Using graded-relevance metrics for evaluating community QA answer selection.
WSDM(2011)
摘要
ABSTRACTCommunity Question Answering (CQA) sites such as Yahoo! Answers have emerged as rich knowledge resources for information seekers. However, answers posted to CQA sites can be irrelevant, incomplete, redundant, incorrect, biased, ill-formed or even abusive. Hence, automatic selection of "good" answers for a given posted question is a practical research problem that will help us manage the quality of accumulated knowledge. One way to evaluate answer selection systems for CQA would be to use the Best Answers (BAs) that are readily available from the CQA sites. However, BAs may be biased, and even if they are not, there may be other good answers besides BAs. To remedy these two problems, we propose system evaluation methods that involve multiple answer assessors and graded-relevance information retrieval metrics. Our main findings from experiments using the NTCIR-8 CQA task data are that, using our evaluation methods, (a) we can detect many substantial differences between systems that would have been overlooked by BA-based evaluation; and (b) we can better identify hard questions (i.e. those that are handled poorly by many systems and therefore require focussed investigation) compared to BAbased evaluation. We therefore argue that our approach is useful for building effective CQA answer selection systems despite the cost of manual answer assessments.
更多查看译文
AI 理解论文
溯源树
样例
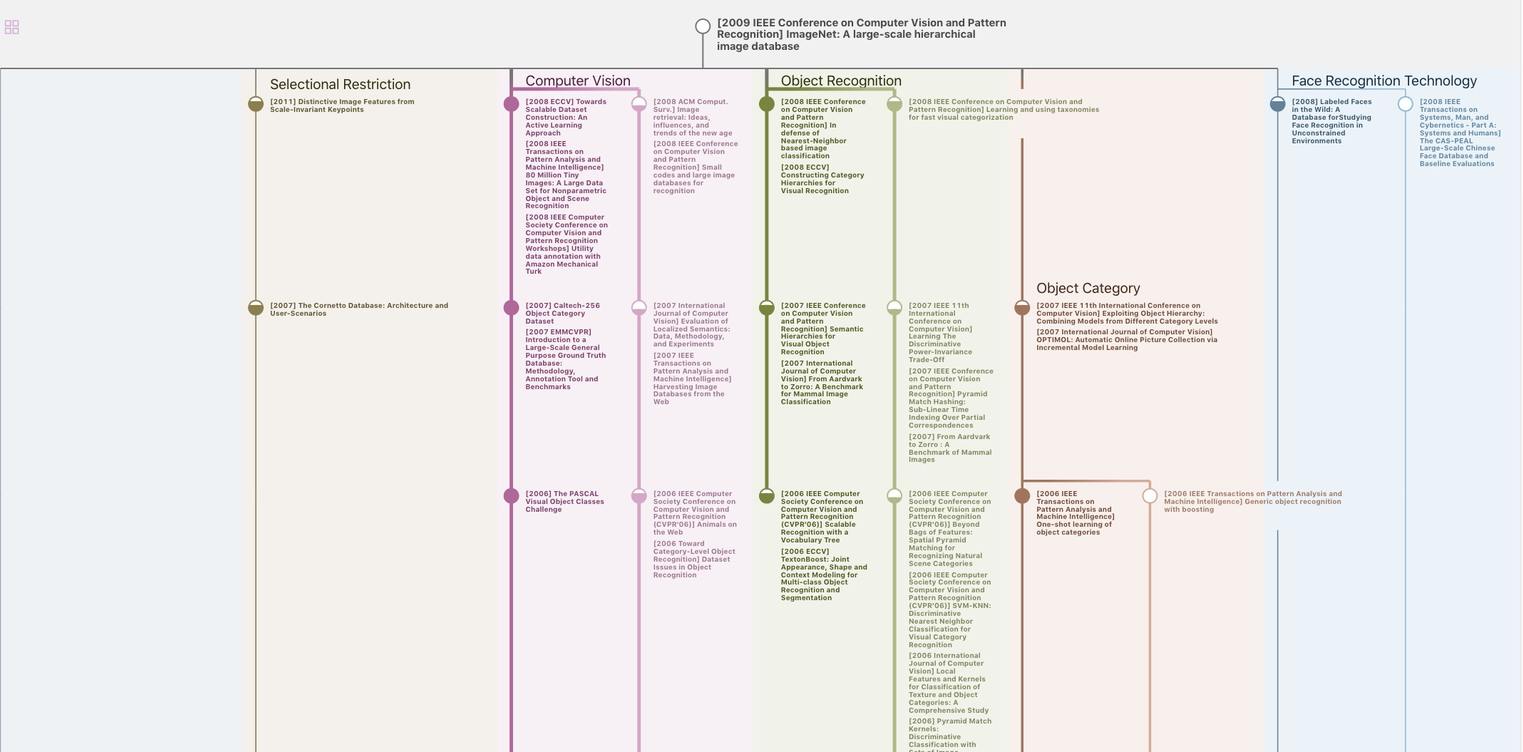
生成溯源树,研究论文发展脉络
Chat Paper
正在生成论文摘要