Scalable Anomaly Detection in Large Homogenous Populations.
Automatica(2014)
摘要
Anomaly detection in large populations is a challenging but highly relevant problem. It is essentially a multi-hypothesis problem, with a hypothesis for every division of the systems into normal and anomalous systems. The number of hypothesis grows rapidly with the number of systems and approximate solutions become a necessity for any problem of practical interest. In this paper we take an optimization approach to this multi-hypothesis problem. It is first shown to be equivalent to a non-convex combinatorial optimization problem and then is relaxed to a convex optimization problem that can be solved distributively on the systems and that stays computationally tractable as the number of systems increase. An interesting property of the proposed method is that it can under certain conditions be shown to give exactly the same result as the combinatorial multi-hypothesis problem and the relaxation is hence tight.
更多查看译文
关键词
Anomaly detection,Outlier detection,Multi-hypothesis testing,Distributed optimization,System identification
AI 理解论文
溯源树
样例
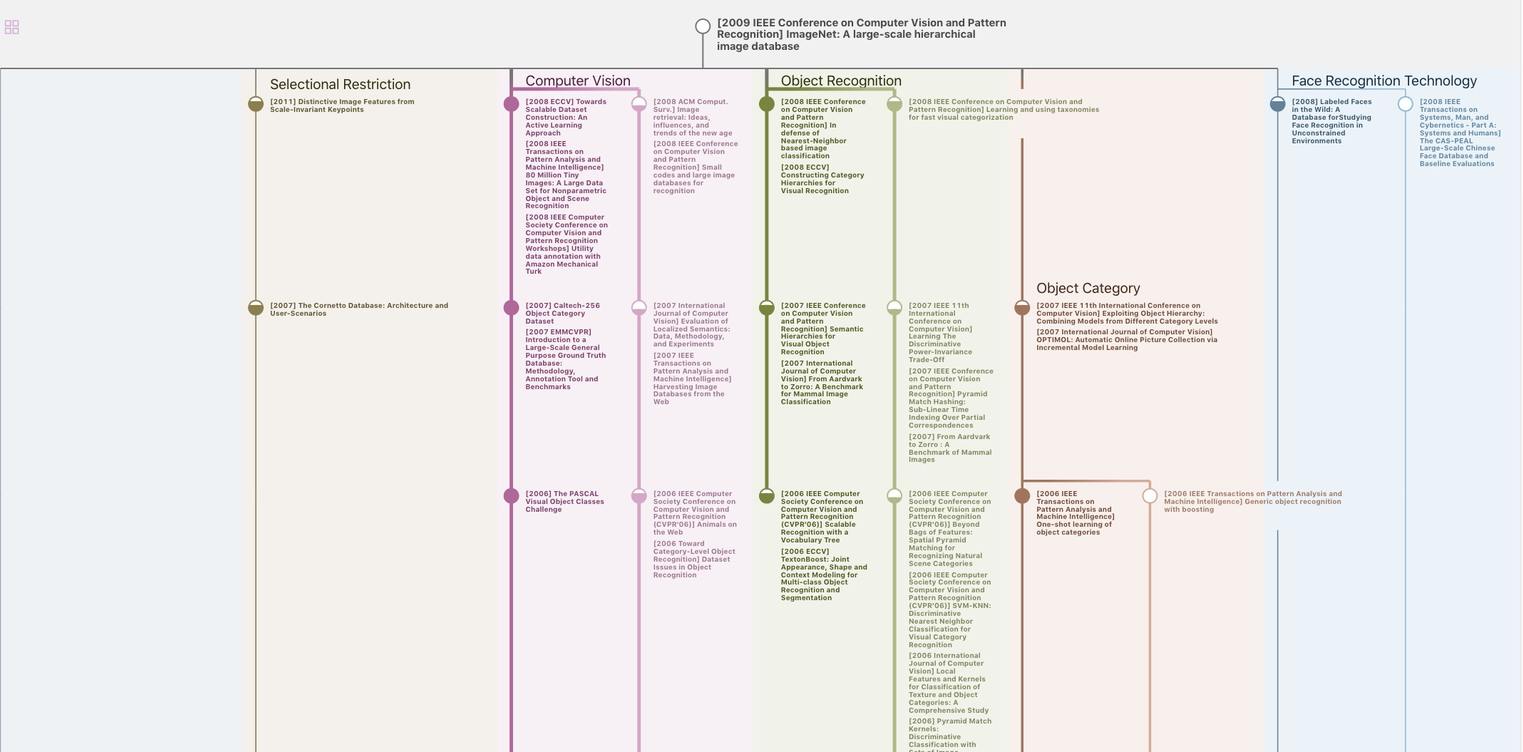
生成溯源树,研究论文发展脉络
Chat Paper
正在生成论文摘要