Graph-Based Semi-Supervised Learning For Phone And Segment Classification
14TH ANNUAL CONFERENCE OF THE INTERNATIONAL SPEECH COMMUNICATION ASSOCIATION (INTERSPEECH 2013), VOLS 1-5(2013)
摘要
This paper presents several novel contributions to the emerging framework of graph-based semi-supervised learning for speech processing. First, we apply graph-based learning to variable-length segments rather than to the fixed-length vector representations that have been used previously. As part of this work we compare various graph-based learners, and we utilize an efficient feature selection technique for high-dimensional feature spaces that alleviates computational costs and improves the performance of graph-based learners. Finally, we present a method to improve regularization during the learning process. Experimental evaluation on the TIMIT frame and segment classification tasks demonstrates that the graph-based classifiers outperform standard baseline classifiers; furthermore, we find that the best learning algorithms are those that can incorporate prior knowledge.
更多查看译文
AI 理解论文
溯源树
样例
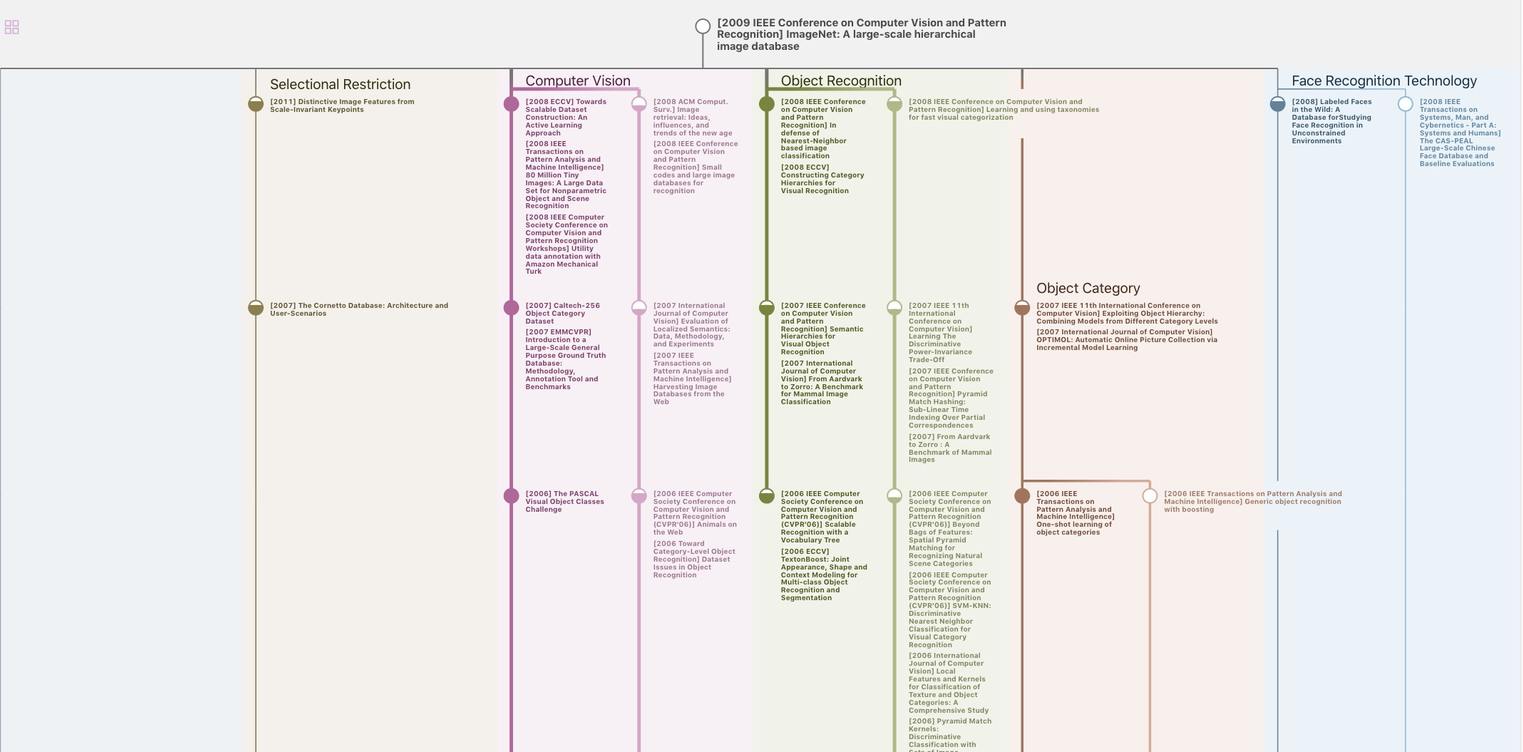
生成溯源树,研究论文发展脉络
Chat Paper
正在生成论文摘要