IMPLICIT ARGUMENTATION IN NOMINAL SEMANTIC ROLE LABELING
msra(2009)
摘要
ABSTRACT IMPLICIT ARGUMENTATION IN NOMINAL SEMANTIC ROLE LABELING By Matthew Gerber Semantic role labeling is a method of identifying predicates and their arguments within natural language. Typically, these predicates denote events and the argu- ments denote event participants. Thus, SRL research has implications for a wide variety of natural language processing (NLP) sub-fields, including question answer- ing, information extraction, and summarization. Traditionally, SRL research has focused on the analysis of verbs due to their strong connection with event descrip- tions. In contrast, this report focuses on emerging topics in noun-based (or nominal) SRL. One key difference between nominal and verbal SRL is that nominals exhibit a high degree of implicit argumentation. Despite its prevalence, implicit argumentation has not been systematically investigated in previous SRL research. Most studies use an evaluation methodology in which SRL systems are tested over predicates that are known to take explicit arguments. For verbal SRL, this approach is not problematic because it is quite rare to observe a verb without arguments. However, our studies have shown that implicit argumentation presents a significant challenge to nominal SRL systems: after introducing implicit argumentation into the evaluation, our state- of-the-art nominal SRL system suffers a performance degradation of more than 9% (Gerber et al., 2009). Motivated by the observations above, this report focuses specifically on implicit argumentation in nominal SRL. Our initial investigations have shown that the afore- mentioned 9% performance degradation can be reduced with a discriminative clas- sifier capable of filtering out nominals whose arguments are implicit. This approach improves performance by approximately 5% - an encouraging result, but one that leaves much to be desired. Our analyses show that implicit arguments constitute a large portion of the predicate-argument structure of a document, but the filter-based nominal SRL system makes no attempt to identify them. In order to maximize the usefulness of SRL analyses for downstream processing,
更多查看译文
关键词
information extraction,semantic role labeling,natural language,noun,natural language processing
AI 理解论文
溯源树
样例
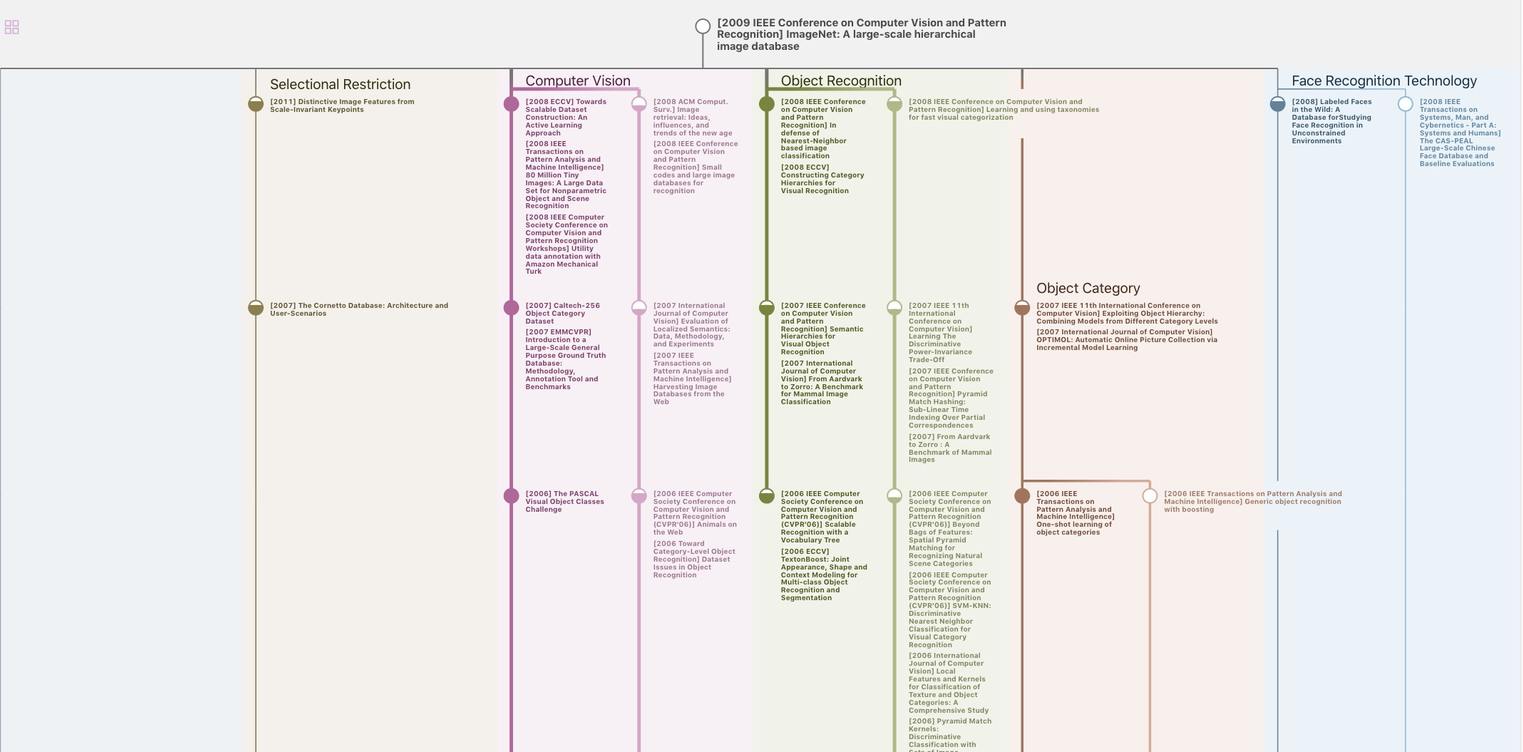
生成溯源树,研究论文发展脉络
Chat Paper
正在生成论文摘要