Spatio-temporal compressive sensing and internet traffic matrices
SIGCOMM(2009)
摘要
Many basic network engineering tasks (e.g., traffic engineering, capacity planning, anomaly detection) rely heavily on the availability and accuracy of traffic matrices. However, in practice it is challenging to reliably measure traffic matrices. Missing values are common. This observation brings us into the realm of compressive sensing, a generic technique for dealing with missing values that exploits the presence of structure and redundancy in many real-world systems. Despite much recent progress made in compressive sensing, existing compressive-sensing solutions often perform poorly for traffic matrix interpolation, because real traffic matrices rarely satisfy the technical conditions required for these solutions. To address this problem, we develop a novel spatio-temporal compressive sensing framework with two key components: (i) a new technique called Sparsity Regularized Matrix Factorization (SRMF) that leverages the sparse or low-rank nature of real-world traffic matrices and their spatio-temporal properties, and (ii) a mechanism for combining low-rank approximations with local interpolation procedures. We illustrate our new framework and demonstrate its superior performance in problems involving interpolation with real traffic matrices where we can successfully replace up to 98% of the values. Evaluation in applications such as network tomography, traffic prediction, and anomaly detection confirms the flexibility and effectiveness of our approach.
更多查看译文
关键词
compressive sensing,traffic matrix,interpolation,tomography,prediction,anomaly detection
AI 理解论文
溯源树
样例
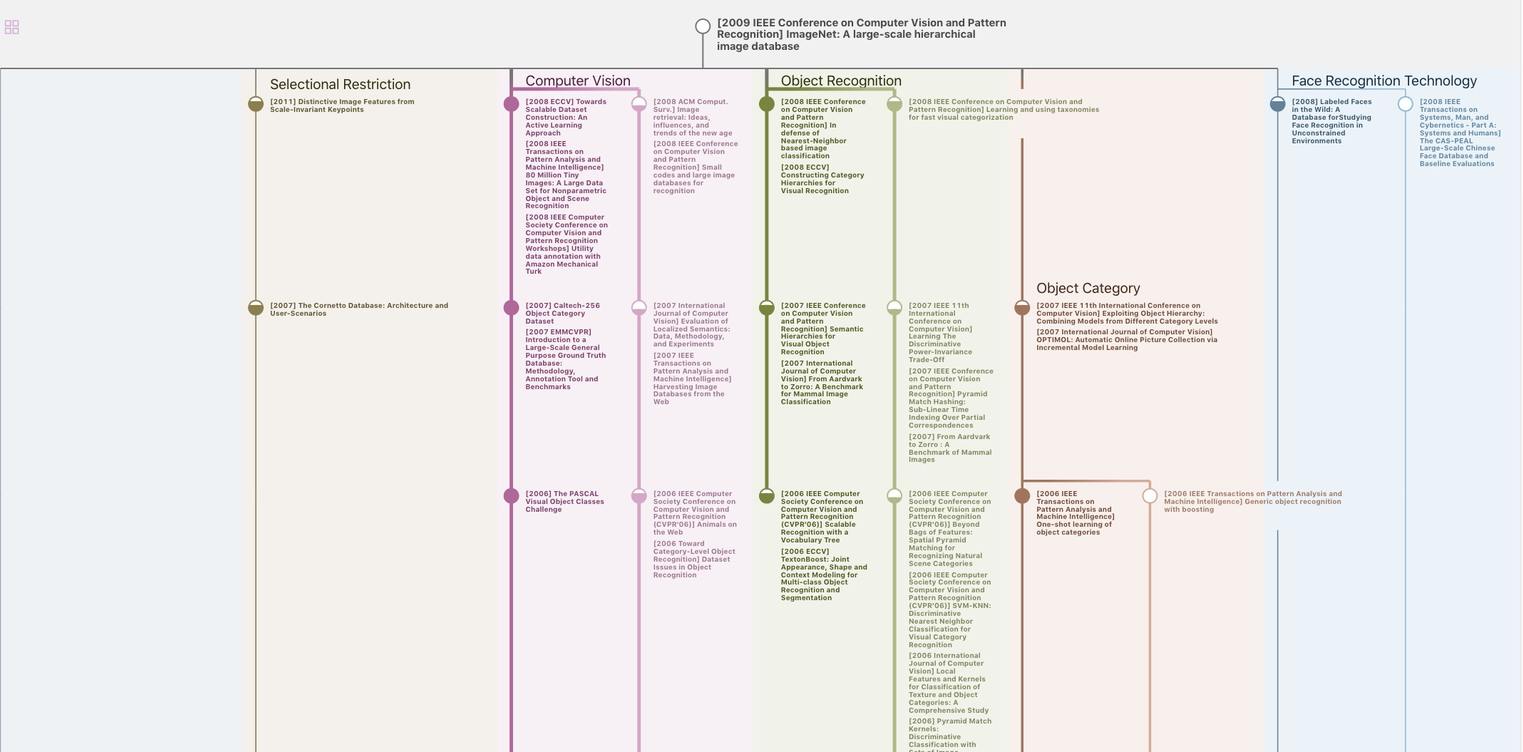
生成溯源树,研究论文发展脉络
Chat Paper
正在生成论文摘要