Learning environmental knowledge from task-based human-robot dialog.
ICRA(2013)
摘要
This paper presents an approach for learning environmental knowledge from task-based human-robot dialog. Previous approaches to dialog use domain knowledge to constrain the types of language people are likely to use. In contrast, by introducing a joint probabilistic model over speech, the resulting semantic parse and the mapping from each element of the parse to a physical entity in the building (e. g., grounding), our approach is flexible to the ways that untrained people interact with robots, is robust to speech to text errors and is able to learn referring expressions for physical locations in a map (e. g., to create a semantic map). Our approach has been evaluated by having untrained people interact with a service robot. Starting with an empty semantic map, our approach is able ask 50% fewer questions than a baseline approach, thereby enabling more effective and intuitive human robot dialog.
更多查看译文
关键词
control engineering computing,human-robot interaction,interactive systems,learning (artificial intelligence),mobile robots,probability,semantic networks,service robots,speech synthesis,domain knowledge,empty semantic map,joint probabilistic model,learning environmental knowledge,physical entity,semantic parse,service robot,speech to text errors,task-based human-robot dialog,untrained people
AI 理解论文
溯源树
样例
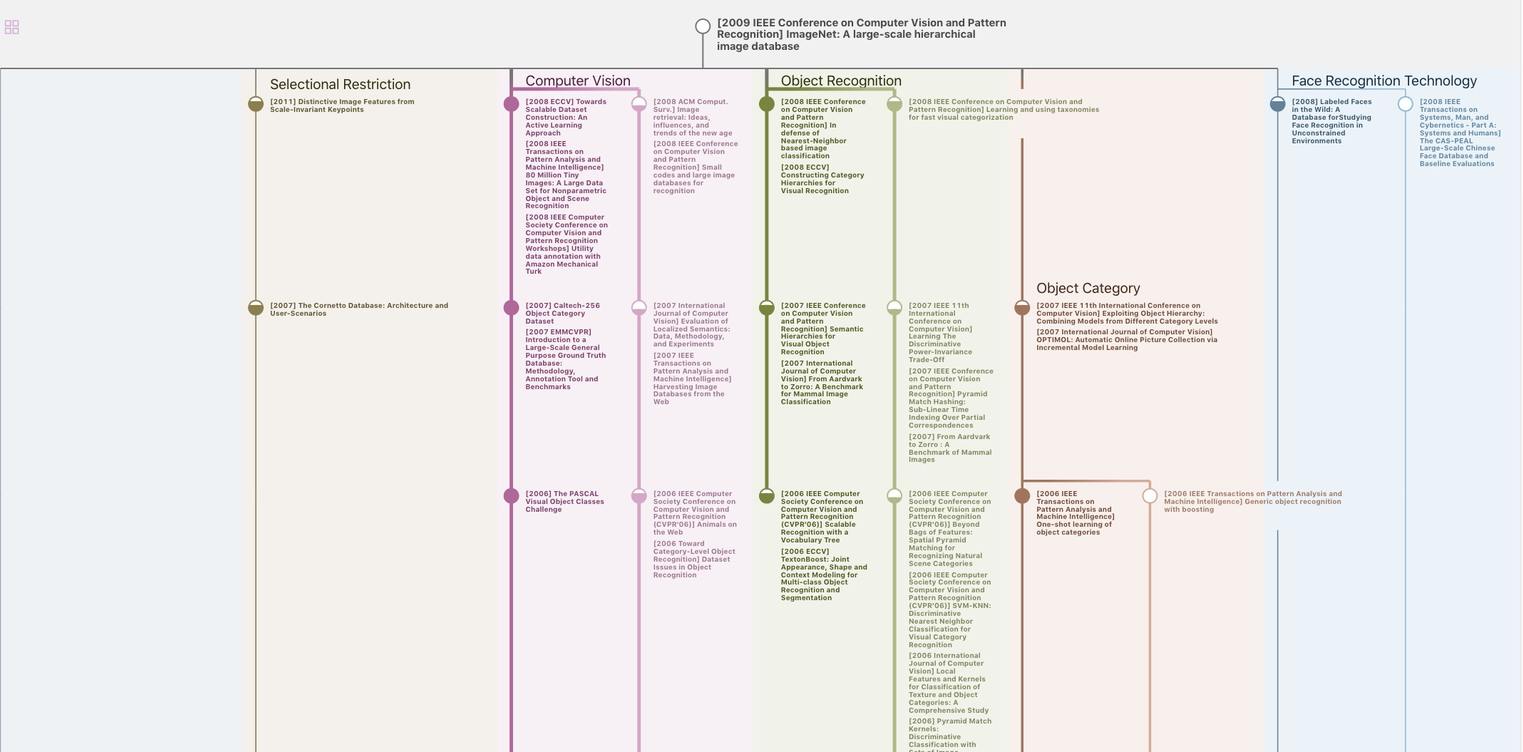
生成溯源树,研究论文发展脉络
Chat Paper
正在生成论文摘要