What and Where: A Bayesian inference theory of visual attention
What and where: a bayesian inference theory of visual attention(2010)
摘要
In the theoretical framework described in this thesis, attention is part of the inference process that solves the visual recognition problem of what is where. The theory proposes a computational role for attention and leads to a model that predicts some of its main properties at the level of psychophysics and physiology. In our approach, the main goal of the visual system is to infer the identity and the position of objects in visual scenes: spatial attention emerges as a strategy to reduce the uncertainty in shape information while feature-based attention reduces the uncertainty in spatial information. Featural and spatial attention represent two distinct modes of a computational process solving the problem of recognizing and localizing objects, especially in difficult recognition tasks such as in cluttered natural scenes. We describe a specific computational model and relate it to the known functional anatomy of attention. We show that several well-known attentional phenomena—including bottom-up pop-out effects, multiplicative modulation of neuronal tuning curves and shift in contrast responses—emerge naturally as predictions of the model. We also show that the bayesian model predicts well human eye fixations (considered as a proxy for shifts of attention) in natural scenes. Finally, we demonstrate that the same model, used to modulate information in an existing feedforward model of the ventral stream, improves its object recognition performance in clutter. (Copies available exclusively from MIT Libraries, Rm. 14-0551, Cambridge, MA 02139-4307. Ph. 617-253-5668; Fax 617-253-1690.)
更多查看译文
关键词
computational role,shape information,existing feedforward model,computational process,object recognition performance,bayesian inference theory,bayesian model,spatial attention,visual attention,specific computational model,feature-based attention,difficult recognition task
AI 理解论文
溯源树
样例
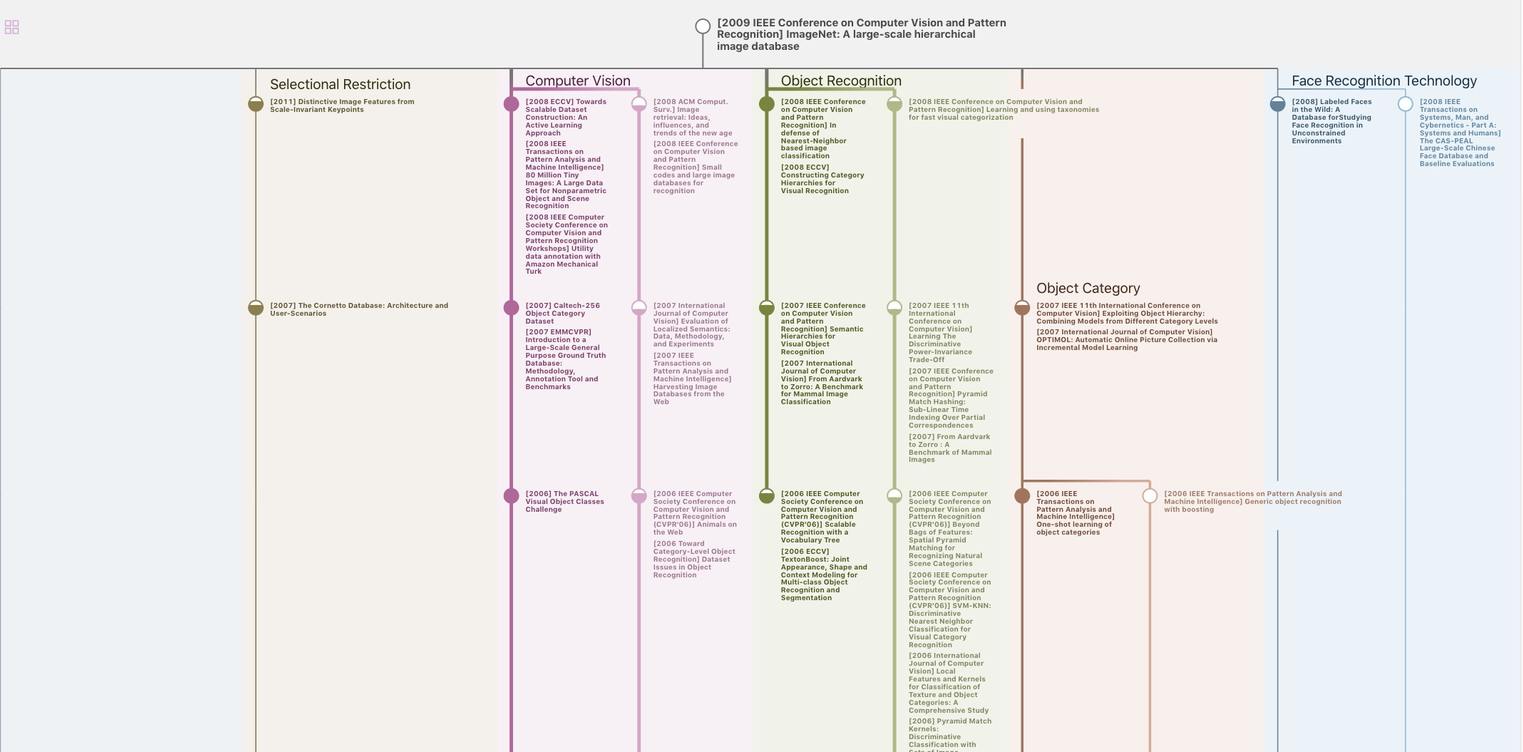
生成溯源树,研究论文发展脉络
Chat Paper
正在生成论文摘要