Dimension Reduction In Regression Using Gaussian Mixture Models
ICASSP(2012)
摘要
Linear-Nonlinear regression models play a fundamental role in characterizing nonlinear systems. In this paper, we propose a method to estimate the linear transform in such models equivalent to a subspace of a small dimension in the input space that is relevant for eliciting response. The novel aspect of this work is the formulation of the mutual information between the transformed inputs and output as a closed-form function of the parameters of their joint density in the form of Gaussian Mixture Models and we subsequently maximize this measure to find relevant dimensions. Instead of a commonly used mutual information measure based on Kullback-Leibler divergence, we use a measure called Quadratic Euclidean Mutual Information. Through experiments on both synthesized data and real MEG recordings, the effectiveness of the proposed method is demonstrated.
更多查看译文
关键词
dimension reduction,regression,mutual information,gaussian mixture models
AI 理解论文
溯源树
样例
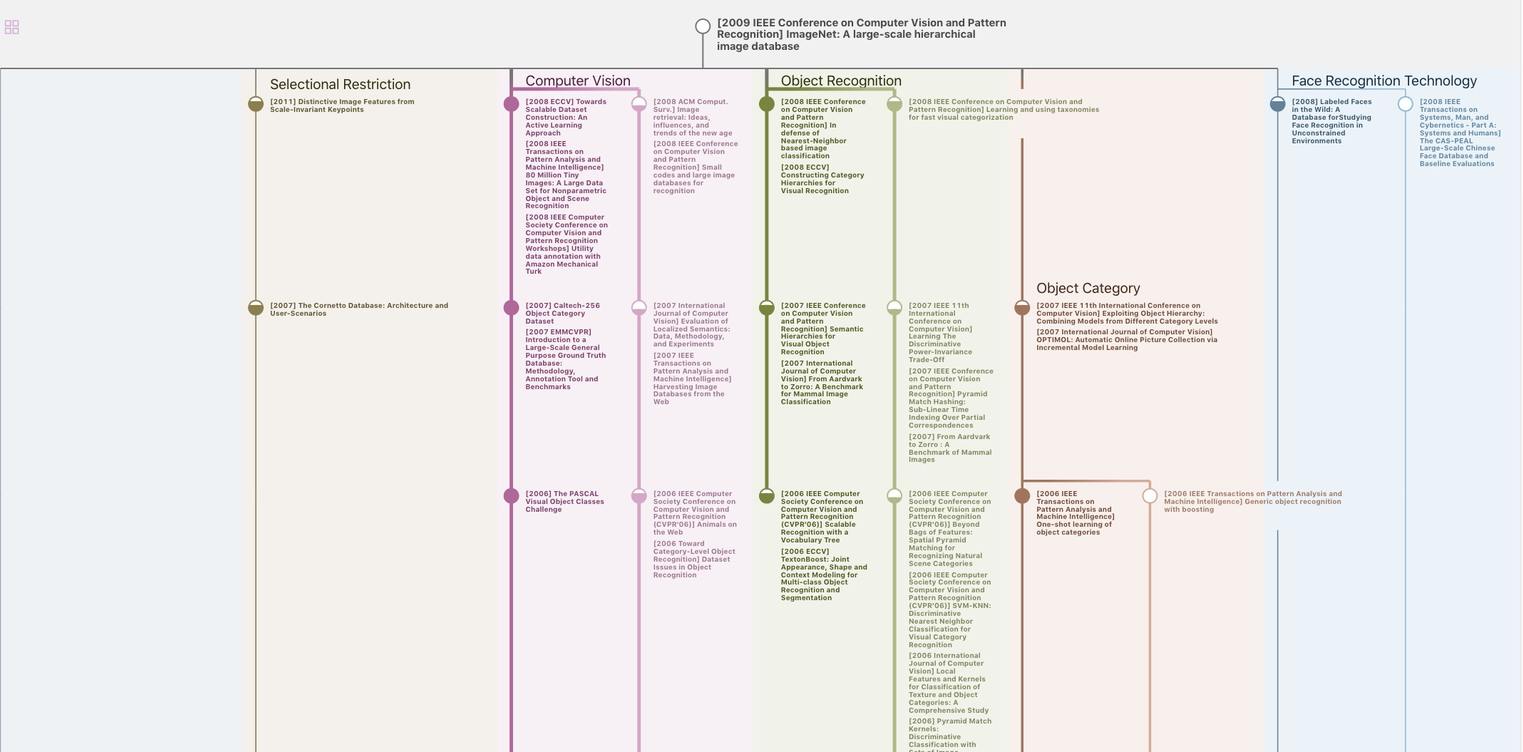
生成溯源树,研究论文发展脉络
Chat Paper
正在生成论文摘要