Probabilistic Sparse Matrix Factorization
msra(2004)
摘要
Many kinds of data can be viewed as consisting of a set of vectors, each of which is a noisy combination of a small number of noisy prototype vec- tors. Physically, these prototype vectors may correspond to different hidden variables that play a role in determining the measured data. For example, a gene's expression is inuenced by the presence of transcription factor pro- teins, and two genes may be activated by overlapping sets of transcription factors. Consequently, the activity of each gene can be explained by the ac- tivities of a small number of transcription factors. This task can be viewed as the problem of factorizing a data matrix, while taking into account hard constraints reecting structural knowledge of the problem and probabilistic relationships between variables that are induced by known uncertainties in the problem. We present soft-decision probabilistic sparse matrix factoriza- tion (PSMF) to better account for uncertainties due to varying levels of noise in the data, varying levels of noise in the prototypes used to explain the data, and uncertainty as to which hidden prototypes are selected to explain each expression vector.
更多查看译文
关键词
hidden variables,sparse matrix,transcription factor
AI 理解论文
溯源树
样例
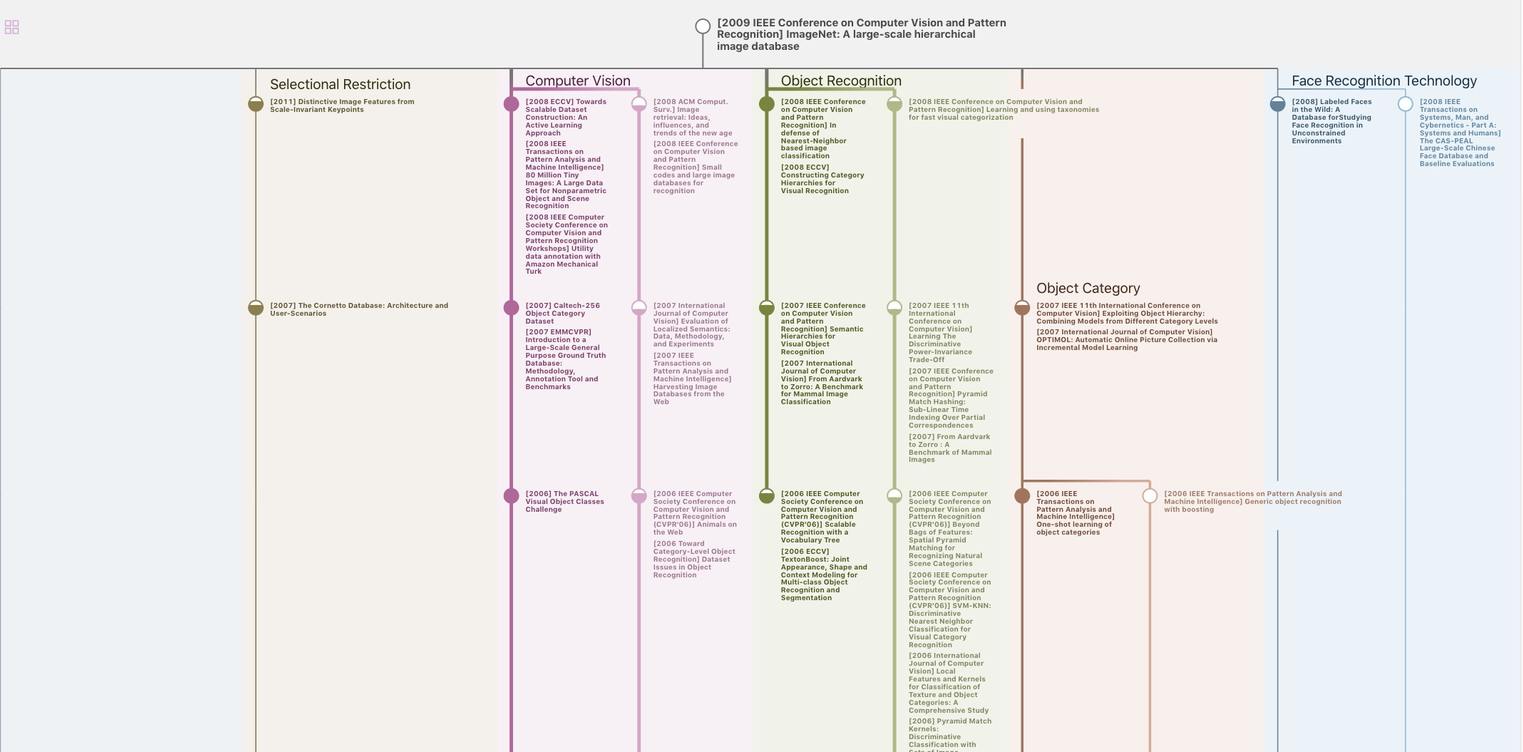
生成溯源树,研究论文发展脉络
Chat Paper
正在生成论文摘要