Robust Boltzmann Machines for recognition and denoising
CVPR(2012)
摘要
While Boltzmann Machines have been successful at unsupervised learning and density modeling of images and speech data, they can be very sensitive to noise in the data. In this paper, we introduce a novel model, the Robust Boltzmann Machine (RoBM), which allows Boltzmann Machines to be robust to corruptions. In the domain of visual recognition, the RoBM is able to accurately deal with occlusions and noise by using multiplicative gating to induce a scale mixture of Gaussians over pixels. Image denoising and in-painting correspond to posterior inference in the RoBM. Our model is trained in an unsupervised fashion with unlabeled noisy data and can learn the spatial structure of the occluders. Compared to standard algorithms, the RoBM is significantly better at recognition and denoising on several face databases.
更多查看译文
关键词
boltzmann machines,unsupervised fashion,visual recognition,novel model,speech data,unsupervised learning,robust boltzmann machines,unlabeled noisy data,robust boltzmann machine,image denoising,density modeling,face,learning artificial intelligence,image recognition,databases,noise measurement,noise,supervised learning,data models,image reconstruction,robustness
AI 理解论文
溯源树
样例
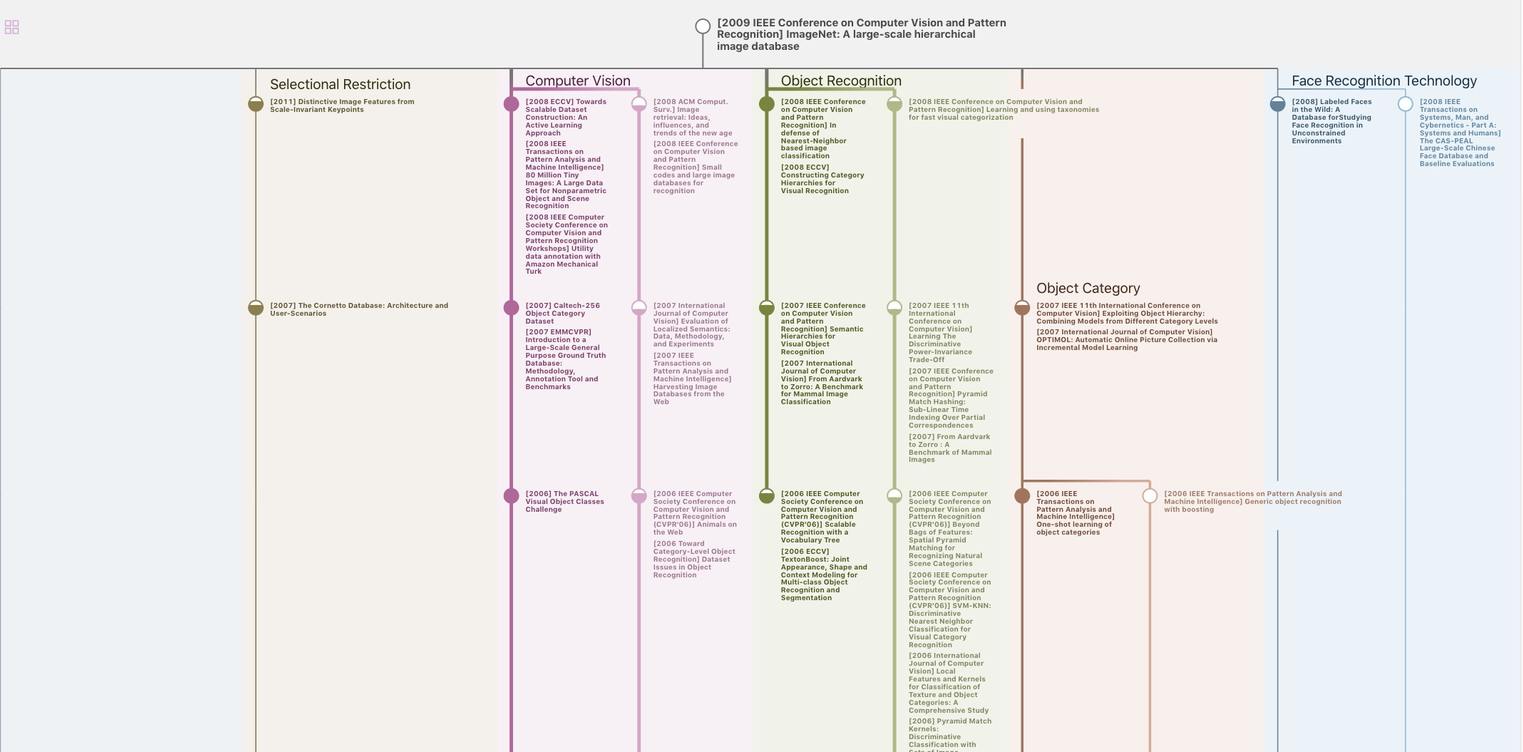
生成溯源树,研究论文发展脉络
Chat Paper
正在生成论文摘要