Joint Training Of Multi-Channel-Condition Dereverberation And Acoustic Modeling Of Microphone Array Speech For Robust Distant Speech Recognition
18TH ANNUAL CONFERENCE OF THE INTERNATIONAL SPEECH COMMUNICATION ASSOCIATION (INTERSPEECH 2017), VOLS 1-6: SITUATED INTERACTION(2017)
摘要
We propose a novel data utilization strategy, called multichannel-condition learning, leveraging upon complementary information captured in microphone array speech to jointly train dereverberation and acoustic deep neural network (DNN) models for robust distant speech recognition. Experimental results, with a single automatic speech recognition (ASR) system, on the REVERB2014 simulated evaluation data show that, on 1-channel testing, the baseline joint training scheme attains a word error rate (WER) of 7.47%, reduced from 8.72% for separate training. The proposed multi-channel-condition learning scheme has been experimented on different channel data combinations and usage showing many interesting implications. Finally, training on all 8-channel data and with DNN-based language model rescoring, a state-of-the-art WER of 4.05% is achieved. We anticipate an even lower WER when combining more top ASR systems.
更多查看译文
关键词
distant speech recognition, reverberant speech recognition, multi-condition, joint training
AI 理解论文
溯源树
样例
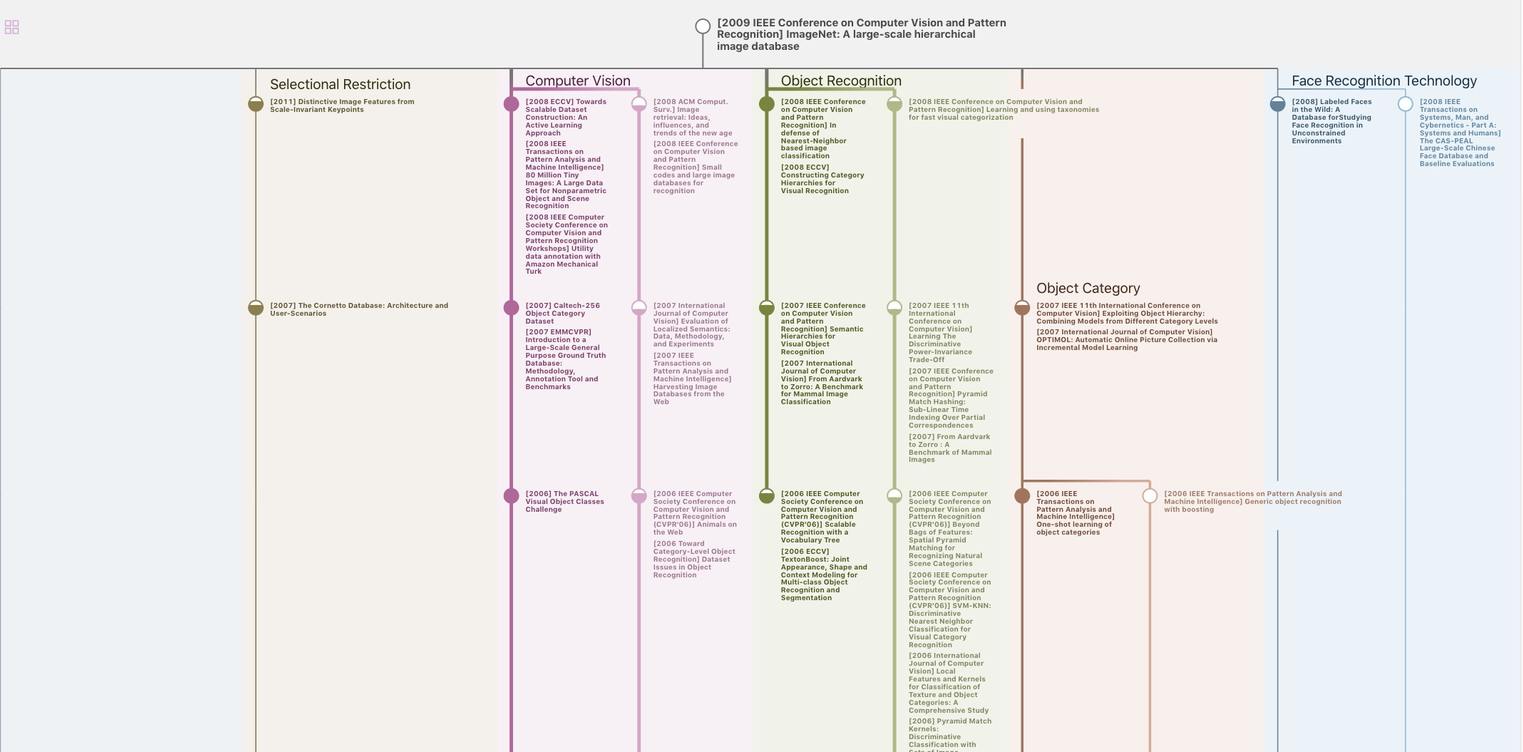
生成溯源树,研究论文发展脉络
Chat Paper
正在生成论文摘要