Approximation Algorithms Going Online
msra(2007)
摘要
In an online linear optimization problem, on each period t, an online algorithm chooses st ∈ S from a fixed (possibly infinite) set S of feasible decisions. Nature (who may be adversarial) chooses a weight vector wt ∈ Rn, and the algorithm incurs cost c(st, wt), where c is a fixed cost function that is linear in the weight vector. In the full-information setting, the vector wt is then revealed to the algorithm, and in the bandit setting, only the cost experienced, c(st, wt), is revealed. The goal of the online algorithm is to perform nearly as well as the best fixed s ∈ S in hindsight. Many repeated decision-making problems with weights fit natural ly into this framework, such as online shortest-path, onlin e TSP, online clustering, and online weighted set cover. Previously, it was shown how to convert any efficient exact offline optimization algorithm for such a problem into an efficient online bandit algorithm in both the full-informat ion and the bandit settings, with average cost nearly as good as that of the best fixed s ∈ S in hindsight. However, in the case where the offline algorith m is an approximation algorithm with ratio α > 1, the previous approach only worked for special types of approximation algorithms. We show how to convert any efficient offline α-approximation algorithm for a linear optimization problem into an efficient algorithm for the corresponding online problem, w ith average cost not much larger than α times that of the best s ∈ S, in both the full-information and the bandit settings. Our m ain innovation is in the full-information setting: we combine Zinkevich's algorithm for convex optimization w ith a geometric transformation that can be applied to any approximation algorithm. In the bandit setting, standard t echniques apply, except that a "Barycentric Spanner" for the problem is also (provably) necessary as input. Our algorithm can also be viewed as a method for playing a large repeated games, where one can only compute approximatebest-responses, rather than best-responses.
更多查看译文
AI 理解论文
溯源树
样例
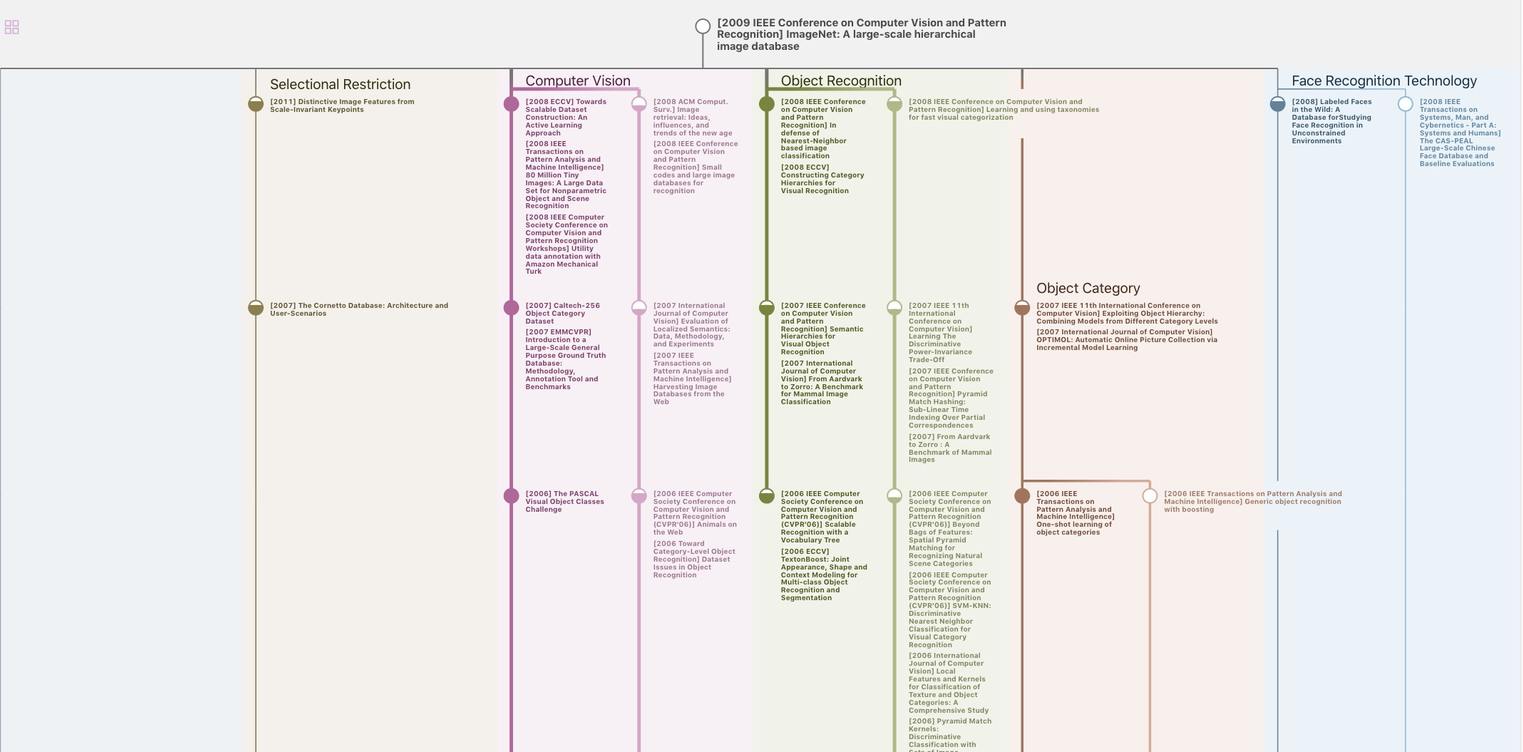
生成溯源树,研究论文发展脉络
Chat Paper
正在生成论文摘要