A two-stage approach to domain adaptation for statistical classifiers.
CIKM(2007)
摘要
ABSTRACTIn this paper, we consider the problem of adapting statistical classifiers trained from some source domains where labeled examples are available to a target domain where no labeled example is available. One characteristic of such a domain adaptation problem is that the examples in the source domains and the target domain are known to follow different distributions. Thus a regular classification method would tend to overfit the source domains. We present a two-stage approach to domain adaptation, where at the first stage, we look for a set of features generalizable across domains, and at the second adaptation stage, we pick up useful features specific to the target domain. Observing that the exact objective function is hard to optimize, we then propose a number of heuristics to approximately achieve the goal of generalization and adaptation. Our experiments on gene name recognition using a real data set show the effectiveness of our general framework and the heuristics.
更多查看译文
AI 理解论文
溯源树
样例
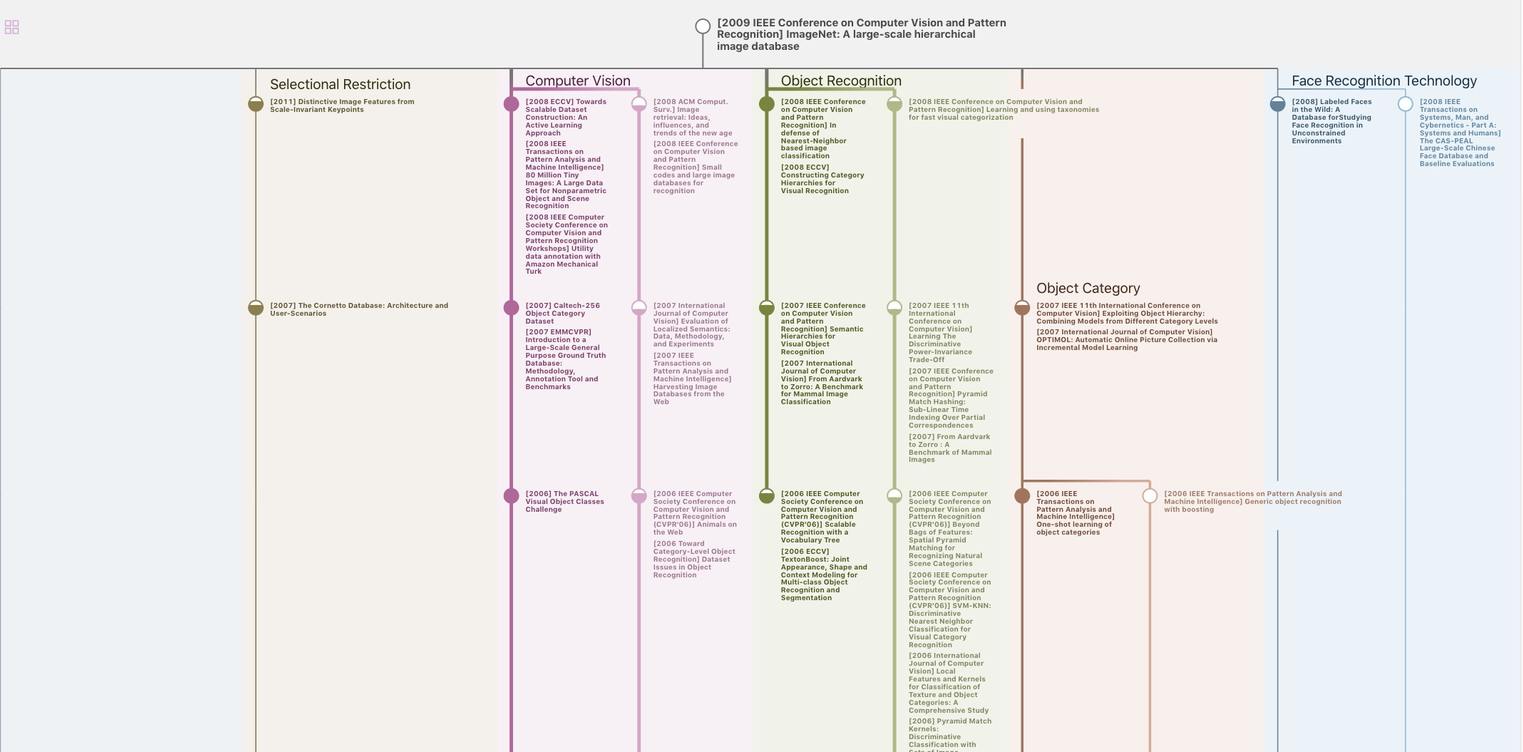
生成溯源树,研究论文发展脉络
Chat Paper
正在生成论文摘要