Statistical Inference in Hidden Markov Models Using k -Segment Constraints.
JOURNAL OF THE AMERICAN STATISTICAL ASSOCIATION(2016)
摘要
Hidden Markov models (HMMs) are one of the most widely used statistical methods for analyzing sequence data. However, the reporting of output from HMMs has largely been restricted to the presentation of the most-probable (MAP) hidden state sequence, found via the Viterbi algorithm, or the sequence of most probable marginals using the forward-backward algorithm. In this article, we expand the amount of information we could obtain from the posterior distribution of an HMM by introducing linear-time dynamic programming recursions that, conditional on a user-specified constraint in the number of segments, allow us to (i) find MAP sequences, (ii) compute posterior probabilities, and (iii) simulate sample paths. We collectively call these recursions -segment algorithms and illustrate their utility using simulated and real examples. We also highlight the prospective and retrospective use of -segment constraints for fitting HMMs or exploring existing model fits. Supplementary materials for this article are available online.
更多查看译文
关键词
Dynamic programming,Hidden Markov models,Segmentation.
AI 理解论文
溯源树
样例
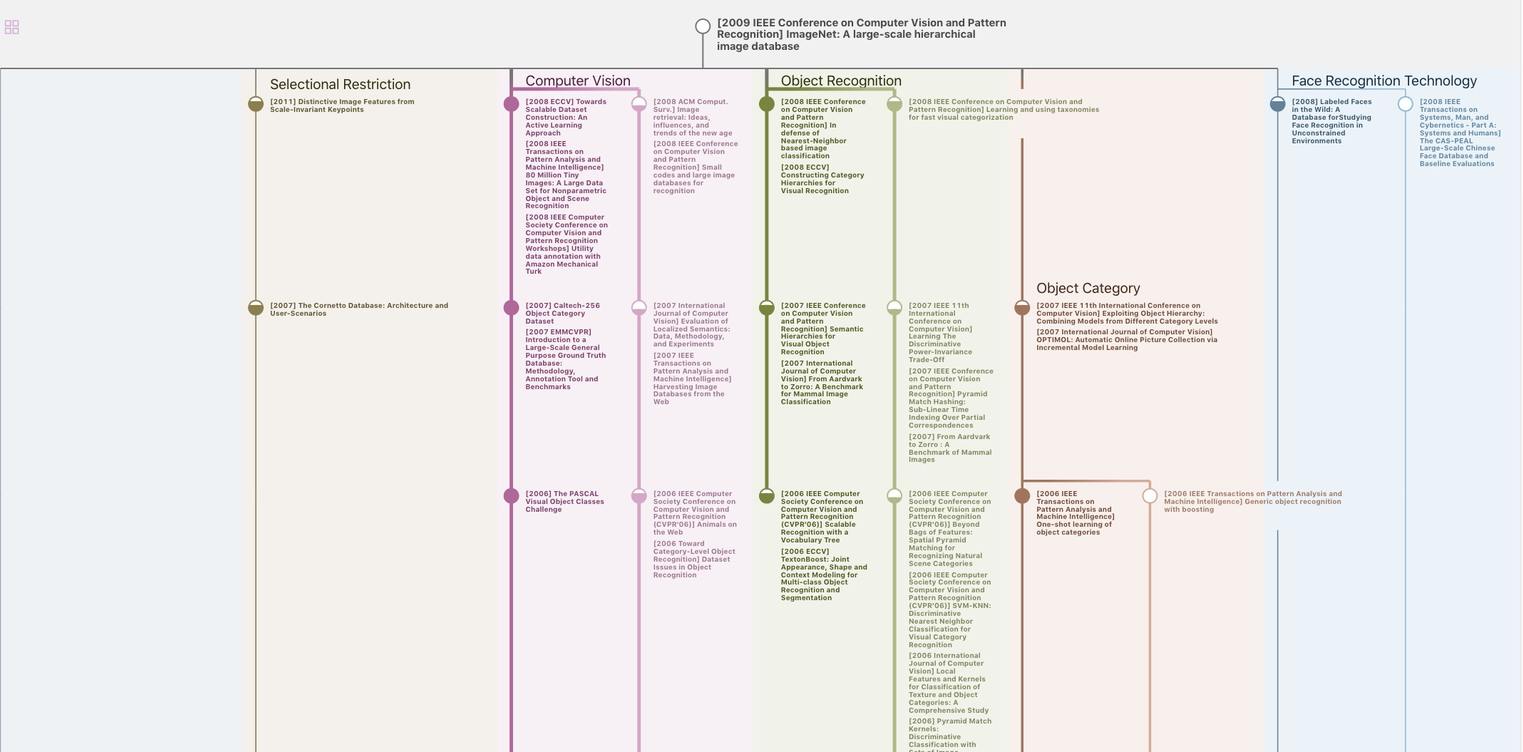
生成溯源树,研究论文发展脉络
Chat Paper
正在生成论文摘要