Gaussian Process Dynamical Models
NIPS(2005)
摘要
This paper introduces Gaussian Process Dynamical Models (GPDM) for nonlinear time series analysis. A GPDM comprises a low-dimensional latent space with associated dynamics, and a map from the latent space to an observation space. We marginalize out the model parameters in closed-form, using Gaussian Process (GP) priors for both the dynamics and the observation mappings. This results in a nonparametric model for dynamical systems that accounts for uncertainty in the model. We demonstrate the approach on human motion capture data in which each pose is 62-dimensional. Despite the use of small data sets, the GPDM learns an effective representation of the nonlinear dynamics in these spaces. Webpage: http://www.dgp.toronto.edu/
更多查看译文
关键词
dynamic system,gaussian process,parametric model,nonlinear dynamics
AI 理解论文
溯源树
样例
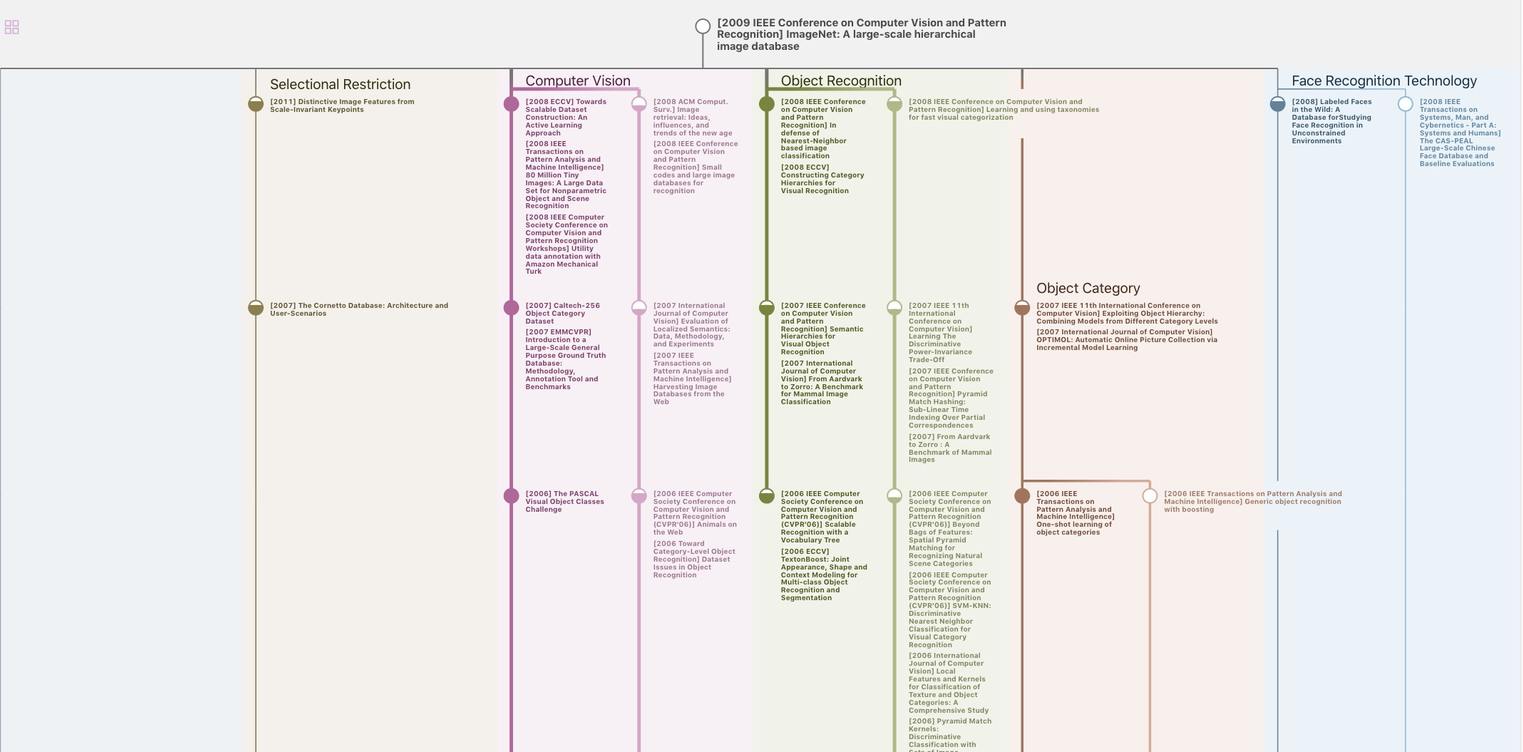
生成溯源树,研究论文发展脉络
Chat Paper
正在生成论文摘要