A Recurrence-Relation-Based Reward Model for Performability Evaluation of Embedded Systems
2008 IEEE International Conference on Dependable Systems and Networks With FTCS and DCC (DSN)(2008)
Abstract
Embedded systems for closed-loop applications often behave as discrete-time semi-Markov processes (DTSMPs). Performability measures most meaningful to iterative embedded systems, such as accumulated reward, are thus difficult to solve analytically in general. In this paper we propose a recurrence-relation-based (RRB) reward model to evaluate such measures. A critical element in RRB reward models is the notion of state-entry probability. This notion enables us to utilize the embedded Markov chain in a DTSMP in a novel way. More specifically, we formulate state-entry probabilities, state-occupancy probabilities, and expressions concerning accumulated reward solely in terms of state-entry probability and its companion term, namely the expected accumulated reward at the point of state entry. As a result, recurrence relations abstract away all the intermediate points that lack the memoryless property, enabling a solvable model to be directly built upon the embedded Markov chain. To show the usefulness of RRB reward models, we evaluate an embedded system for which we leverage the proposed notion and methods to solve a variety of probabilistic measures analytically.
MoreTranslated text
Key words
Markov processes,discrete time systems,embedded systems,probability,software performance evaluation,discrete-time semiMarkov process,iterative embedded systems,performability evaluation,recurrence-relation-based reward model,state-entry probability,state-occupancy probability
AI Read Science
Must-Reading Tree
Example
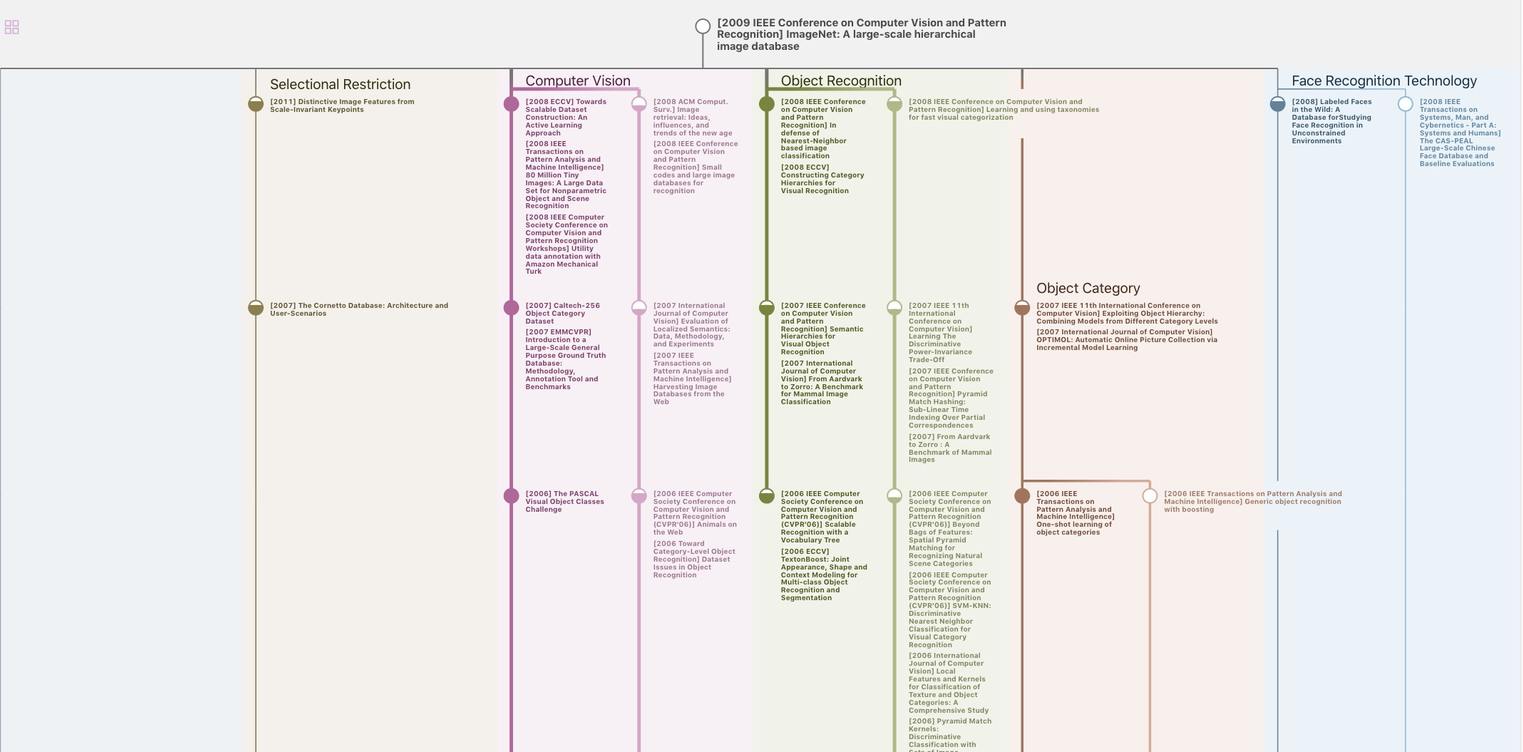
Generate MRT to find the research sequence of this paper
Chat Paper
Summary is being generated by the instructions you defined