论文
溯源树
样例
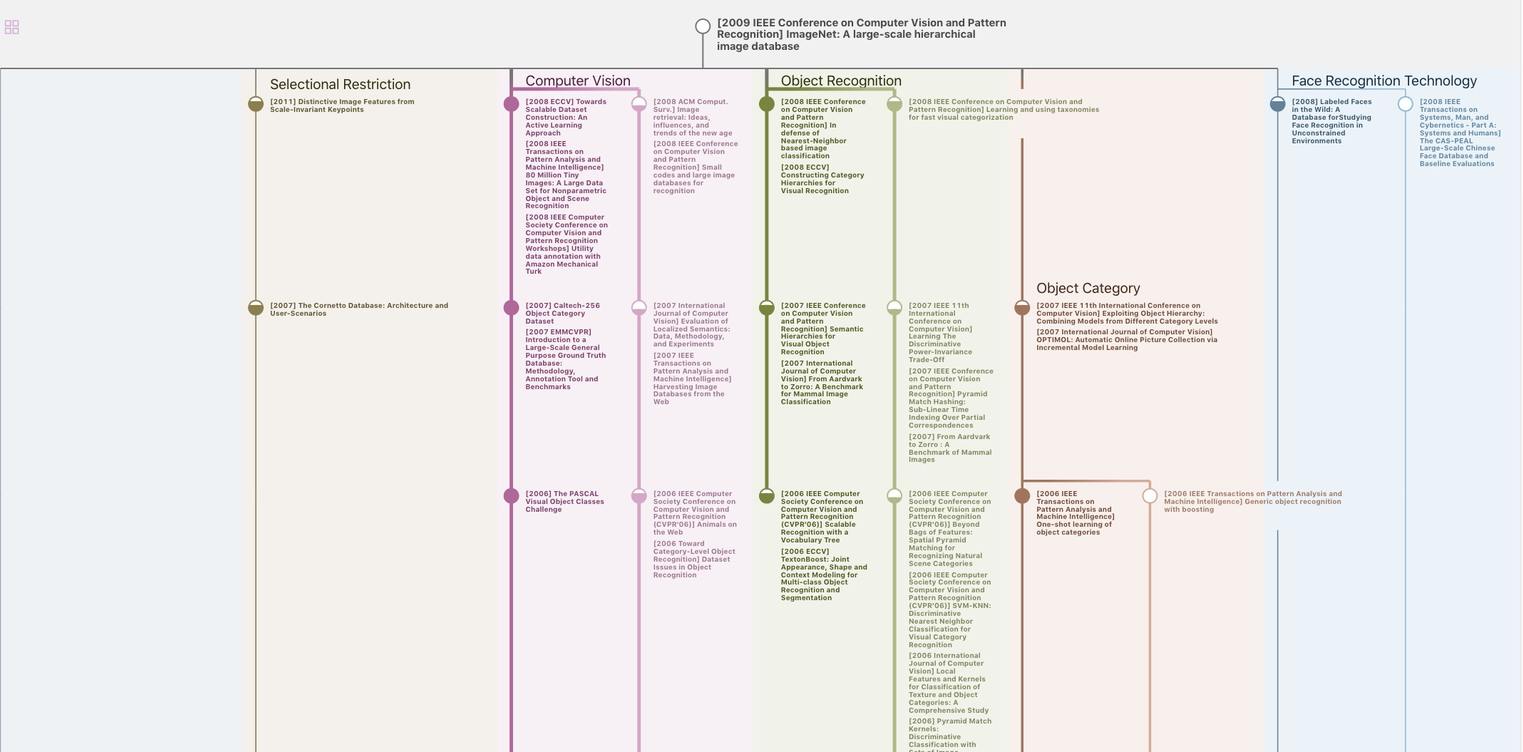
生成溯源树,研究论文发展脉络
Chat Paper
正在生成论文摘要