Far Casting Cross-Validation
JOURNAL OF COMPUTATIONAL AND GRAPHICAL STATISTICS(2012)
摘要
Cross-validation has long been used for choosing tuning parameters and other model selection tasks. It generally performs well provided the data are independent, or nearly so. Improvements have been Suggested which address ordinary cross-validation's (OCV) shortcomings in correlated data. Whereas these techniques have merit, they can still lead to poor model selection in correlated data or are not readily generalizable to high-dimensional data. The proposed solution, far casting cross-validation (FCCV), addresses these problems. FCCV withholds correlated neighbors in every aspect of the cross-validation procedure. The result is a technique that stresses a fitted model's ability to extrapolate rather than interpolate. This generally leads to better model selection in correlated datasets. Whereas FCCV is less than optimal in the independence case, our improvement of OCV applies more generally to higher dimensional error processes and to both parametric and nonparametric model selection problems. To facilitate introduction, we consider only one application, namely estimating global bandwidths for curve estimation with local linear regression. We provide theoretical motivation and report some comparative results from a simulation experiment and on a time series of annual global temperature deviations. For such data, FCCV generally has lower average squared error when disturbances are correlated. Supplementary materials are available online.
更多查看译文
关键词
Dependent data,Optimistic error rates,Prediction,Temporal correlation,Tuning parameter
AI 理解论文
溯源树
样例
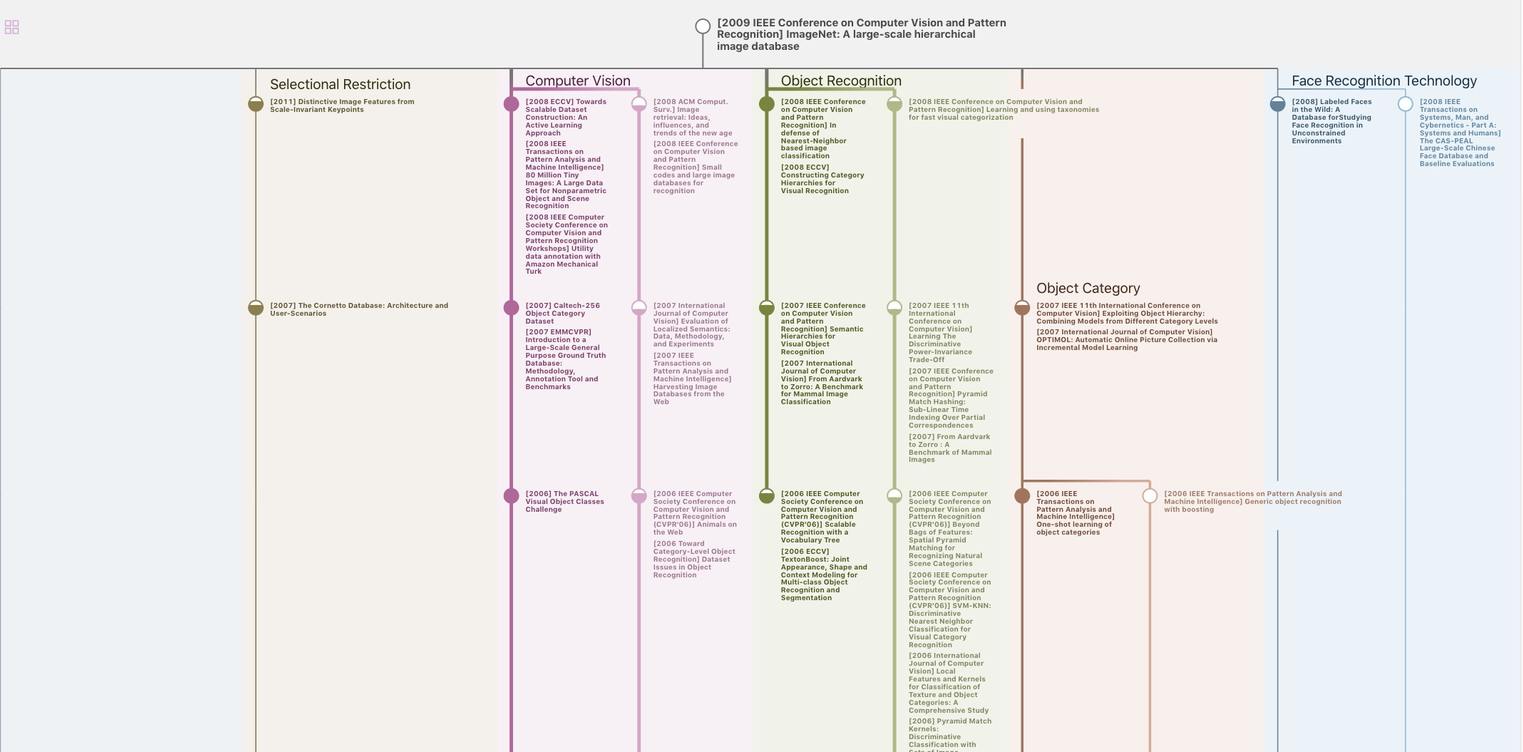
生成溯源树,研究论文发展脉络
Chat Paper
正在生成论文摘要