Learning To Infer Social Ties In Large Networks
ECMLPKDD'11: Proceedings of the 2011th European Conference on Machine Learning and Knowledge Discovery in Databases - Volume Part III(2011)
摘要
In online social networks, most relationships are lack of meaning labels (e. g., "colleague" and "intimate friends"), simply because users do not take the time to label them. An interesting question is: can we automatically infer the type of social relationships in a large network? what are the fundamental factors that imply the type of social relationships? In this work, we formalize the problem of social relationship learning into a semi-supervised framework, and propose a Partially-labeled Pairwise Factor Graph Model (PLP-FGM) for learning to infer the type of social ties. We tested the model on three different genres of data sets: Publication, Email and Mobile. Experimental results demonstrate that the proposed PLP-FGM model can accurately infer 92.7% of advisor-advisee relationships from the coauthor network (Publication), 88.0% of manager-subordinate relationships from the email network (Email), and 83.1% of the friendships from the mobile network (Mobile). Finally, we develop a distributed learning algorithm to scale up the model to real large networks.
更多查看译文
关键词
social relationship,online social network,social tie,coauthor network,email network,large network,mobile network,real large network,proposed PLP-FGM model,Graph Model
AI 理解论文
溯源树
样例
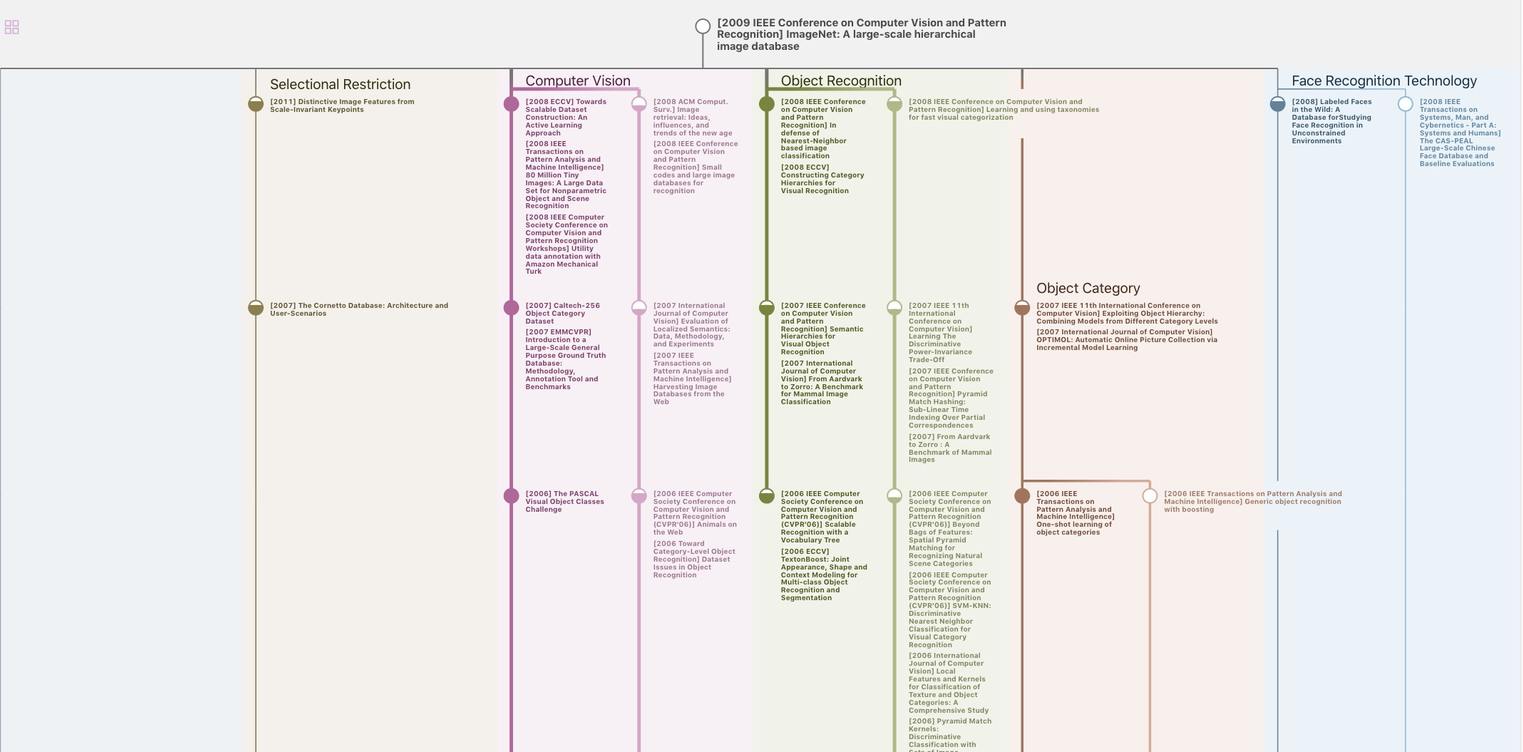
生成溯源树,研究论文发展脉络
Chat Paper
正在生成论文摘要