Implementing componentwise Hastings algorithms
Computational Statistics & Data Analysis(2005)
摘要
Markov chain Monte Carlo (MCMC) routines have revolutionized the application of Monte Carlo methods in statistical application and statistical computing methodology. The Hastings sampler, encompassing both the Gibbs and Metropolis samplers as special cases, is the most commonly applied MCMC algorithm. The performance of the Hastings sampler relies heavily on the choice of sweep strategy, that is, the method by which the components or blocks of the random variable X of interest are visited and updated, and the choice of proposal distribution, that is the distribution from which candidate variates are drawn for the accept–reject rule in each iteration of the algorithm. We focus on the random sweep strategy, where the components of X are updated in a random order, and random proposal distributions, where the proposal distribution is characterized by a randomly generated parameter. We develop an adaptive Hastings sampler which learns from and adapts to random variates generated during the algorithm towards choosing the optimal random sweep strategy and proposal distribution for the problem at hand. As part of the development, we prove convergence of the random variates to the distribution of interest and discuss practical implementations of the methods. We illustrate the results presented by applying the adaptive componentwise Hastings samplers developed to sample multivariate Gaussian target distributions and Bayesian frailty models.
更多查看译文
关键词
Markov chain Monte Carlo,Metropolis algorithm,Gibbs sampler,Adaptive sweep strategies,Random proposal distributions
AI 理解论文
溯源树
样例
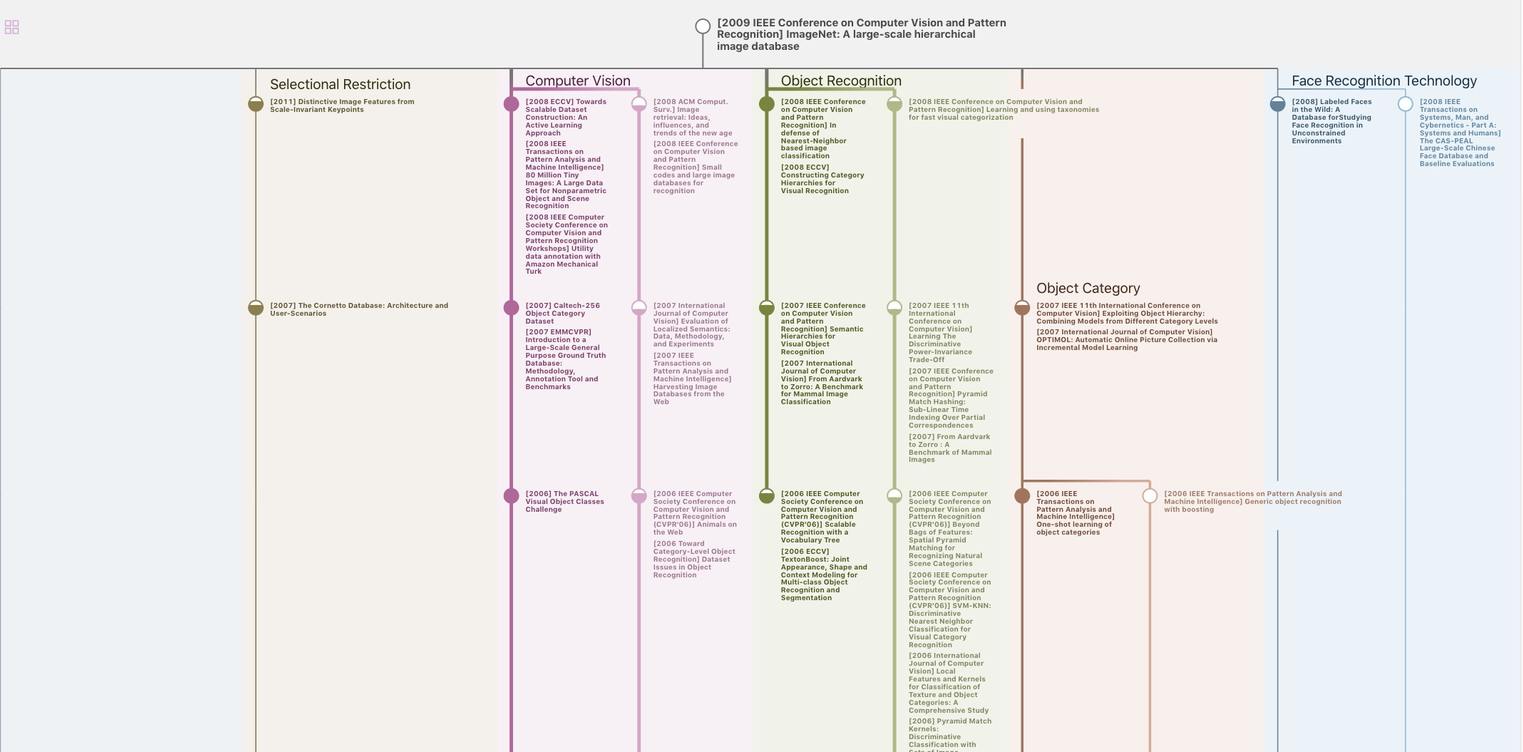
生成溯源树,研究论文发展脉络
Chat Paper
正在生成论文摘要