Learning Polyhedral Classifiers Using Logistic Function
JMLR Workshop and Conference Proceedings(2010)
摘要
In this paper we propose a new algorithm for learning polyhedral classifiers. In contrast to existing methods for learning polyhedral classifier which solve a constrained optimization problem, our method solves an unconstrained optimization problem. Our method is based on a logistic function based model for the posterior probability function. We propose an alternating optimization algorithm, namely, SPLA1 (Single Polyhedral Learning Algorithm1) which maximizes the log-likelihood of the training data to learn the parameters. We also extend our method to make it independent of any user specified parameter (e.g., number of hyperplanes required to form a polyhedral set) in SPLA2. We show the effectiveness of our approach with experiments on various synthetic and real world datasets and compare our approach with a standard decision tree method (OC1) and a constrained optimization based method for learning polyhedral sets (Astorino and Gaudioso, 2002).
更多查看译文
关键词
Polyhedral Learning,Logistic Regression,Alternating Optimization
AI 理解论文
溯源树
样例
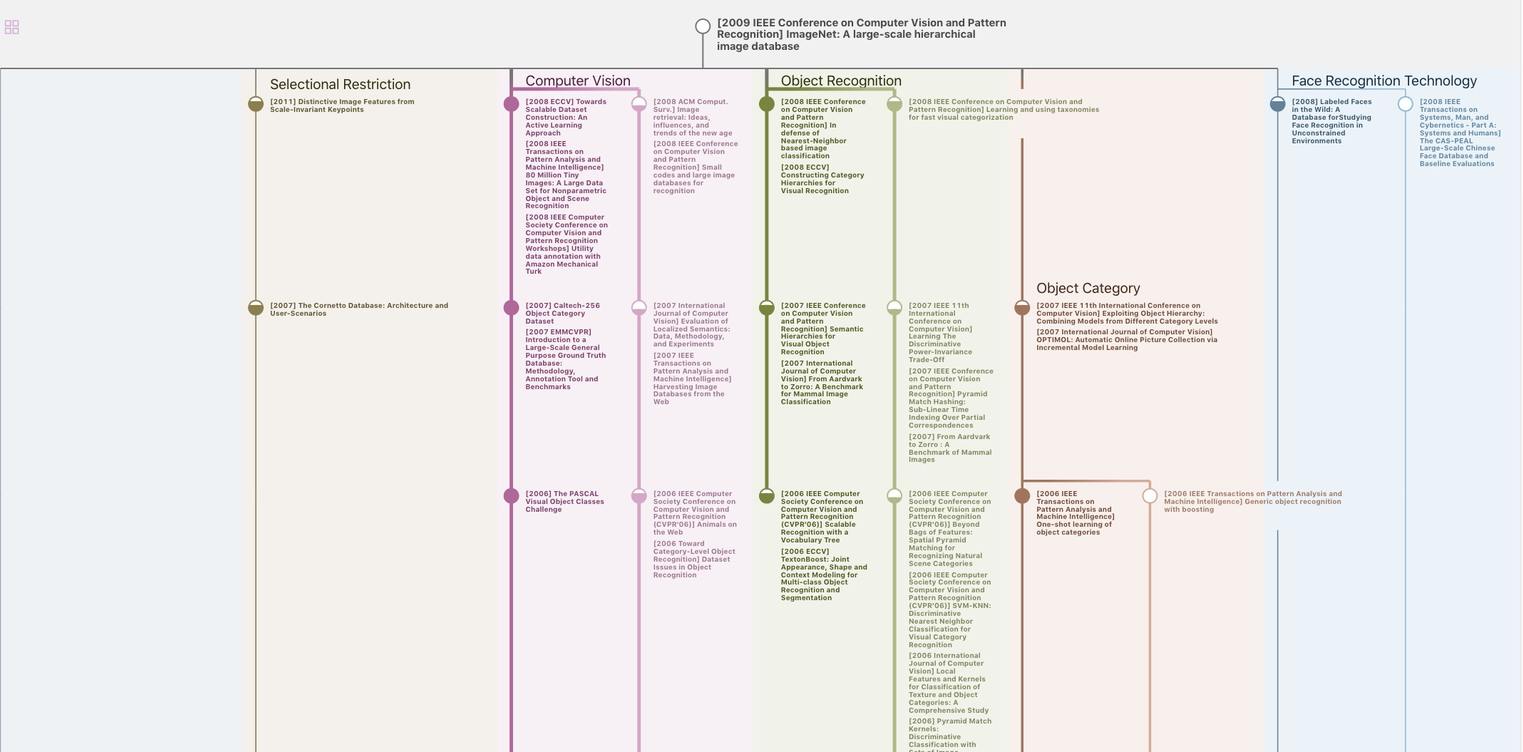
生成溯源树,研究论文发展脉络
Chat Paper
正在生成论文摘要