BM: An iterative algorithm to learn stable non-linear dynamical systems with Gaussian mixture models
ICRA(2010)
摘要
We model the dynamics of non-linear point-to- point robot motions as a time-independent system described by an autonomous dynamical system (DS). We propose an iterative algorithm to estimate the form of the DS through a mixture of Gaussian distributions. We prove that the resulting model is asymptotically stable at the target. We validate the accuracy of the model on a library of 2D human motions and to learn a control policy through human demonstrations for two multi- degrees of freedom robots. We show the real-time adaptation to perturbations of the learned model when controlling the two kinematically-driven robots. motion to parts of space not seen before, and can immediately adapt to spatial perturbations. We first show that though existing regression techniques can be used to estimate the underlying ODE, they fail to handle the classical problem of ODE functions that has been posed repeatedly in the field of dynamics, i.e. stability. Therefore, we propose a learning procedure, called Binary Merging (BM), that tackles the problem of estimating (identifying) an unknown non-linear dynamical system from a few demonstrations while ensuring its global stability.
更多查看译文
关键词
Gaussian distribution,iterative methods,learning systems,motion control,nonlinear dynamical systems,perturbation techniques,robot dynamics,robot kinematics,stability,Gaussian distribution,Gaussian mixture models,autonomous dynamical system,binary merging,iterative algorithm,kinematically-driven robot,learned model perturbation,multidegrees of freedom robots,nonlinear point-to-point robot motion dynamics,stable nonlinear dynamical system learning,time-independent system
AI 理解论文
溯源树
样例
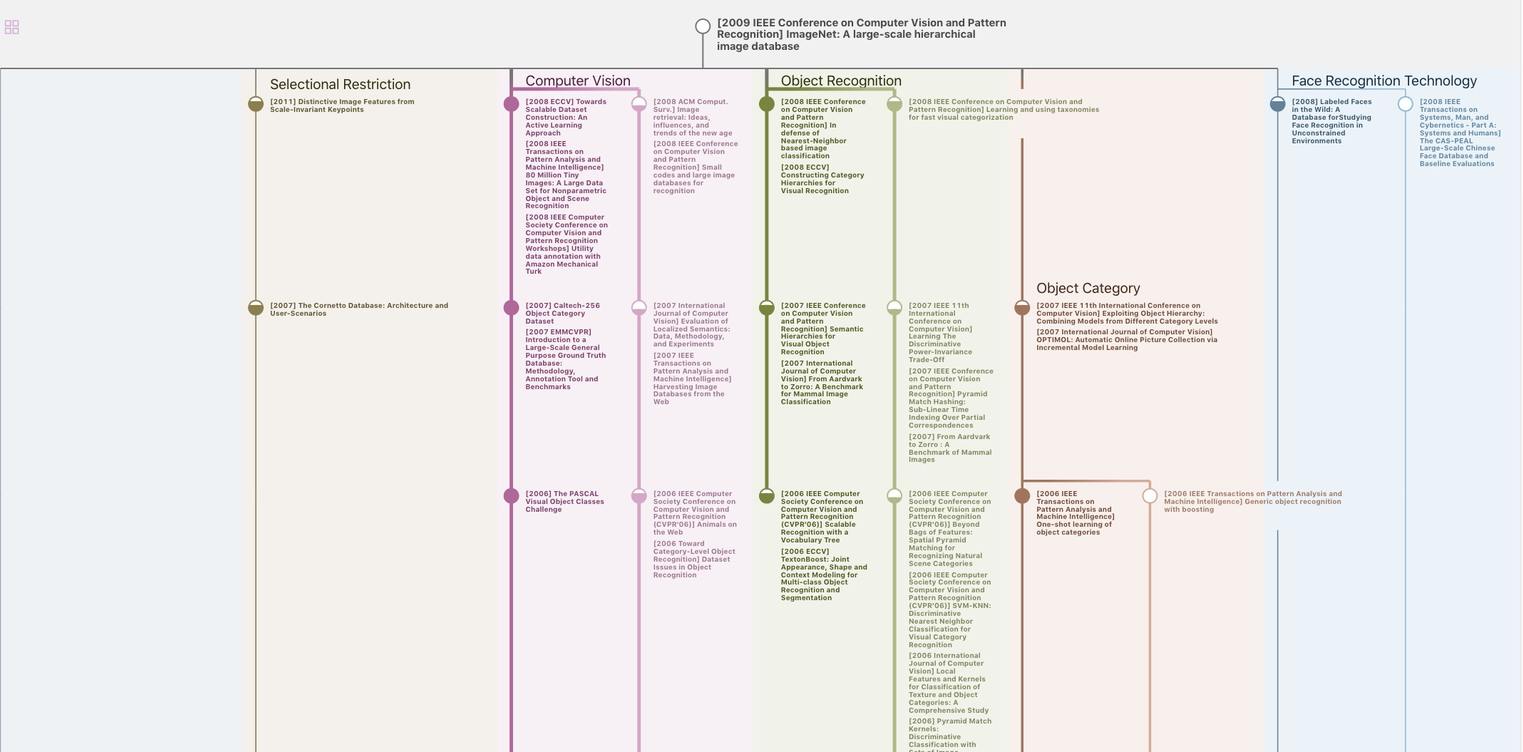
生成溯源树,研究论文发展脉络
Chat Paper
正在生成论文摘要