Sensor-based activity recognition with improved GP-based classifier
IEEE Congress on Evolutionary Computation(2013)
摘要
Compared to conventional activity recognition methods using feature extraction followed by classification, the Genetic Programming (GP) based classification applied to raw sensor data can avoid the time-consuming and knowledge-dependent feature extraction procedure. However, the traditional GP-based classifier using accuracy as fitness function is sensitive to the choice of threshold values. Furthermore, sensor data of the same activity might demonstrate remarkable distinction when the signal is collected in the changing environment, which will lead to inconsistency between training and testing data and consequently degrade the generalization power of the trained classifier. Moreover, the GP-based classifier cannot well distinguish less separable activities in the presence of multiple activities. Our work aims to address these issues by improving the GP-based classifier via: (1) using the area under the receiver operating characteristic curve (AUC) as fitness function, (2) using an online local time series normalization procedure to pre-smooth undesirable features, and (3) using a binary tree based classification framework to force GP to learn key discriminating features that can better distinguish less separable activities. We test the proposed method on a sensor data set collected from a smartphone, consisting of four common human activities, sitting, standing, walking and running. The proposed GP-based classifier achieves the outstanding performance on recognizing each of four activities in terms of both high true positive and low false alarm rates, which much improves over the traditional GP-based classifier and several of its variants.
更多查看译文
关键词
fitness function,sitting activity,false alarm rate,time series normalization procedure,area under the receiver operating characteristic curve,walking activity,knowledge-dependent feature extraction procedure,trees (mathematics),genetic programming,pattern classification,gp-based classifier,smartphone,sensor data,sensor-based activity recognition,feature extraction,genetic algorithms,human activity,threshold value,running activity,time series,binary tree based classification framework,sensor fusion,standing activity,feature classification,activity recognition,testing,accuracy,indexes,time series analysis
AI 理解论文
溯源树
样例
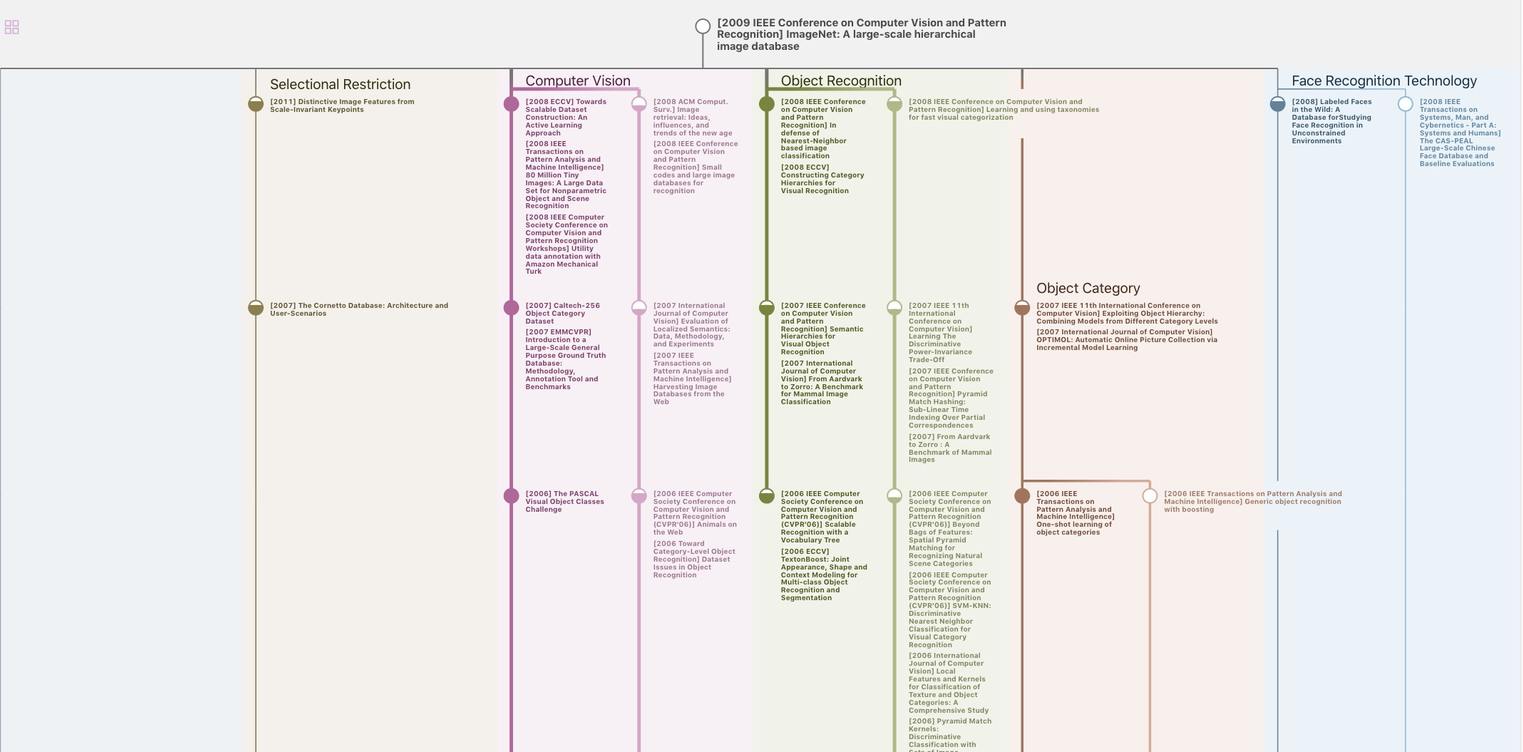
生成溯源树,研究论文发展脉络
Chat Paper
正在生成论文摘要