Learning hierarchical similarity metrics
CVPR(2012)
摘要
Categories in multi-class data are often part of an underlying semantic taxonomy. Recent work in object classification has found interesting ways to use this taxonomy structure to develop better recognition algorithms. Here we propose a novel framework to learn similarity metrics using the class taxonomy. We show that a nearest neighbor classifier using the learned metrics gets improved performance over the best discriminative methods. Moreover, by incorporating the taxonomy, our learned metrics can also help in some taxonomy specific applications. We show that the metrics can help determine the correct placement of a new category that was not part of the original taxonomy, and can provide effective classification amongst categories local to specific subtrees of the taxonomy.
更多查看译文
关键词
taxonomy structure,similarity metrics,original taxonomy,object classification,class taxonomy,underlying semantic taxonomy,better recognition algorithm,effective classification,hierarchical similarity metrics,specific subtrees,taxonomy specific application
AI 理解论文
溯源树
样例
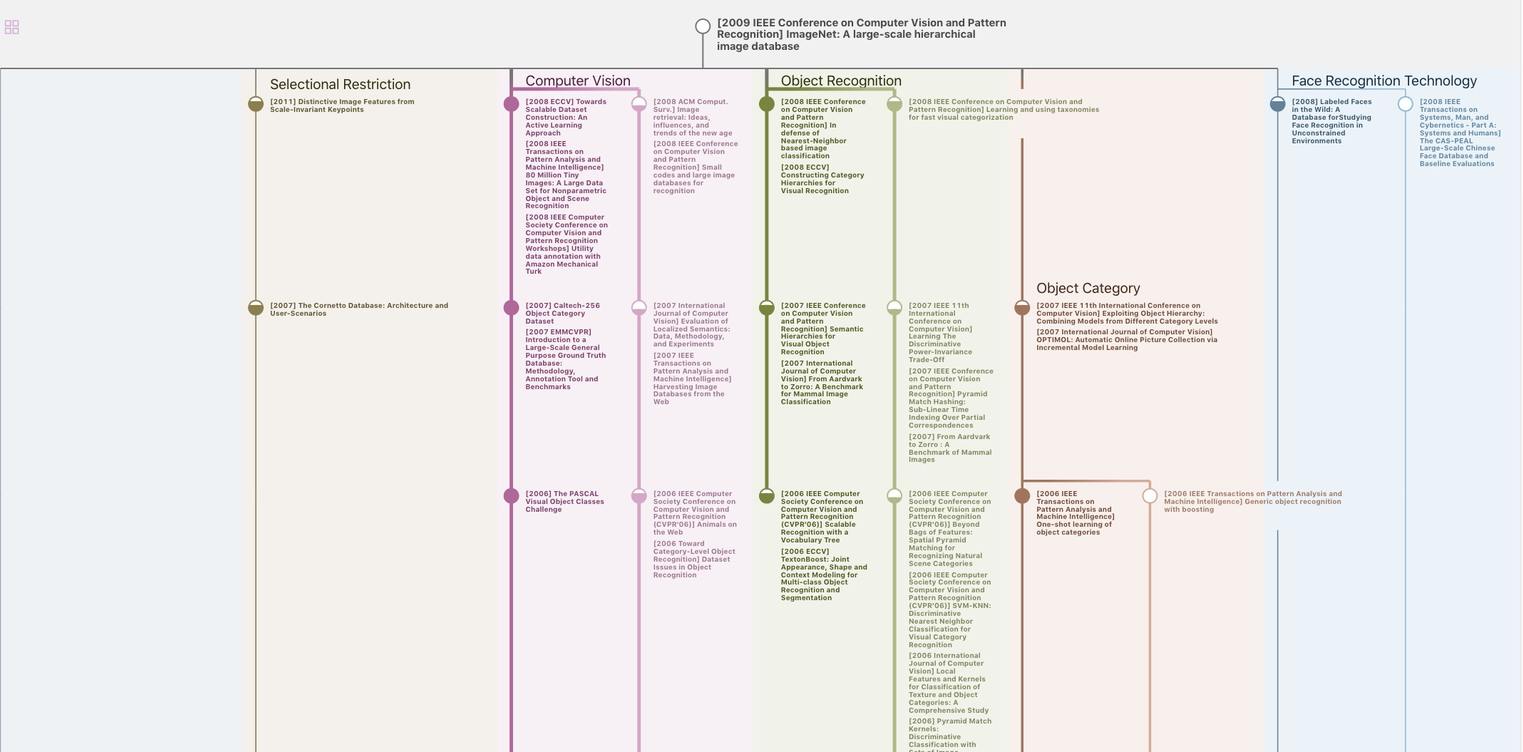
生成溯源树,研究论文发展脉络
Chat Paper
正在生成论文摘要