Predicting Best Responder in Community Question Answering Using Topic Model Method
Web Intelligence(2012)
摘要
Community question answering (CQA) services provide an open platform for people to share their knowledge and have attracted great attention for its rapidly increasing popularity. As the more knowledge people provided are shared in CQA, how to use the historical knowledge for solving new questions has become a crucial problem. In this paper, we investigate the problem as predicting best responders for new questions and tackle the problem from two perspectives, one is from the asker of the new question, and the other is from the question itself. We propose two supervised topic models, Asker-Responder Topic Model (ARTM) and Question-Responder Topic Model (QRTM) for both two perspectives by tracking people's answering history as background knowledge. Our experiments show that the two supervised topic models can effectively predict best responders for new questions in CQA without any additional works and have significant improvement over the baseline method.
更多查看译文
关键词
people answering history,knowledge people,supervised topic model,asker-responder topic model,community question answering services,community question answering,knowledge management,crucial problem,knowledge sharing,topic model method,historical knowledge,question-responder topic model,best responder prediction,new question,qrtm,social networking (online),best responder,cqa,question answering (information retrieval),artm
AI 理解论文
溯源树
样例
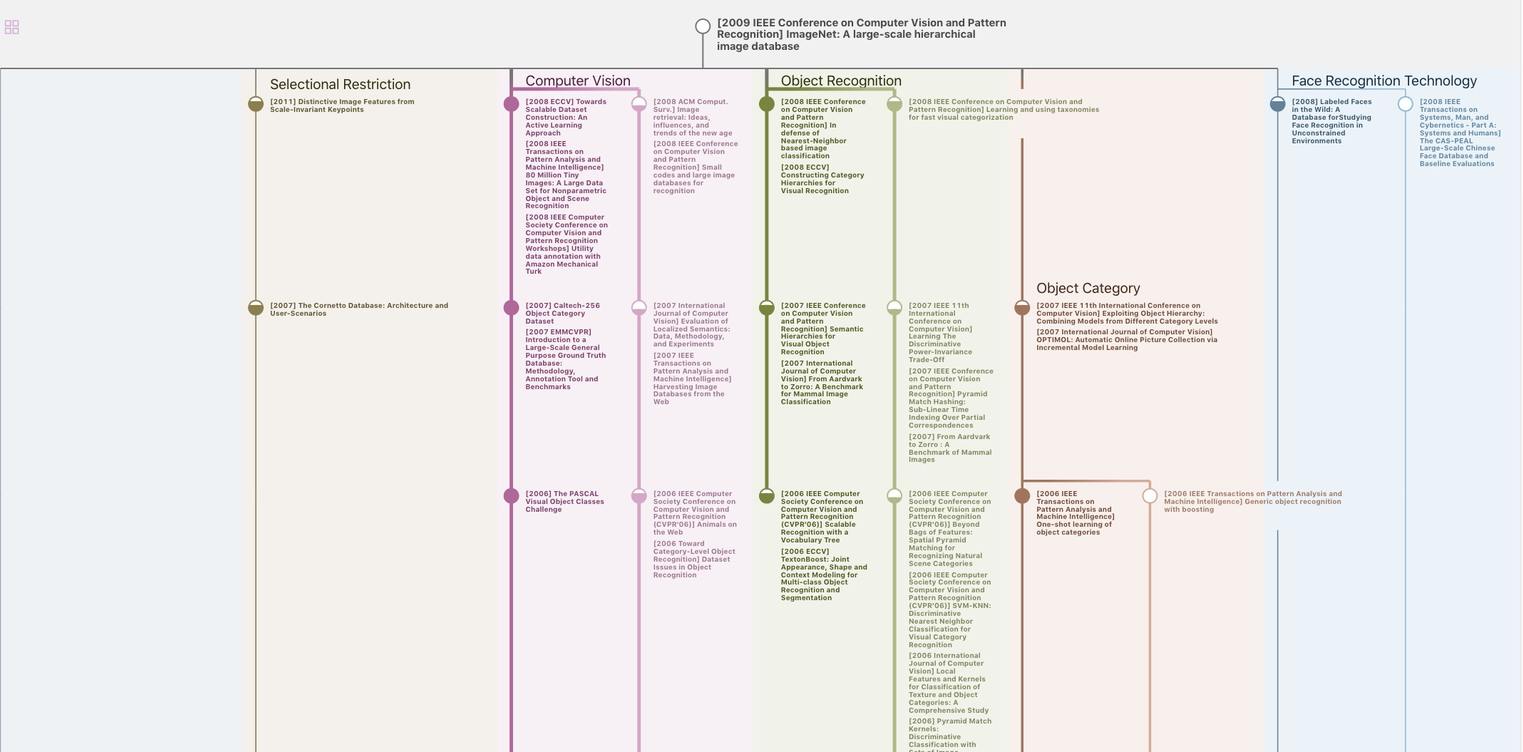
生成溯源树,研究论文发展脉络
Chat Paper
正在生成论文摘要