Hill-Climbing Feature Selection For Multi-Stream Asr
INTERSPEECH 2009: 10TH ANNUAL CONFERENCE OF THE INTERNATIONAL SPEECH COMMUNICATION ASSOCIATION 2009, VOLS 1-5(2009)
摘要
We performed automated feature selection for multi-stream (i.e., ensemble) automatic speech recognition, using a hill-climbing (HC) algorithm that changes one feature at a time if the change improves a performance score. For both clean and noisy data sets (using the OGI Numbers corpus), HC usually improved performance on held out data compared to the initial system it started with, even for noise types that were not seen during the HC process. Overall, we found that using Opitz's scoring formula, which blends single-classifier word recognition accuracy and ensemble diversity, worked better than ensemble accuracy as a performance score for guiding HC in cases of extreme mismatch between the SNR of training and test sets.Our noisy version of the Numbers corpus, our multi-layer-perceptron-based Numbers ASR system, and our HC scripts are available online.
更多查看译文
关键词
speech recognition, feature selection, ensemble
AI 理解论文
溯源树
样例
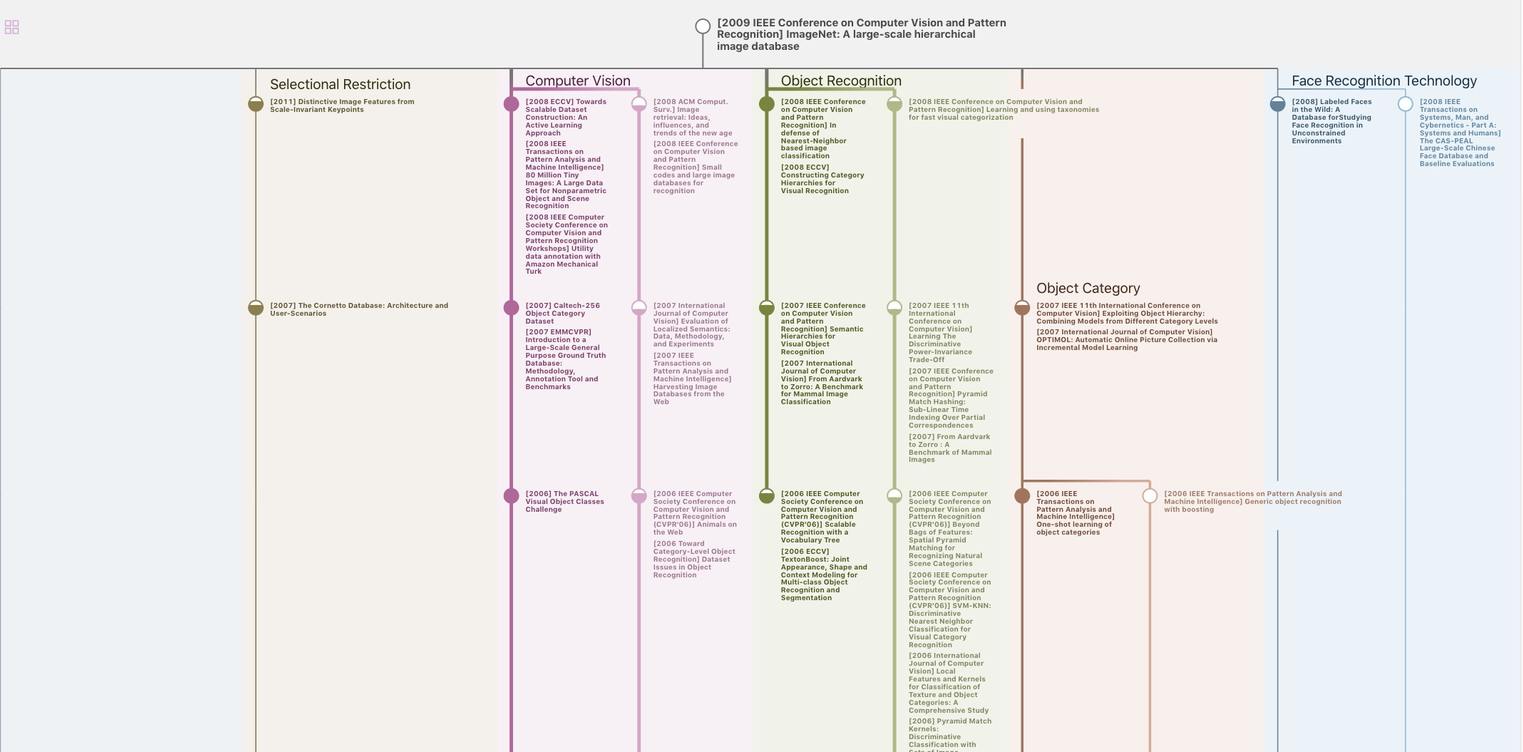
生成溯源树,研究论文发展脉络
Chat Paper
正在生成论文摘要