Towards Learning Human-Robot Dialogue Policies Combining Speech and Visual Beliefs
msra(2011)
摘要
We describe an approach for multi-modal dialogue strategy learning combining two sources of uncertainty: speech and gestures.
Our approach represents the state-action space of a reinforcement learning dialogue agent with relational representations
for fast learning, and extends it with belief state variables for dialogue control under uncertainty. Our approach is evaluated,
using simulation, on a robotic spoken dialogue system for an imitation game of arm movements. Preliminary experimental results
show that the joint optimization of speech and visual beliefs results in better overall system performance than treating them
in isolation.
更多查看译文
关键词
Bayesian Network, Markov Decision Process, Gesture Recognition, Belief State, Partially Observable Markov Decision Process
AI 理解论文
溯源树
样例
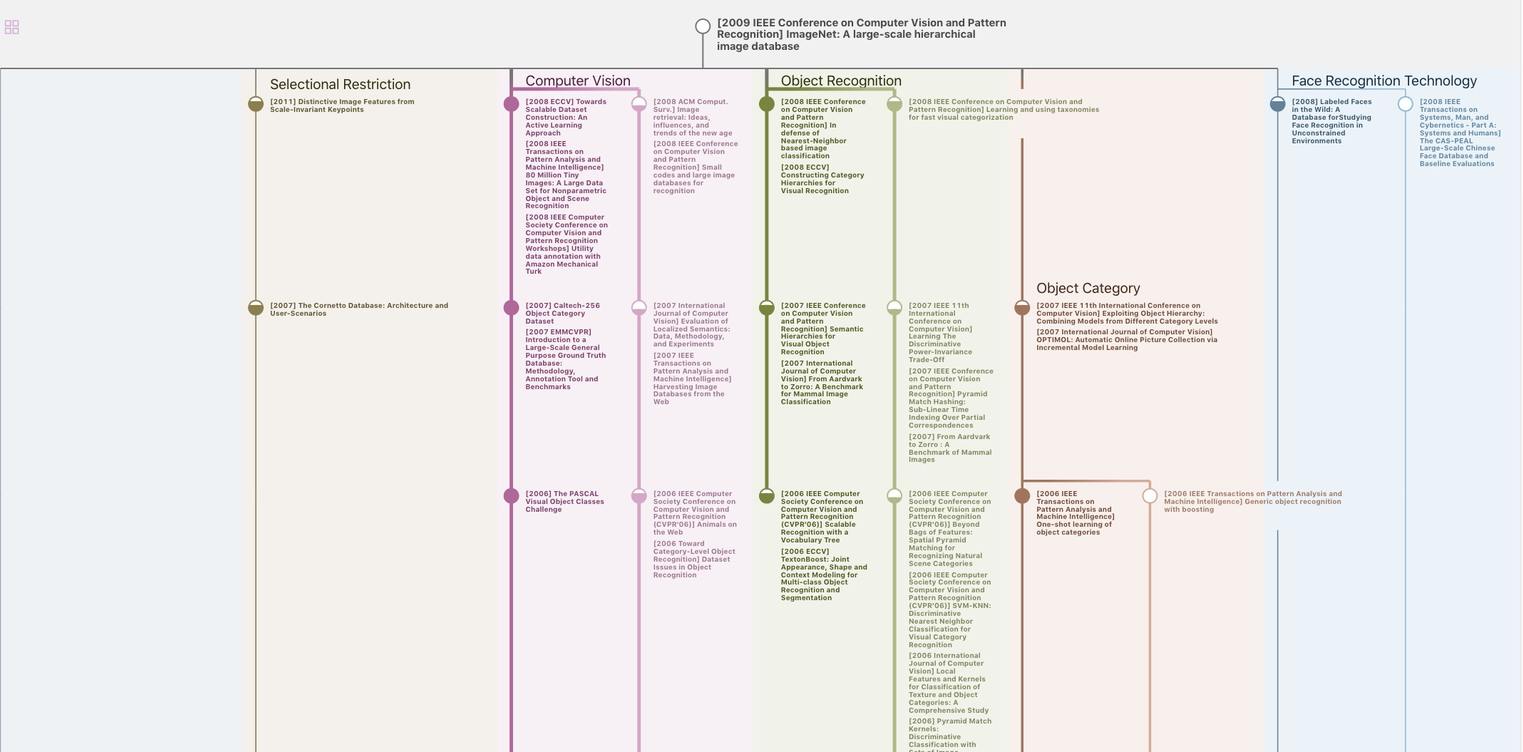
生成溯源树,研究论文发展脉络
Chat Paper
正在生成论文摘要