Categorization of display ads using image and landing page features
Proceedings of the Third Workshop on Large Scale Data Mining: Theory and Applications(2011)
摘要
We consider the problem of automatically categorizing display ad images into a taxonomy of relevant interest categories. In particular, we focus on the efficacy of using image features extracted by OCR techniques from the ad images, in addition to the features from the text in the title, keywords and body of the landing page of the ad, and the features of the advertiser, in predicting the category of the display ad. An automated ad categorization tool has multiple uses in display advertising including increasing the ad categorization coverage, scaling up the ad categorization capacity to handle large volumes of ads by reducing the amount of human editorial effort and better utilizing the human editorial experts to focus on categorizing difficult ads. The ad image and landing page features extracted in this ad categorization system can also be used to improve the matching and ranking steps of ad selection algorithms in display ad serving systems. We learn multiple one-versus-rest SVM models to categorize the display ads, from a historical dataset of ads labeled into these categories by human editors. The OCR features extracted by common open source tools are by themselves noisy, and models trained using only the OCR features are not competitive with the performance of models trained using the landing page features. However, for categories with a small number of training examples, the OCR features improve the categorization performance metrics when used in addition to the features from the landing page. The OCR features also provide a useful signal to predict the category of an ad when features from the landing pages are not available. Our models have an average precision of 0.6 and recall of 0.37 over more than 1200 categories when evaluated on a hold out dataset. The precision and recall values are considerably higher for categories with larger amounts of training data, with precision larger than 0.84 and recall larger than 0.7 in all the categories that have more than 100,000 samples in the training dataset. Features from the text in the body of the landing page of the ads increase the recall of the categorization models and to a lesser extent increase the precision of these models, especially in categories with a smaller number of training samples.
更多查看译文
关键词
display ad,difficult ad,landing page,landing page feature,automated ad categorization tool,ad categorization coverage,categorizing display ad image,ad image,ad categorization capacity,ad categorization system,ad selection algorithm,feature extraction,multi class classification,image features
AI 理解论文
溯源树
样例
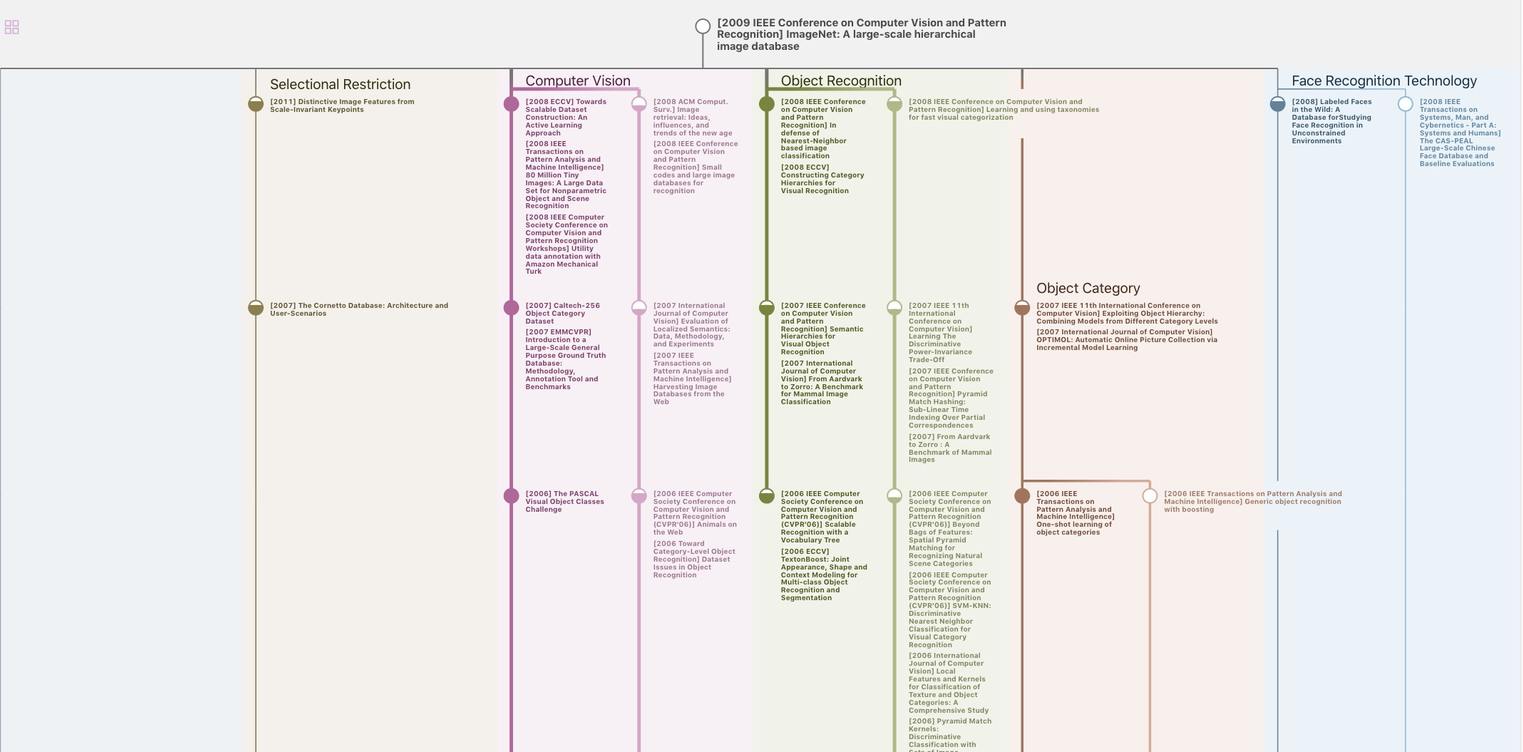
生成溯源树,研究论文发展脉络
Chat Paper
正在生成论文摘要