Dimensionality Reduction for Improved Source Separation in FMRI Data
BIOSIGNALS 2008: PROCEEDINGS OF THE FIRST INTERNATIONAL CONFERENCE ON BIO-INSPIRED SYSTEMS AND SIGNAL PROCESSING, VOL II(2008)
摘要
Functional magnetic resonance imaging (fMRI) captures brain activity by measuring the hemodynamic response. It is often used to associate specific brain activity with specific behavior or tasks. The analysis of fMRI scans seeks to recover this association by differentiating between task and non-task related activation and by spatially isolating brain activity. In this paper, we frame the association problem as a convolution of activation patterns. We project MU scans into a low dimensional space using manifold learning techniques. In this subspace, we transform the time course of each projected fMRI volume into the frequency domain. We use independent component analysis to discover task related activations. The combination of these methods discovers sources that show stronger correlation with the activation reference function than previous methods.
更多查看译文
关键词
dimensionality reduction,ICA,fMRI
AI 理解论文
溯源树
样例
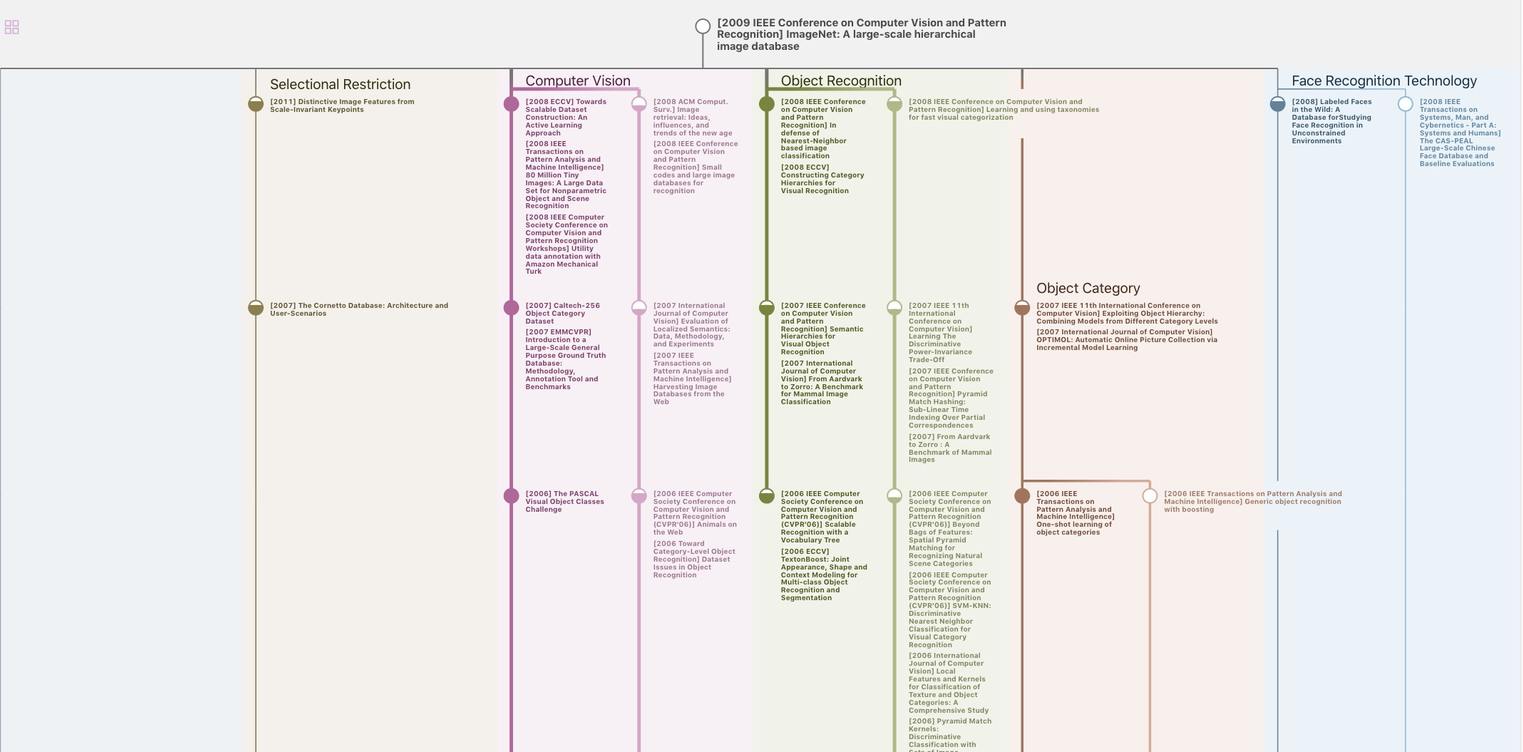
生成溯源树,研究论文发展脉络
Chat Paper
正在生成论文摘要