A Unified View of Kernel k-means, Spectral Clustering and Graph Cuts
msra(2004)
摘要
Recently, a variety of clustering algorithms have been proposed to handle data that is not linearly separable. Spectral clustering and kernel k-means are two such methods that are seemingly quite different. In this paper, we show that a general weighted kernel k-means objective is mathematically equivalent to a weighted graph partitioning objective. Special cases of this graph partitioning objective include ratio cut, normalized cut and ratio association. Our equivalence has important consequences: the weighted kernel k-means algorithm may be used to directly optimize the graph partitioning objectives, and conversely, spectral methods may be used to optimize the weighted kernel k-means objective. Hence, in cases where eigenvector computation is prohibitive, we eliminate the need for any eigenvector computation for graph partitioning. Moreover, we show that the Kernighan-Lin objective can also be incorporated into our framework, leading to an incremental weighted kernel k-means algorithm for local optimization of the objective. We further discuss the issue of convergence of weighted kernel k-means for an arbitrary graph affinity matrix and provide a number of experimental results. Theseresults show that non-spectral methods for graph partitioning are as effective as spectral methods and can be used for problems such as image segmentation in addition to data clustering.
更多查看译文
关键词
eigenvectors,spectral methods,kernel k-means,trace maxi- mization,graph partitioning,clustering
AI 理解论文
溯源树
样例
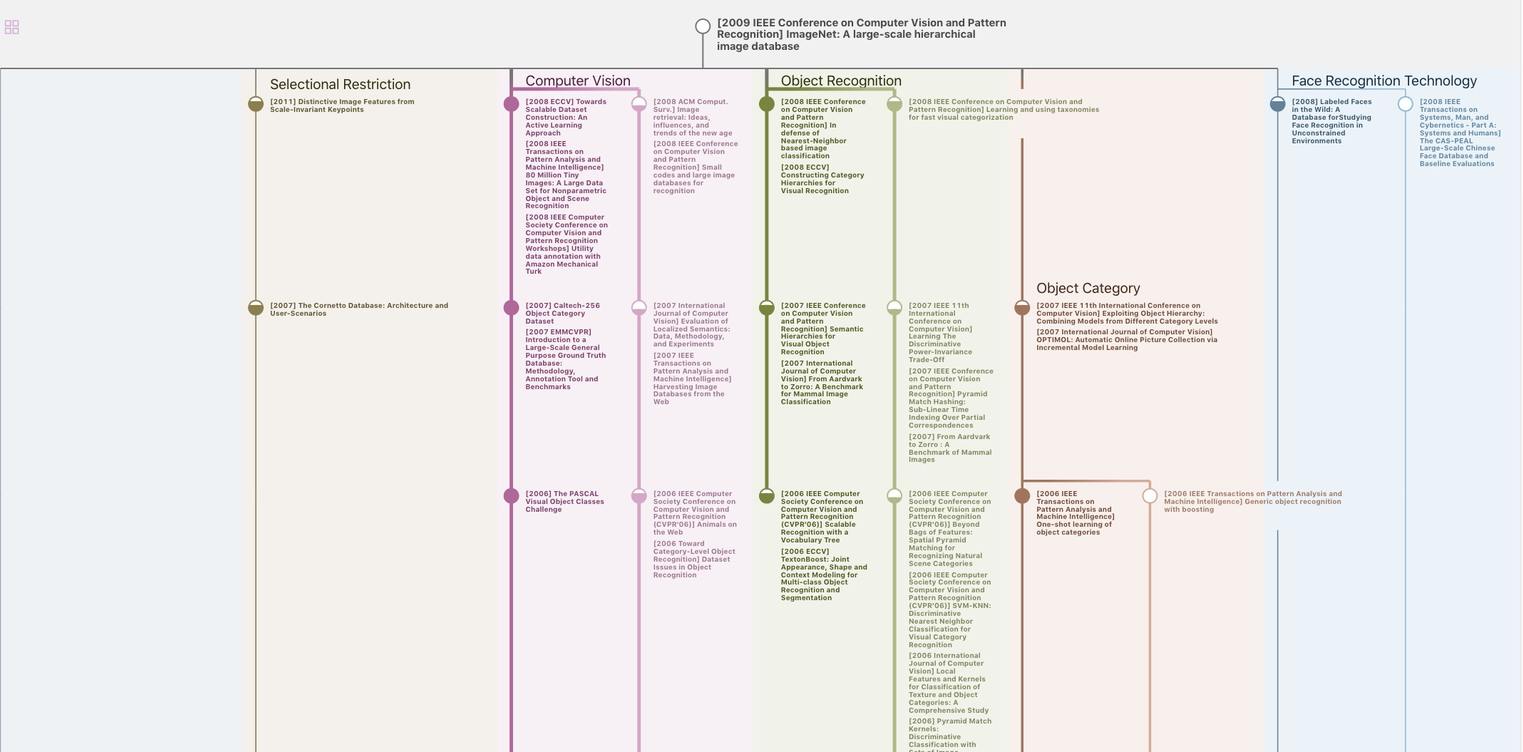
生成溯源树,研究论文发展脉络
Chat Paper
正在生成论文摘要