Using compressive sensing to reduce fingerprint collection for indoor localization
WCNC(2013)
摘要
Many WLAN-based indoor localization techniques estimate a target's location by comparing received signal strength indicator (RSSI) with stored fingerprints. However, the collection of fingerprints is notoriously tedious and time-consuming. It is challenging to reduce the fingerprint collection and recover absent data without introducing errors. In this article, a new approach based on compressive sensing is presented for recovering absent fingerprints. The hidden structure and redundancy characteristics of fingerprints are revealed in the Merging Matrix. The spatial and temporal relativity of fingerprints leads the rank of the Merging Matrix to be small. But the multipath effect in indoor environments conceals the nature of the matrix. The algorithm Sparsity Rank Singular Value Decomposition (SRSVD) can clear away the interference. Experiment results show that using 10% of the data can recover all of the fingerprint information with error rate less than 16%. The localization accuracy with the recovered fingerprints is similar to the one with the original complete fingerprints.
更多查看译文
关键词
stored fingerprints,merging matrix,sparsity rank singular value decomposition,fingerprint information,indoor localization,fingerprint collection,interpolation,fingerprint collection reduction,localization accuracy,compressive sensing,fingerprints temporal relativity,rssi,received signal strength indicator,srsvd,fingerprints spatial relativity,indoor environments,wireless lan,wlan-based indoor localization techniques,singular value decomposition,indoor radio,compressed sensing,merging,fingerprint recognition,sparse matrices,matrix decomposition
AI 理解论文
溯源树
样例
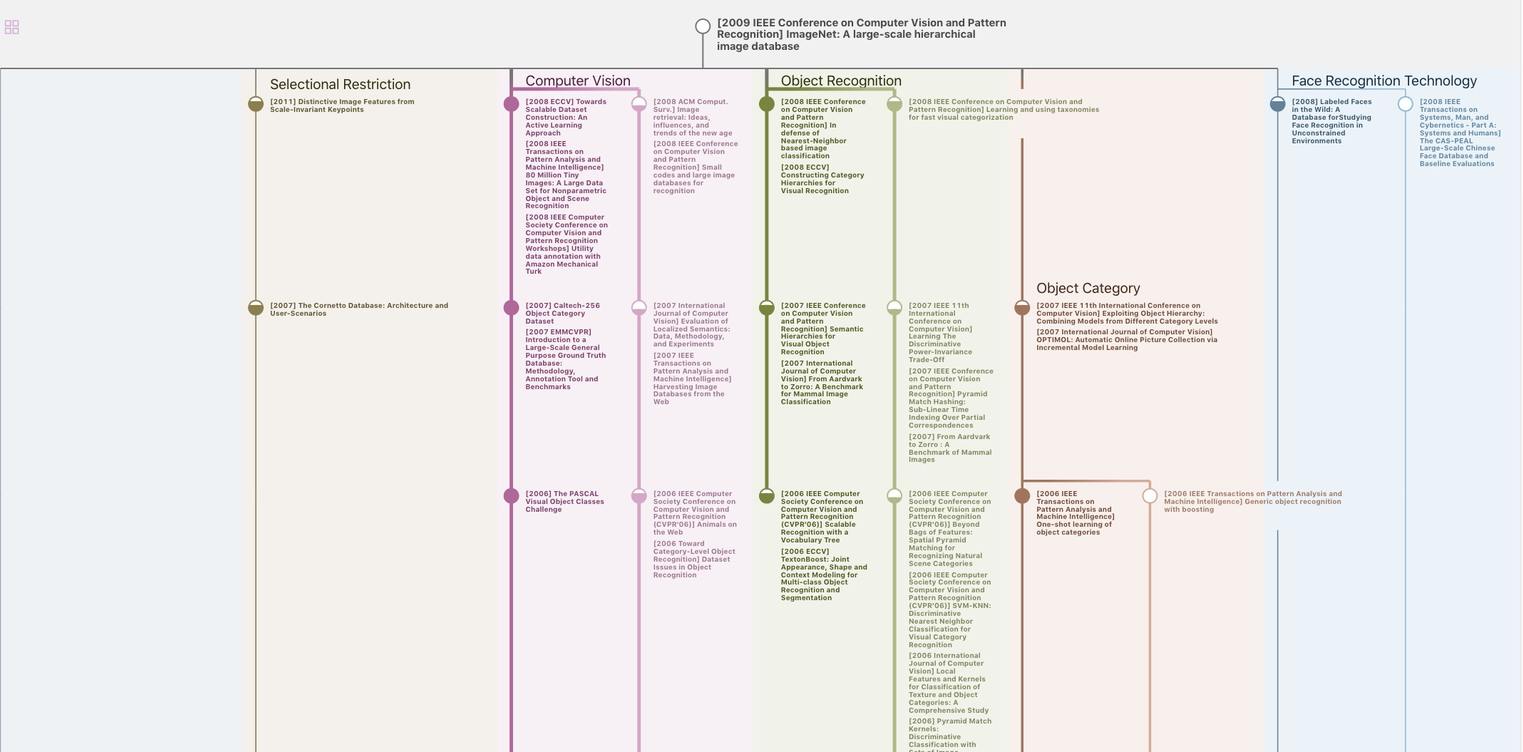
生成溯源树,研究论文发展脉络
Chat Paper
正在生成论文摘要