Automatic Construction Of Hierarchical Hidden Markov Model Structure For Discovering Semantic Patterns In Motion Data
VISAPP 2010: PROCEEDINGS OF THE INTERNATIONAL CONFERENCE ON COMPUTER VISION THEORY AND APPLICATIONS, VOL 1(2010)
摘要
The objective of this paper is to automatically build a Hierarchical Hidden Markov Model (HHMM) (Fine et al., 1998) structure to detect semantic patterns from data with an unknown structure by exploring the natural hierarchical decomposition embedded in the data. The problem is important for effective motion data representation and analysis in a variety of applications: film and game making, military, entertainment, sport and medicine. We propose to represent the patterns of the data as an HHMM built utilising a two-stage learning algorithm. The novelty of our method is that it is the first fully automated approach to build an HHMM structure for motion data. Experimental results on different motion features (3D and angular pose coordinates, silhouettes extracted from the video sequence) demonstrate the approach is effective at automatically constructing efficient HHMM with a structure which naturally represents the underlying motion that allows for accurate modelling of the data for applications such as tracking and motion resynthesis.
更多查看译文
关键词
HHMM structure,Pattern recognition,Motion analysis
AI 理解论文
溯源树
样例
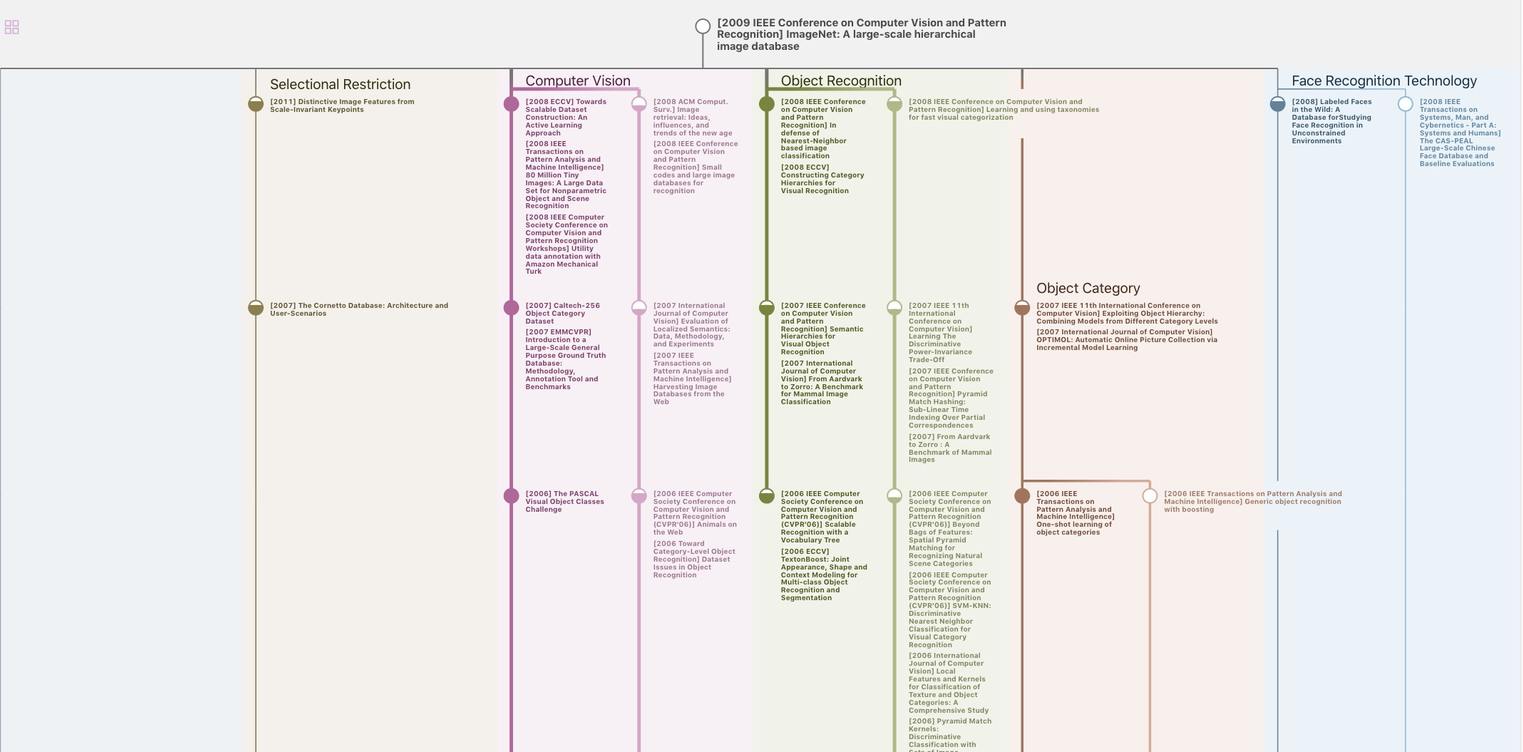
生成溯源树,研究论文发展脉络
Chat Paper
正在生成论文摘要