Real-Time Large-Scale Visual Concept Detection With Linear Classifiers
International Conference on Pattern Recognition(2012)
摘要
Many emerging application areas in video and image processing require real-time or faster visual concept detection. Examples include indexing of online user-generated video content and 24/7 archiving of TV broadcasts. The current state-of-the-art in concept detection uses bag-of-visual-words features with computationally heavy kernel-based classifiers. We argue that this approach is not feasible for real-time applications, and propose instead to use combinations of fast linear classifiers. In experiments with the large-scale TRECVID 2011 video database and 50 concepts, we compare several methods to improve the retrieval performance of standard linear classifiers. Fusing classifiers trained on different features and using multi-learn and homogeneous kernel maps achieve state-of-the-art retrieval precision, while retaining real-time performance even for large sets of concepts.
更多查看译文
关键词
image classification,video databases,video signal processing,TV broadcasts archiving,bag-of-visual-words features,computationally heavy kernel-based classifiers,fast linear classifiers,homogeneous kernel maps,image processing,large-scale TRECVID 2011 video database,multilearn kernel maps,online user-generated video content indexing,realtime large-scale visual concept detection,standard linear classifiers,video processing,
AI 理解论文
溯源树
样例
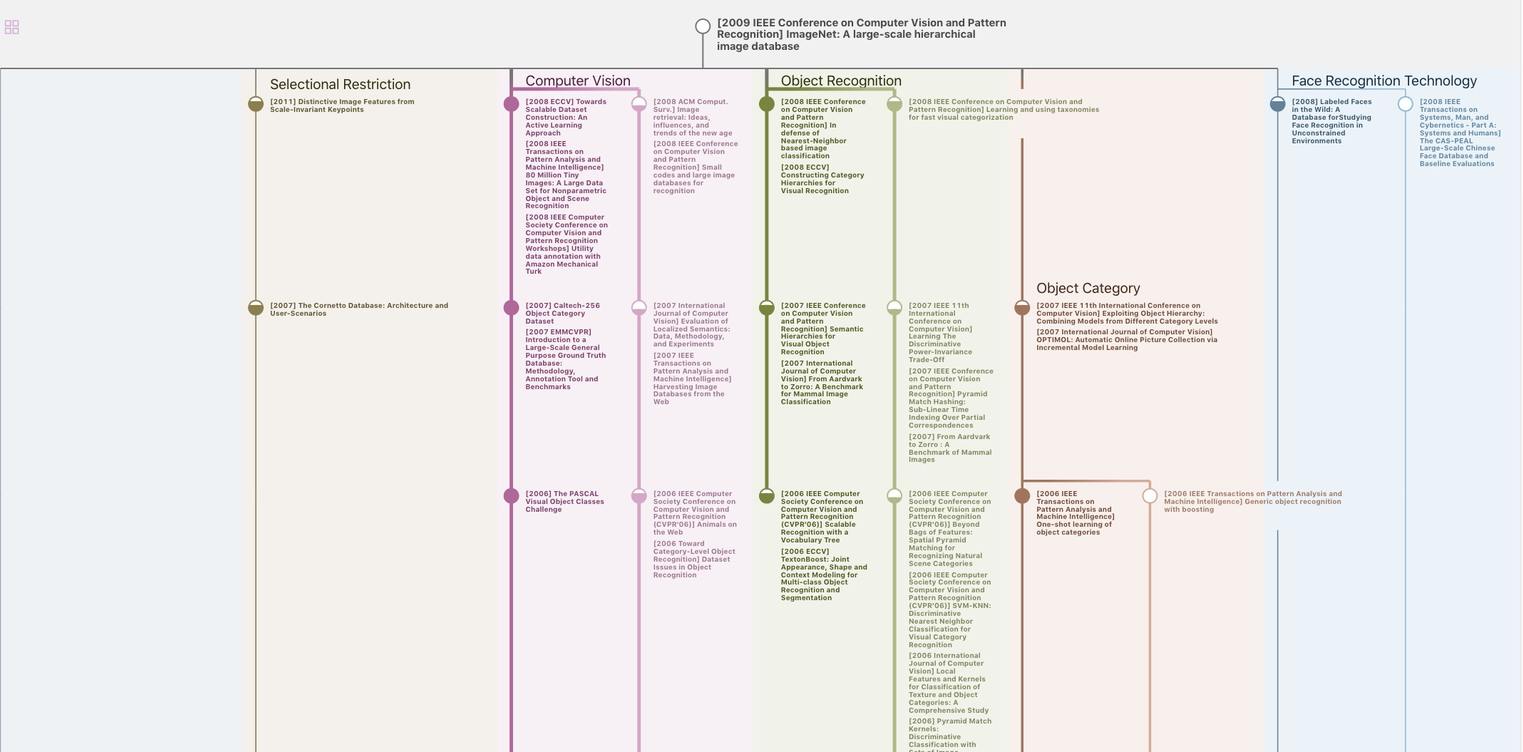
生成溯源树,研究论文发展脉络
Chat Paper
正在生成论文摘要