Empirical Evaluation of Missing Data Techniques for Effort Estimation
msra(2008)
摘要
Multivariate regression models have been commonly used to estimate the software development effort to assist project planning and/or management. These models require a complete data set that has no missing values for model construction. The complete data set is usually built either by using imputation methods or by deleting projects and/or metrics that have missing values (we call this RC deletion). However, it is unclear which method is the most suitable for the effort estimation. In this paper, using the ISBSG data set of 706 projects (containing 47% missing values) collected from several companies, we applied four imputation methods (mean imputation, pairwise deletion, k-NN method and CF method) and RC deletion to build regression models. Then, using a data set of 143 projects (with no missing values), we evaluated the estimation performance of models after applying each imputation or the RC deletion. The result showed that the similarity-based imputation method (k-NN method and CF method) showed better performance than other methods in terms of MdMAE, MdMRE, MdMER and Pred(25).
更多查看译文
AI 理解论文
溯源树
样例
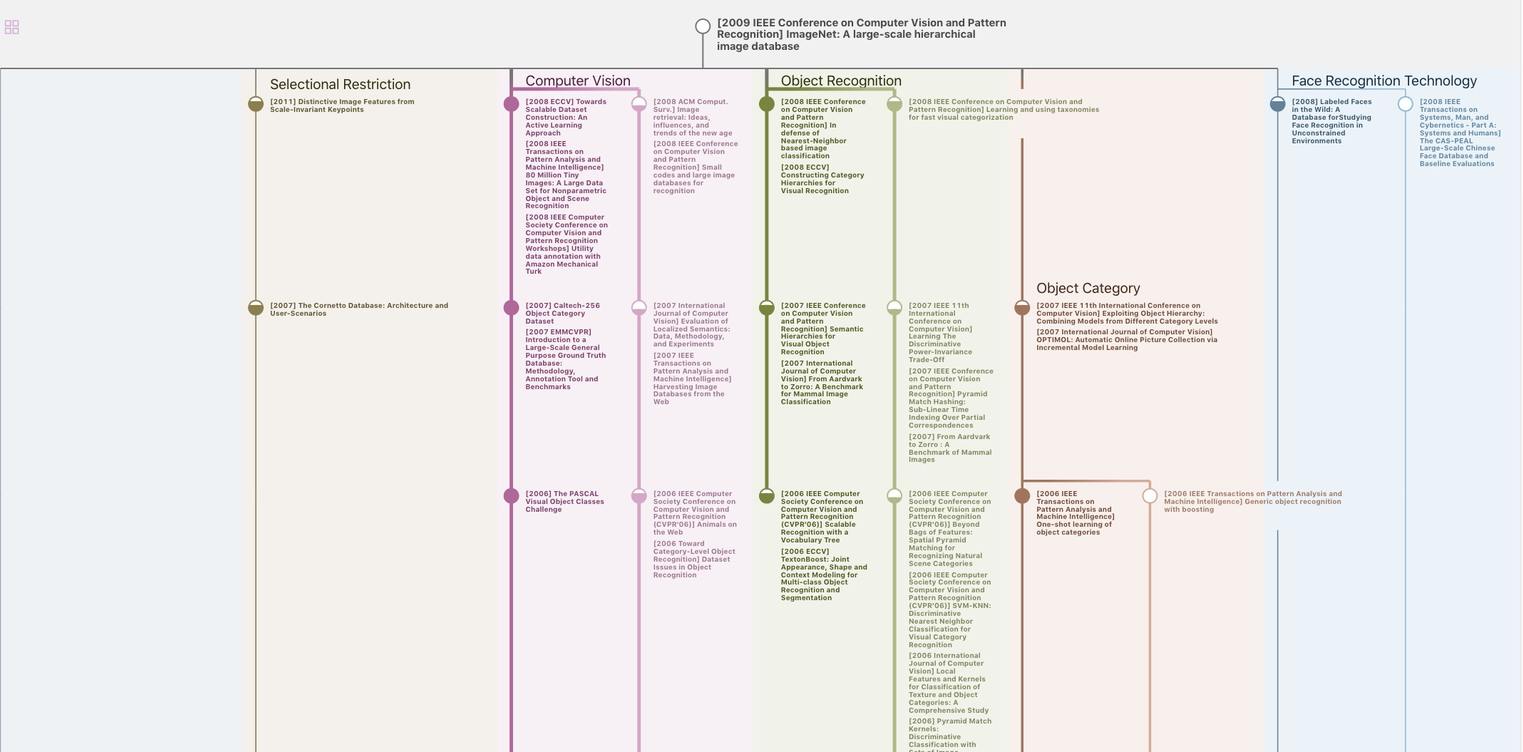
生成溯源树,研究论文发展脉络
Chat Paper
正在生成论文摘要