Multibody Factorization with Uncertainty and Missing Data Using the EM Algorithm
CVPR(2004)
摘要
Multibody factorization algorithms (2, 1, 16) give an el- egant and simple solution to the problem of structure from motion even for scenes containing multiple independent mo- tions. Despite this elegance, it is still quite difficult to ap- ply these algorithms to arbitrary scenes. First, their per- formance deteriorates rapidly with increasing noise. Sec- ond, they cannot be applied unless all the points can be tracked in all the frames (as will rarely happen in real scenes). Third, they cannot incorporate prior knowledge on the structure or the motion of the objects. In this paper we present a multibody factorization algo- rithm that can handle arbitrary noise covariance for each feature as well as missing data. We show how to formu- late the problem as one of factor analysis and derive an expectation-maximization based maximum-likelihood algo- rithm. One of the advantages of our formulation is that we can easily incorporate prior knowledge, including the as- sumption of temporal coherence. We show that this assump- tion greatly enhances the robustness of our algorithm and present results on challenging sequences.
更多查看译文
关键词
covariance analysis,covariance matrices,image segmentation,image sequences,matrix decomposition,maximum likelihood estimation,optimisation,arbitrary noise covariance,arbitrary scenes,directional uncertainty,expectation-maximization algorithm,image sequence,maximum likelihood algorithm,missing data,motion segmentation,multibody factorization algorithm,multiple independent motions,prior knowledge,robustness,temporal coherence
AI 理解论文
溯源树
样例
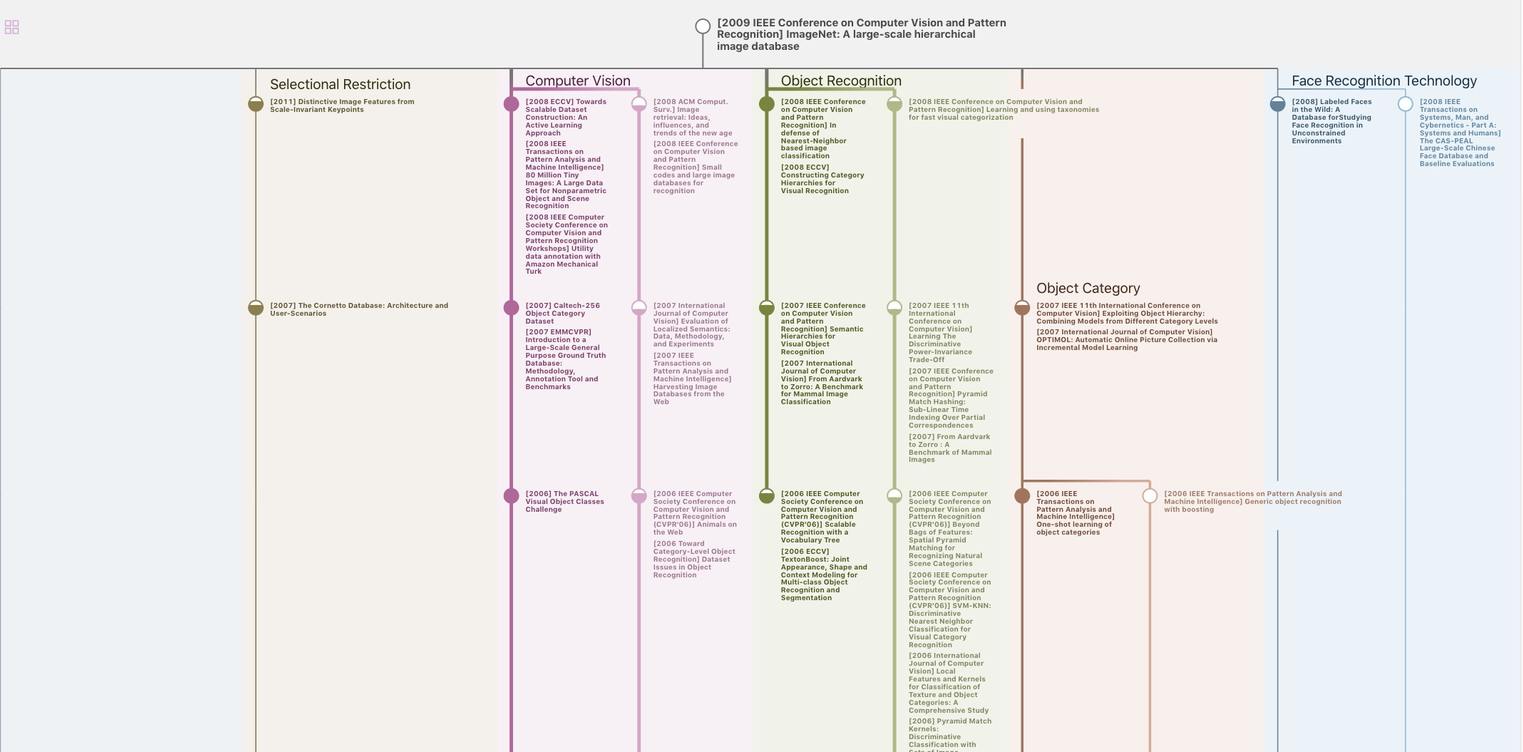
生成溯源树,研究论文发展脉络
Chat Paper
正在生成论文摘要