How Concrete Do We Get Telling Stories?
TOPICS IN COGNITIVE SCIENCE(2018)
摘要
Will reading different stories about the same event in the world result in a similar image of the world? Will reading the same story by different people result in a similar proxy for experiencing the story? The answer to both questions is no because language is abstract by definition and relies on our episodic experience to turn a story into a more concrete mental movie. Since our episodic knowledge differs, also the mental movie will be different. Language leaves out details, and this becomes specifically clear when building machines that read texts to represent events and to establish event relations across mentions, such as co-reference, causality, subevents, scripts, timelines, and storylines. There is a lot of information and knowledge on the event that is not in the text but is needed to reconstruct these relations and understand the story. Machines lack this knowledge and experience and likewise make explicit what it takes to understand stories from text. In this paper, we report on experiments to automatically model event descriptions and instances across different news articles. We will show that event information is scattered over the text but also varies a lot in the degree it abstracts from details, which makes establishing event identity and relations extremely difficult. The variation in granularity of event descriptions seems to vary with pragmatic communicative strategies and defines the problem at different levels of complexity. Abstraction is a process through which we gradually leave out colourful details of individual experiences. Vossen and colleagues present a series of machine-learning algorithms which, when fed with newspapers' texts, automatically identify and represent the events being mentioned in the texts at various levels of granularity. The authors show that the more an event is far in the past, the more the information contained in related news texts becomes less specific and thus more abstract.
更多查看译文
关键词
Event mention,Event instance,Event coreference,Storyline extraction,Computational models
AI 理解论文
溯源树
样例
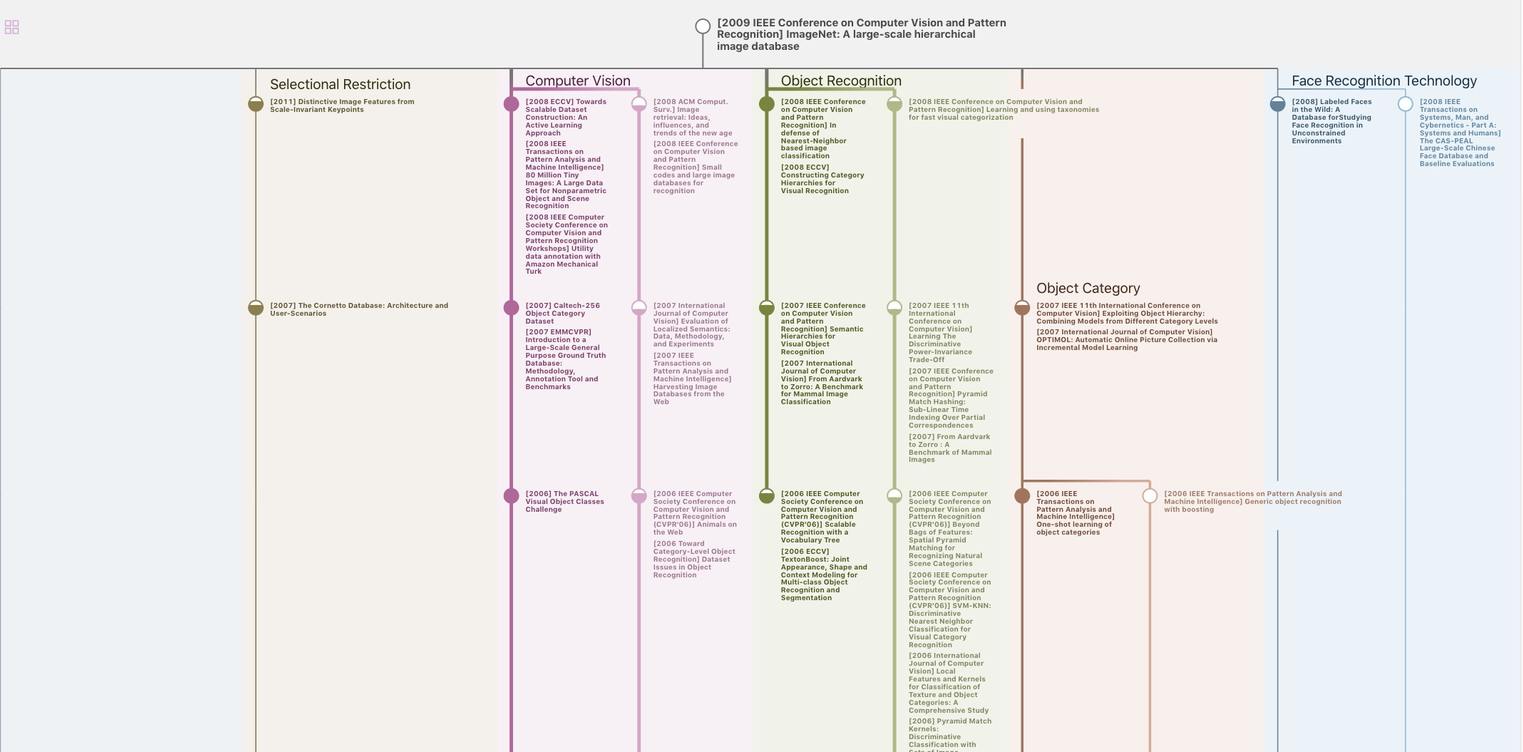
生成溯源树,研究论文发展脉络
Chat Paper
正在生成论文摘要