Application of machine learning approaches on quantitative structure activity relationships
CIBCB(2009)
摘要
Machine Learning techniques are successfully applied to establish quantitative relations between chemical structure and biological activity (QSAR), i.e. classify compounds as active or inactive with respect to a specific target biological system. This paper presents a comparison of Artificial Neural Networks (ANN), Support Vector Machines (SVM), and Decision Trees (DT) in an effort to identify potentiators of metabotropic glutamate receptor 5 (mGluR5), compounds that have potential as novel treatments against schizophrenia. When training and testing each of the three techniques on the same dataset enrichments of 61, 64, and 43 were obtained and an area under the curve (AUC) of 0.77, 0.78, and 0.63 was determined for ANNs, SVMs, and DTs, respectively. For the top percentile of predicted active compounds, the true positives for all three methods were highly similar, while the inactives were diverse offering the potential use of jury approaches to improve prediction accuracy.
更多查看译文
关键词
decision trees,drugs,learning (artificial intelligence),medical computing,neural nets,support vector machines,ANN,SVM,area under the curve,artificial neural networks,biological activity,chemical structure,decision trees,machine learning,metabotropic glutamate receptor 5,quantitative structure activity relationships,schizophrenia,support vector machines,Artificial Neural Network (ANN),Decision Trees (DT),Machine Learning,Support Vector Machine (SVM),area under the curve (AUC),high-throughput screening (HTS),quantitative structure activity relationship (QSAR),receiver operator characteristics (ROC)
AI 理解论文
溯源树
样例
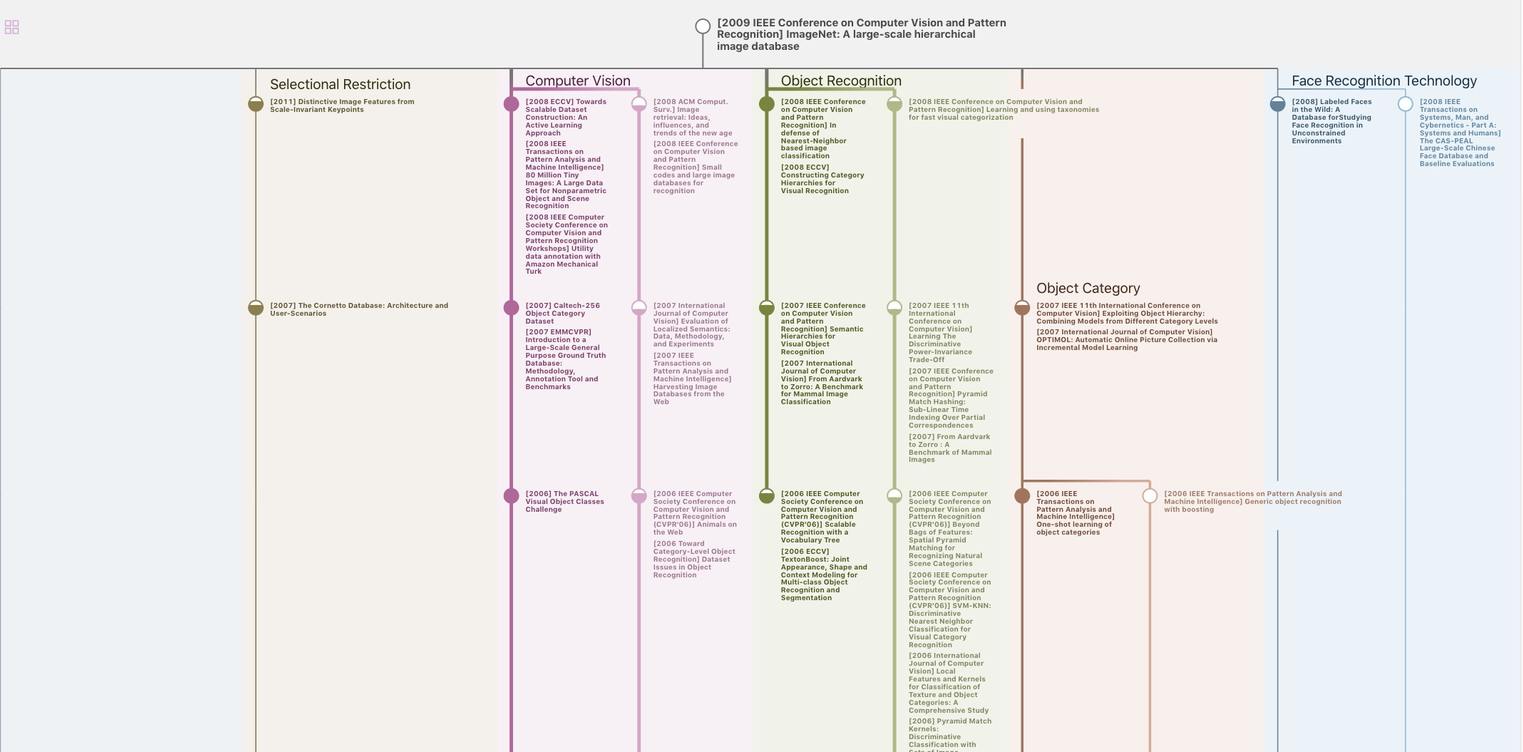
生成溯源树,研究论文发展脉络
Chat Paper
正在生成论文摘要