Locally Boosted Graph Aggregation for Community Detection.
CoRR(2014)
摘要
Learning the right graph representation from noisy, multi-source data has garnered significant interest in recent years. A central tenet of this problem is relational learning. Here the objective is to incorporate the partial information each data source gives us in a way that captures the true underlying relationships. To address this challenge, we present a general, boosting-inspired framework for combining weak evidence of entity associations into a robust similarity metric. Building on previous work, we explore the extent to which different local quality measurements yield graph representations that are suitable for community detection. We present empirical results on a variety of datasets demonstrating the utility of this framework, especially with respect to real datasets where noise and scale present serious challenges. Finally, we prove a convergence theorem in an ideal setting and outline future research into other application domains.
更多查看译文
关键词
boosted graph aggregation,community,detection
AI 理解论文
溯源树
样例
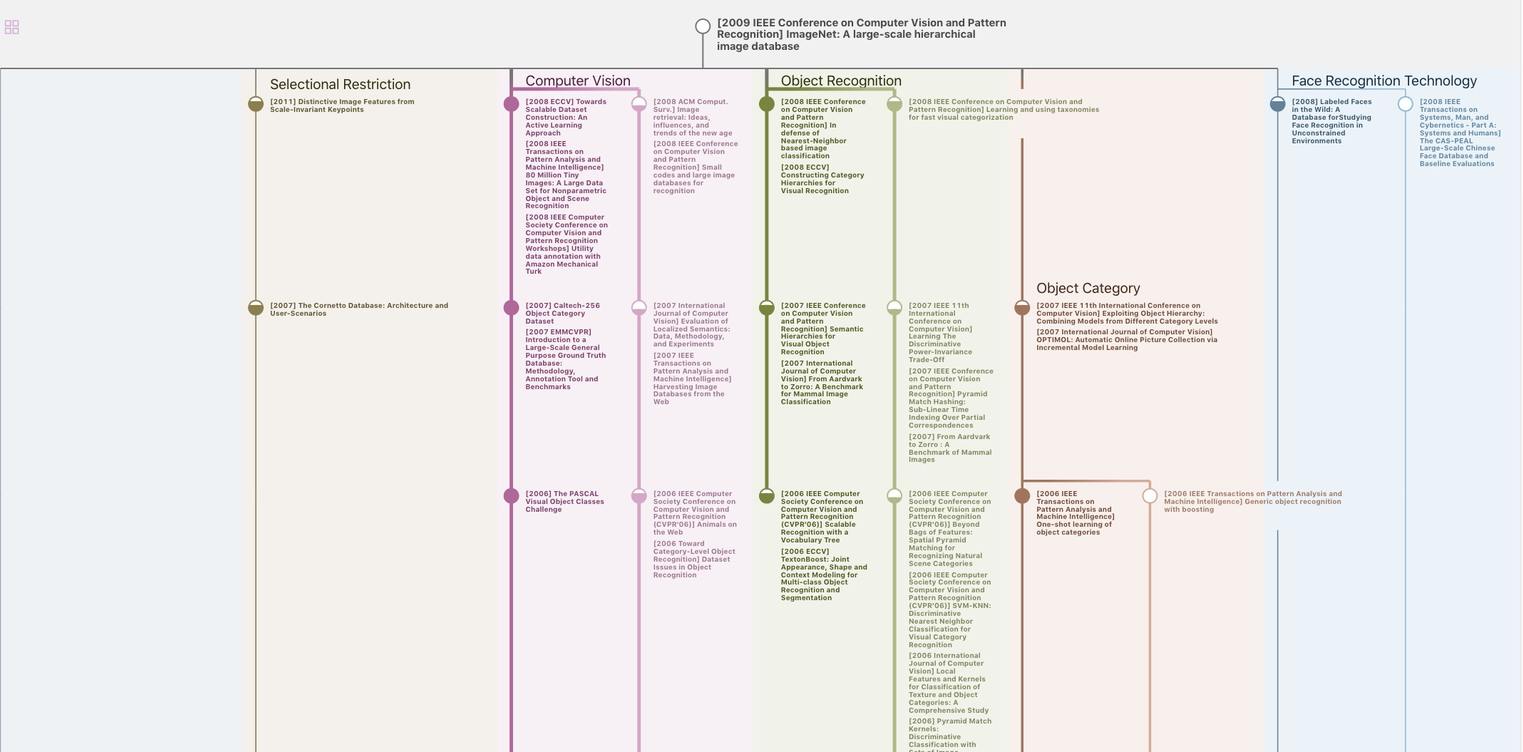
生成溯源树,研究论文发展脉络
Chat Paper
正在生成论文摘要