Web-based keyword adapted Language Modeling for Keyword Spotting
ISCSLP(2010)
摘要
Language Model (LM) constitutes one of the key components in Keyword Spotting (KWS). The rapid development of the World Wide Web (WWW) makes it an extremely large and valuable data source for LM training, but it is not optimal to use the raw transcripts from WWW due to the mismatch of content between the web corpus and the test data. This paper proposes a novel two-step data selection method based on the predefined keyword list in language modeling for keyword spotting. First we exploit the keywords to be spotted, by submitting every keyword as a independent search engine query, it retrieves web corpus that can be used directly to train a web LM (However we didn't); Second we select the sentences with the predefined keywords from the raw web corpus. The final keyword-specific corpus selected is applied to train adaptive LM used to adapt general purpose one. Our keyword-specific LM allows the KWS task to be topic-independent, allowing the keywords to be random and irrelevant. Our experimental results show that the keyword-specific LM outperforms the one trained on the raw web corpus, while expanding the size of the web-based data corpus no longer improve the EER point of the KWS system, but improve the performance on both end of the DET (Detection Error Tradeoff) curve.
更多查看译文
关键词
mixture language model,world wide web,web corpus,data selection,web based keyword adapted language modeling,detection error tradeoff curve,two-step data selection method,predefined keyword list,web sites,internet,keyword spotting,keyword specific corpus,text analysis,search engines,search engine query,query processing,search engine,training data,acoustics,data models,language model,web pages
AI 理解论文
溯源树
样例
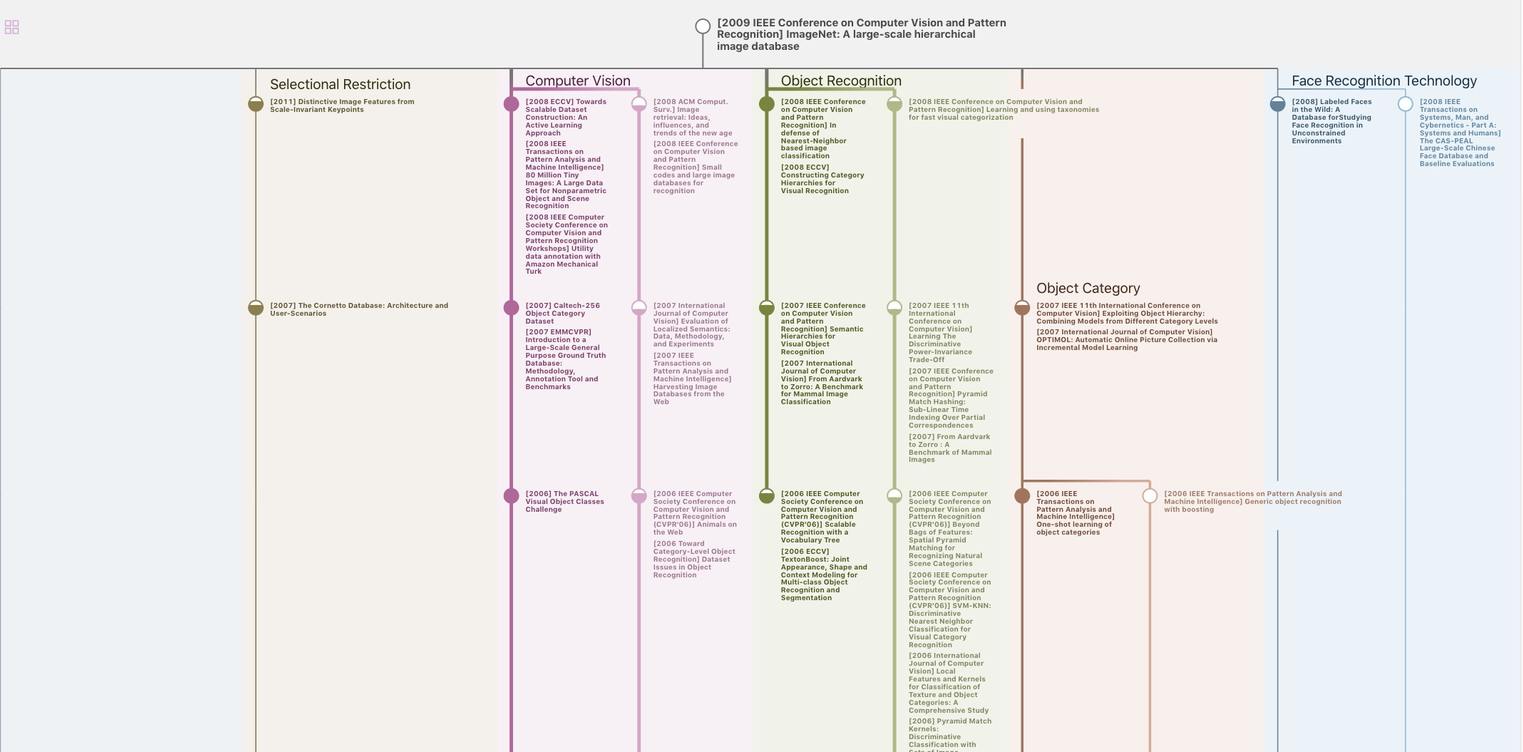
生成溯源树,研究论文发展脉络
Chat Paper
正在生成论文摘要