Non-Local Contrastive Objectives
ICML(2010)
摘要
Pseudo-likelihood and contrastive divergence are two well-known examples of contrastive methods. These algorithms trade o the probability of the correct label with the prob- abilities of other "nearby" instantiations. In this paper we explore more general types of contrastive objectives, which trade o the probability of the correct label against an arbitrary set of other instantiations. We prove that a large class of contrastive ob- jectives are consistent with maximum likeli- hood, even for finite amounts of data. This result generalizes asymptotic consistency for pseudo-likelihood. The proof gives signifi- cant insight into contrastive objectives, sug- gesting that they enforce (soft) probability- ratio constraints between pairs of instanti- ations. Based on this insight, we propose Contrastive Constraint Generation (CCG), an iterative constraint-generation style algo- rithm that allows us to learn a log-linear model using only MAP inference. We evalu- ate CCG on a scene classification task, show- ing that it significantly outperforms pseudo- likelihood, contrastive divergence, and a well- known margin-based method.
更多查看译文
关键词
log linear model
AI 理解论文
溯源树
样例
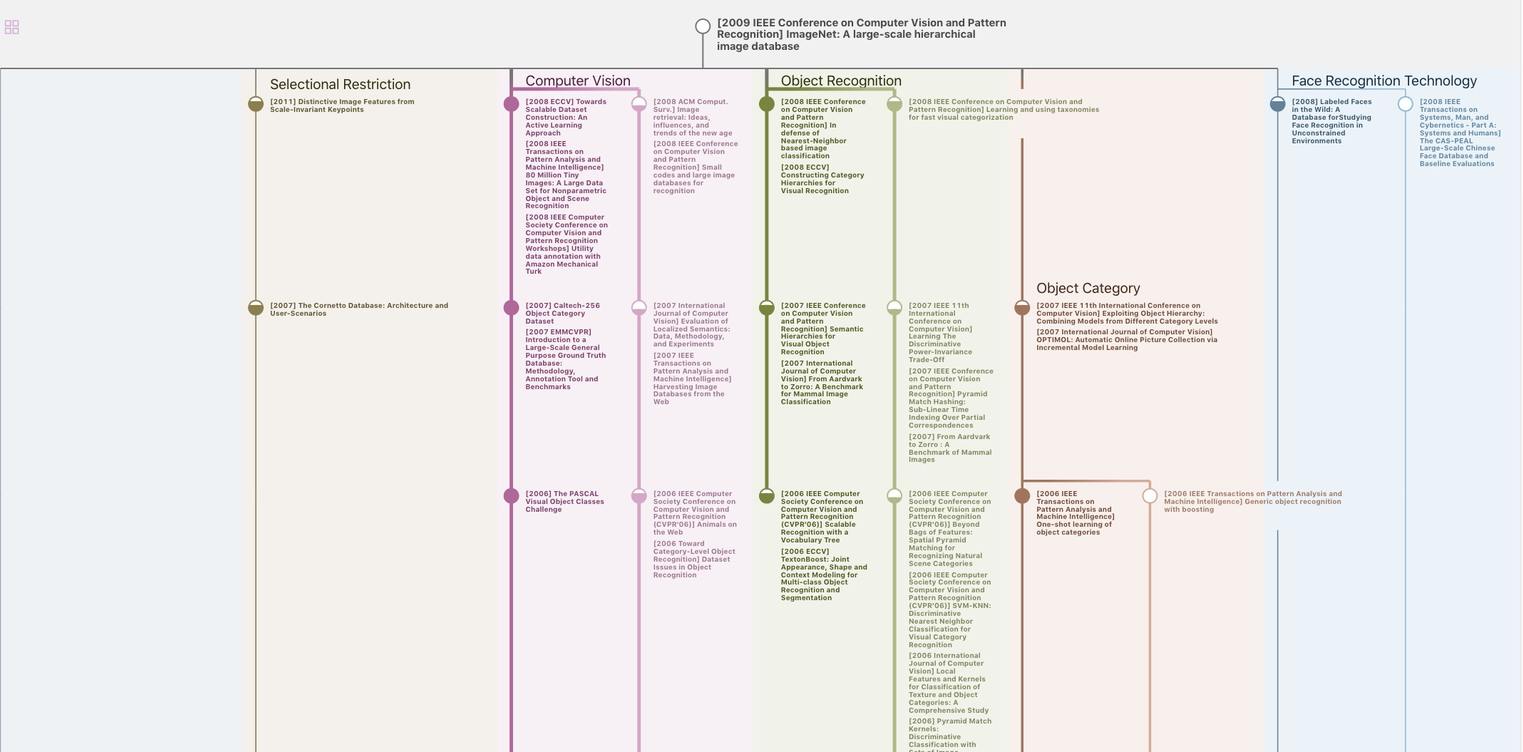
生成溯源树,研究论文发展脉络
Chat Paper
正在生成论文摘要