Review of MIR-Max Algorithm and Potential Improvements
ICIII '11 Proceedings of the 2011 International Conference on Information Management, Innovation Management and Industrial Engineering - Volume 01(2011)
摘要
This paper discusses in depth the different parts of the MIR-max clustering algorithm with respect to the problem of diagnosing river quality. An equivalent information theoretic measure is proposed in this paper for clustering which is based on conditional entropy. The original mutual information method of clustering is compared with the proposed conditional entropy of states given to the cluster. This information theoretic concept measures the quality of cluster in terms of uncertainty existing within a cluster. It is found that the measure of conditional entropy is also useful for quantifying the 'fit' of a new sample in a cluster. Indifferent mutual information is also described in the paper. Numeric examples are provided in this paper regarding the feasibility of the proposed measure for the clustering algorithm.
更多查看译文
关键词
entropy,environmental science computing,optimisation,pattern clustering,regression analysis,rivers,MIR-max clustering algorithm,conditional entropy,information theoretic measure,river quality diagnosis,Biological Monitoring,Clustering,Conditional Entropy,Information & Regression-maximization,Mutual Information,
AI 理解论文
溯源树
样例
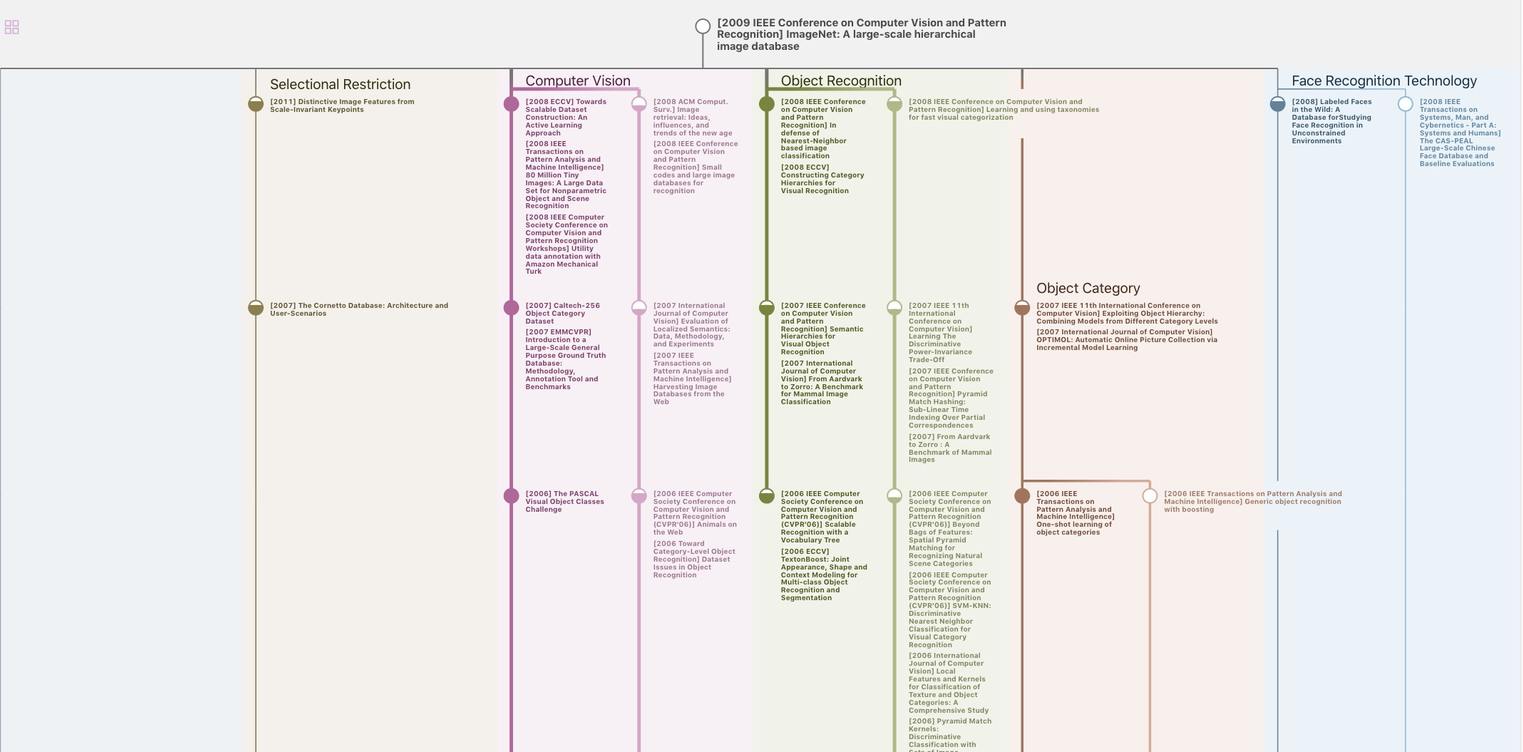
生成溯源树,研究论文发展脉络
Chat Paper
正在生成论文摘要