Profiling linked open data with ProLOD
ICDE Workshops(2010)
摘要
Linked open data (LOD), as provided by a quickly growing number of sources constitutes a wealth of easily accessible information. However, this data is not easy to understand. It is usually provided as a set of (RDF) triples, often enough in the form of enormous files covering many domains. What is more, the data usually has a loose structure when it is derived from end-user generated sources, such as Wikipedia. Finally, the quality of the actual data is also worrisome, because it may be incomplete, poorly formatted, inconsistent, etc.To understand and profile such linked open data, traditional data profiling methods do not suffice. With ProLOD, we propose a suite of methods ranging from the domain level (clustering, labeling), via the schema level (matching, disambiguation), to the data level (data type detection, pattern detection, value distribution). Packaged into an interactive, web-based tool, they allow iterative exploration and discovery of new LOD sources. Thus, users can quickly gauge the relevance of the source for the problem at hand (e.g., some integration task), focus on and explore the relevant subset.
更多查看译文
关键词
iterative methods,meta data,LOD,ProLOD,RDF,Web based tool,data profiling methods,data type detection,iterative exploration,linked open data,pattern detection,profiling linked open data,value distribution
AI 理解论文
溯源树
样例
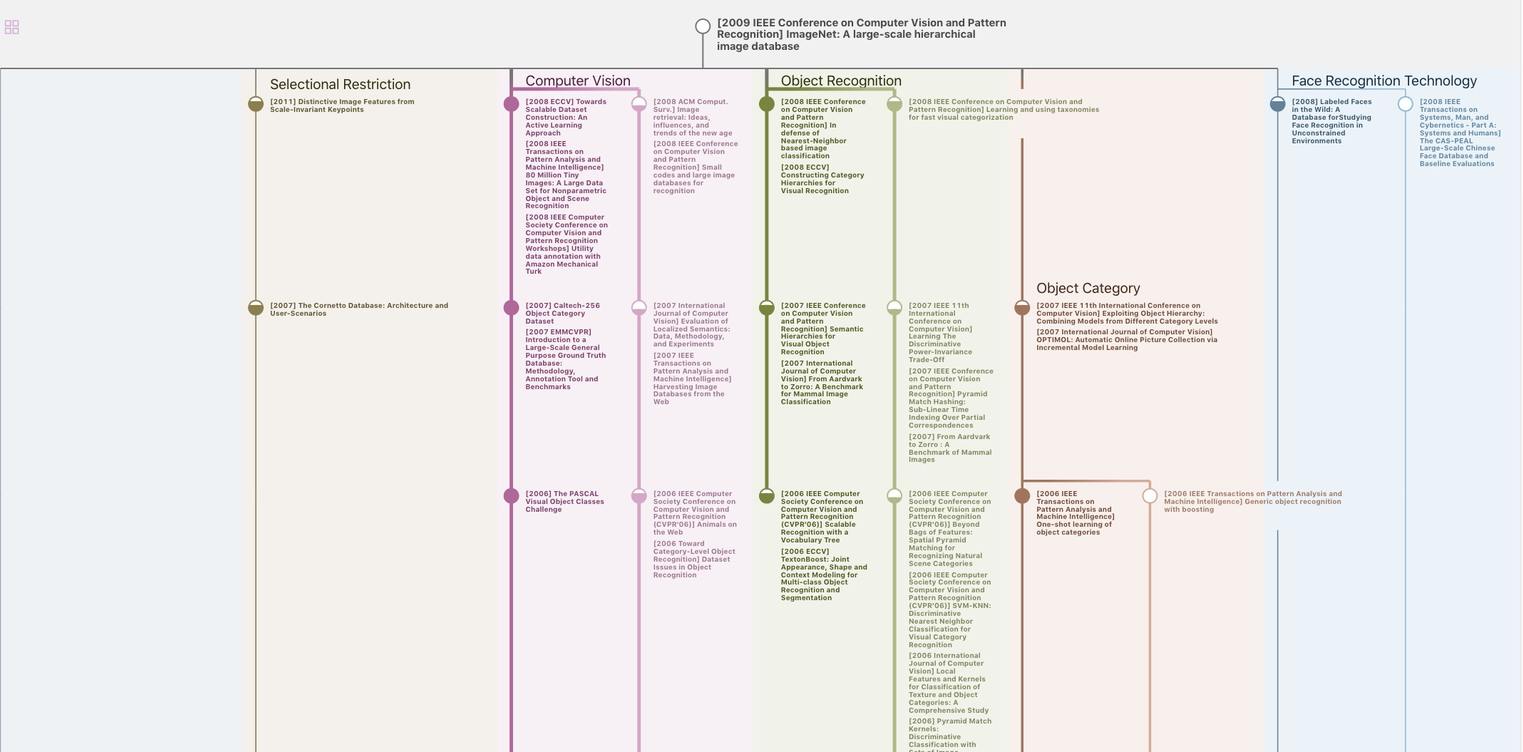
生成溯源树,研究论文发展脉络
Chat Paper
正在生成论文摘要