Applications of distributional vector space models to modeling of psycholinguistic phenomena
Applications of distributional vector space models to modeling of psycholinguistic phenomena(2010)
Abstract
Distributional vector-space models (DVSMs) are unsupervised statistical models of meaning of words and text documents. They derive their representation by analyzing word occurrence patterns in large collections of natural language text. Distributional models provide an efficient and robust way to represent semantics of words and text, which is useful in various Natural Language Processing applications, such as information retrieval, word sense disambiguation and intelligent tutoring systems. On the theoretical side, these methods offer insights and compelling hypotheses of important processes in human memory and language acquisition. On the practical side their unsupervised nature and straightforward numerical representation enables them to be useful as part of sophisticated language technologies for a wide range of applications. This work will focus on applying distributional vector space models to three new areas. These areas are interesting both theoretically, by providing insights into psycholinguistic properties of language, as well as for practical applications, such as information retrieval, language tutoring and cognitive accessibility. Previous approaches in all of these areas relied on simple statistical heuristics, such as word frequency. This work is the first to introduce semantic analysis to all of these areas. The first area is word specificity: the notion that some words are more precise and carry more semantic content than other words that are more vague and general. I show, using a broad range of quantitative and qualitative tests that a DVSM allows for a simple yet effective way to computationally estimate word specificity based on the rate of meaning acquisition. Furthermore, I demonstrate that the specificity metric derived in this way can be used in some DVSMs to improve the accuracy of document representation. The second area is the metric for determining meaning maturity of words and texts. By modeling language acquisition using DVSMs, we can provide an accurate picture of how well we would expect certain words to be known or text passages to be understood by typical language learners at particular levels of language exposure. The word maturity metric is very useful in educational and accessibility applications. Lastly, I propose the notion of word importance, and develop a DVSM-based algorithm to measure it. Word importance refers to the importance of individual words for constructing the meaning of particular text passages or shaping the development of meaning of other words. Word importance is highly useful in educational applications for informing which words to prioritize in targeted vocabulary instruction. Finally, I briefly describe ongoing work in personalized vocabulary instruction that uses all three of these aspects in a sophisticated educational technology.
MoreTranslated text
Key words
distributional vector space model,word sense disambiguation,information retrieval,word occurrence pattern,word frequency,language acquisition,psycholinguistic phenomenon,word maturity metric,word importance,certain word,estimate word specificity,individual word
AI Read Science
Must-Reading Tree
Example
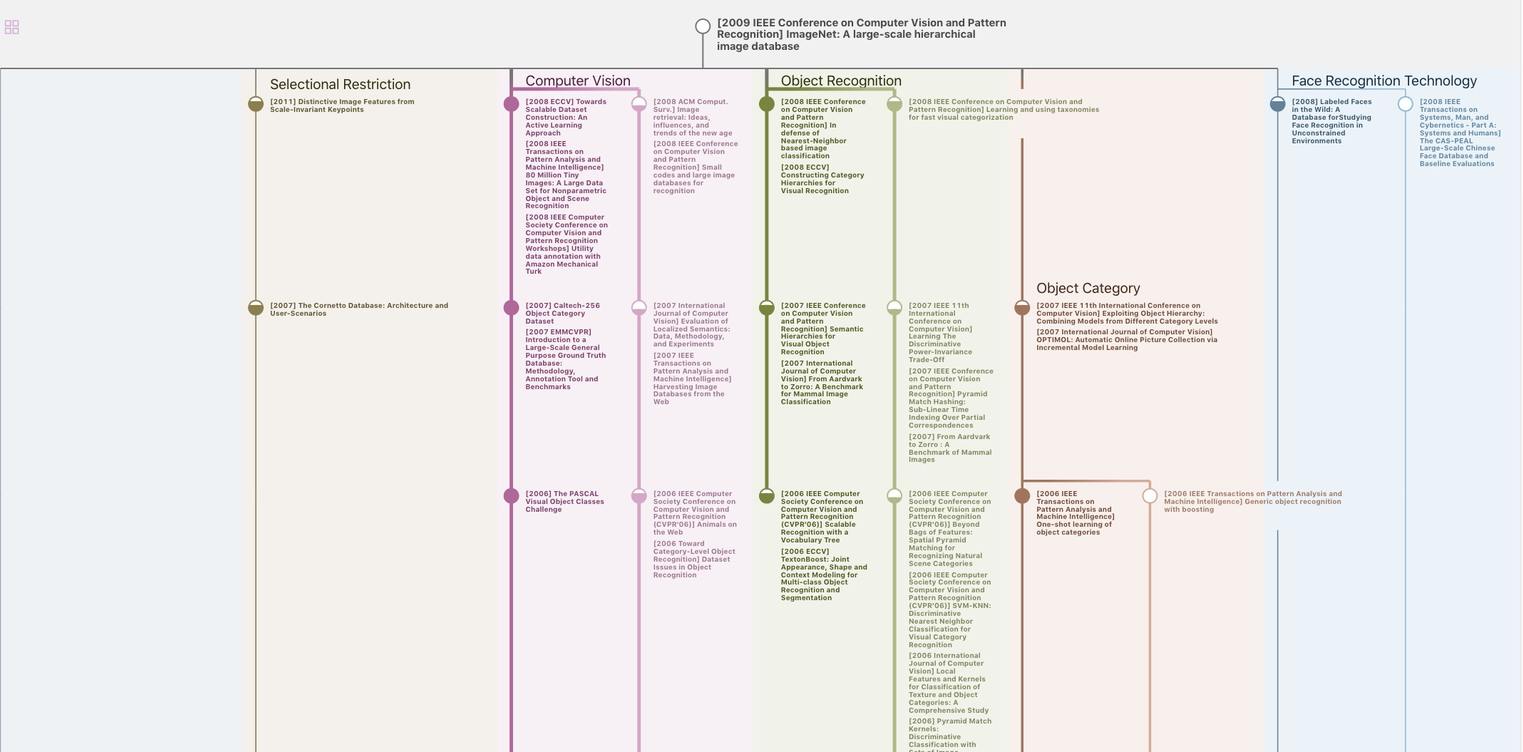
Generate MRT to find the research sequence of this paper
Chat Paper
Summary is being generated by the instructions you defined