Back-off action selection in summary space-based POMDP dialogue systems.
ASRU(2009)
摘要
This paper deals with the issue of invalid state-action pairs in the Partially Observable Markov Decision Process (POMDP) framework, with a focus on real-world tasks where the need for approximate solutions exacerbates this problem. In particular, when modelling dialogue as a POMDP, both the state and the action space must be reduced to smaller scale summary spaces in order to make learning tractable. However, since not all actions are valid in all states, the action proposed by the policy in summary space sometimes leads to an invalid action when mapped back to master space. Some form of back-off scheme must then be used to generate an alternative action. This paper demonstrates how the value function derived during reinforcement learning can be used to order back-off actions in an N-best list. Compared to a simple baseline back-off strategy and to a strategy that extends the summary space to minimise the occurrence of invalid actions, the proposed N-best action selection scheme is shown to be significantly more robust.
更多查看译文
关键词
Markov processes,learning (artificial intelligence),speech processing,N-best action selection scheme,POMDP dialogue systems,action space,back-off scheme,partially observable Markov decision process,reinforcement learning,state space
AI 理解论文
溯源树
样例
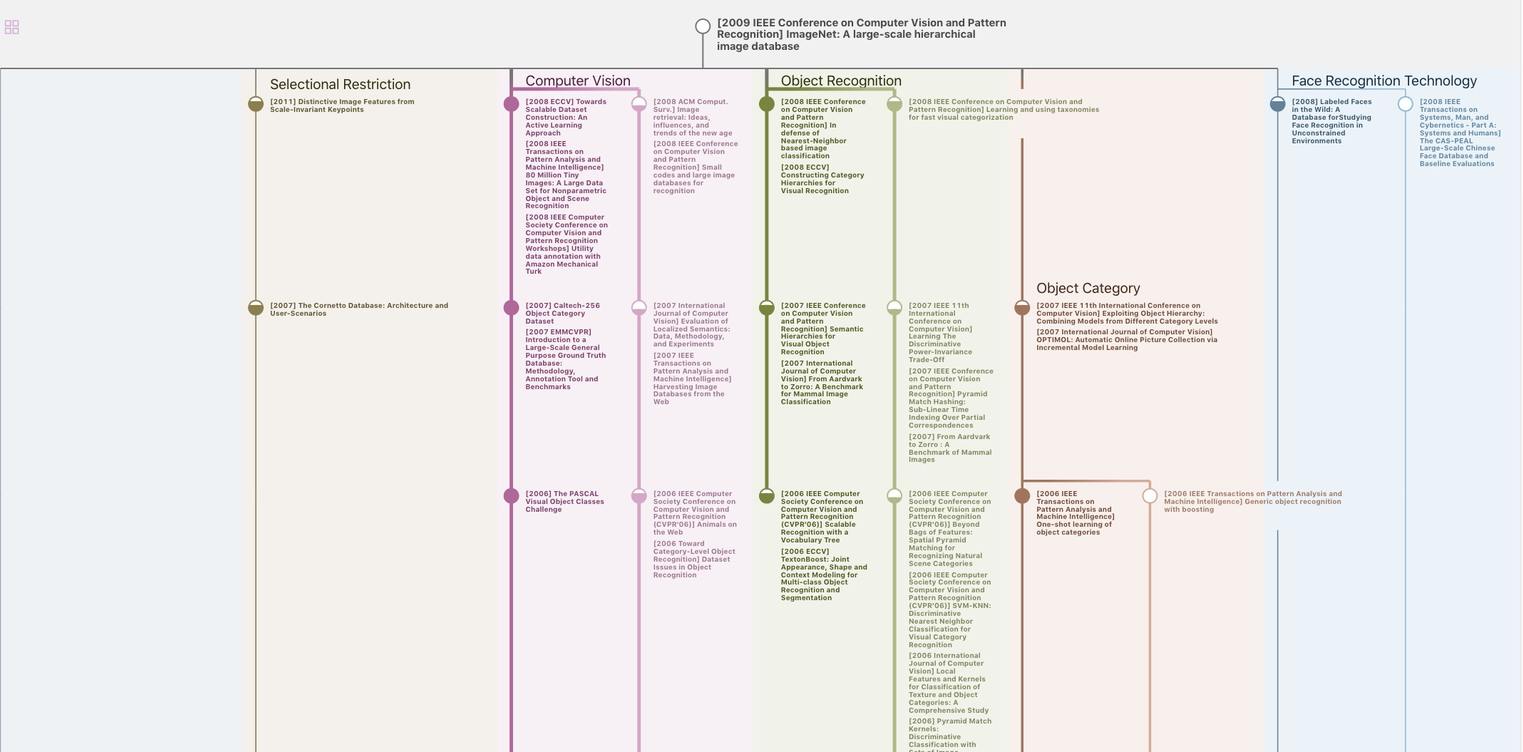
生成溯源树,研究论文发展脉络
Chat Paper
正在生成论文摘要