Topological transformation approaches to TCAM-based packet classification
IEEE/ACM Trans. Netw.(2011)
摘要
Several range reencoding schemes have been proposed to mitigate the effect of range expansion and the limitations of small capacity, large power consumption, and high heat generation of ternary content addressable memory (TCAM)-based packet classification systems. However, they all disregard the semantics of classifiers and therefore miss significant opportunities for space compression. In this paper, we propose new approaches to range reencoding by taking into account classifier semantics. Fundamentally different from prior work, we view reencoding as a topological transformation process from one colored hyperrectangle to another, where the color is the decision associated with a given packet. Stated another way, we reencode the entire classifier by considering the classifier's decisions rather than reencode only ranges in the classifier ignoring the classifier's decisions as prior work does. We present two orthogonal, yet composable, reencoding approaches: domain compression and prefix alignment. Our techniques significantly outperform all previous reencoding techniques. In comparison to prior art, our experimental results show that our techniques achieve at least five times more space reduction in terms of TCAM space for an encoded classifier and at least three times more space reduction in terms of TCAM space for a reencoded classifier and its transformers. This, in turn, leads to improved throughput and decreased power consumption.
更多查看译文
关键词
Associative memory,Energy consumption,Virtual private networks,Network address translation,Quality of service,Hardware,Power generation,Color,Art,Transformers
AI 理解论文
溯源树
样例
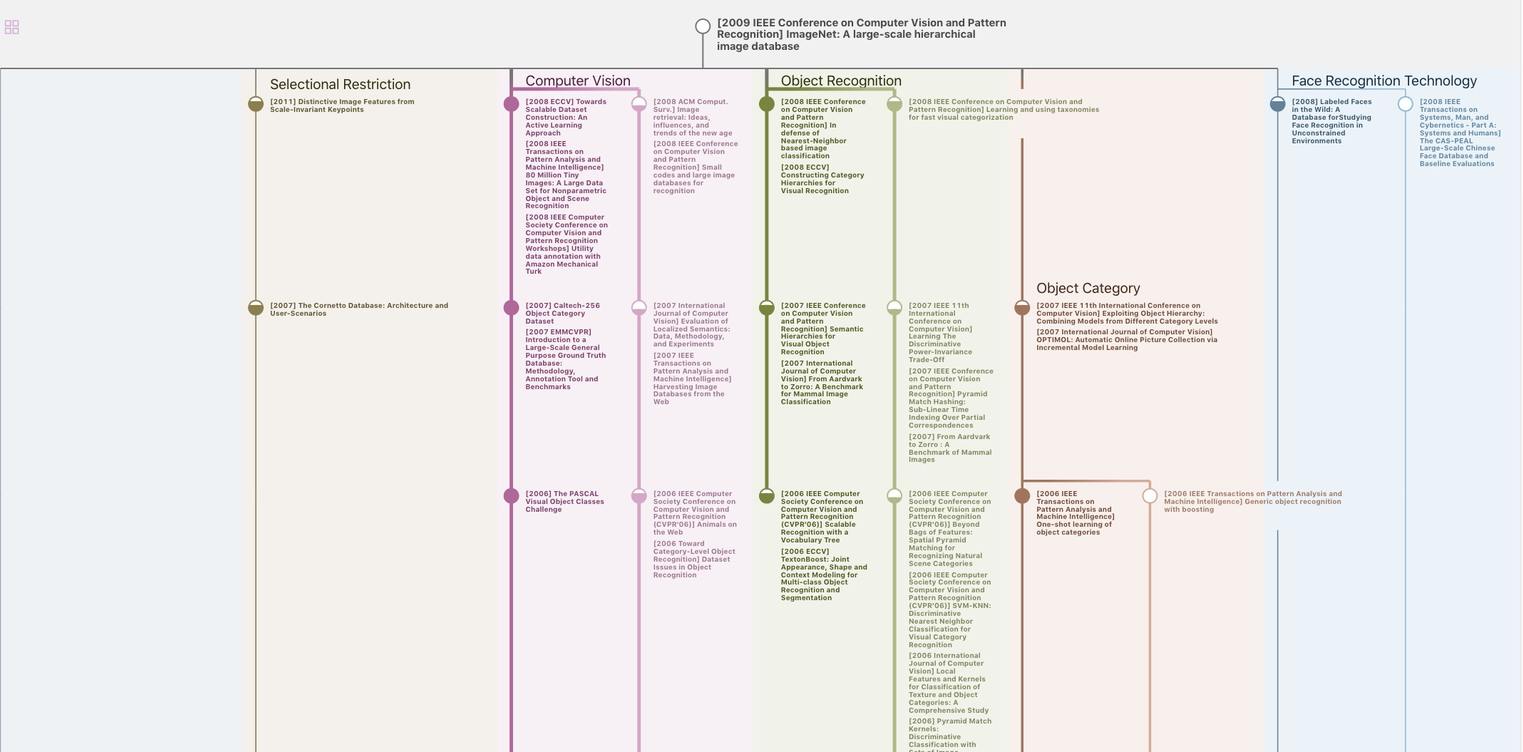
生成溯源树,研究论文发展脉络
Chat Paper
正在生成论文摘要