Support Vector Machine-Based Classification Of Rock Texture Images Aided By Efficient Feature Selection
2012 INTERNATIONAL JOINT CONFERENCE ON NEURAL NETWORKS (IJCNN)(2012)
摘要
This paper presents a study on rock texture image classification using support vector machines (and also K-nearest neighbours and decision trees) with the aid of feature selection techniques. It offers both unsupervised and supervised methods for feature selection, based on data reliability and information gain ranking respectively. Following this approach, the conventional classifiers which are sensitive to the dimensionality of feature patterns, become effective on classification of images whose pattern representation may otherwise involve a large number of features. The work is successfully applied to complex images. Classifiers built using features selected by either of these methods generally outperform their counterparts that employ the full set of original features which has a dimensionality several folds higher than that of the selected feature subset. This is confirmed by systematic experimental investigations. This study therefore, helps to accomplish challenging image classification tasks effectively and efficiently. In particular, the approach retains the underlying semantics of a selected feature subset. This is very important to ensure that the classification results are understandable by the user.
更多查看译文
关键词
geology,image classification,image texture,rocks,support vector machines,conventional classifiers,data reliability,feature pattern dimensionality,feature selection techniques,information gain ranking,rock texture image classification,support vector machine-based classification,unsupervised methods,
AI 理解论文
溯源树
样例
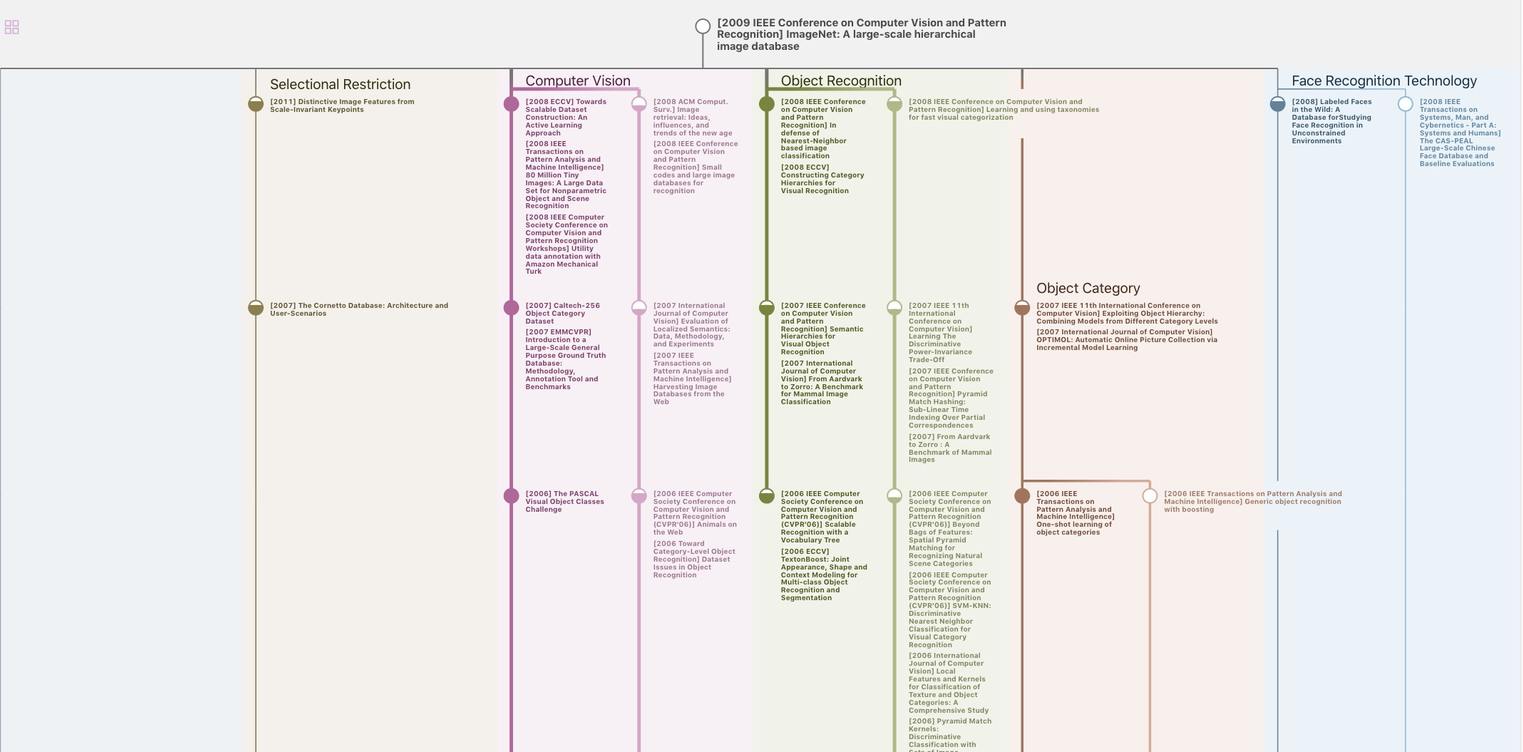
生成溯源树,研究论文发展脉络
Chat Paper
正在生成论文摘要