Unifying Plda And Polynomial Kernel Svms
2013 IEEE INTERNATIONAL CONFERENCE ON ACOUSTICS, SPEECH AND SIGNAL PROCESSING (ICASSP)(2013)
摘要
Probabilistic linear discriminant analysis (PLDA) is a generative model to explain between and within class variations. When the underlying latent variables are modelled by standard Gaussian distributions, the PLDA recognition scores can be evaluated as a dot product between a high dimensional PLDA feature vector and a weight vector. A key contribution of this paper is showing that the high dimensional PLDA feature vectors can be equivalently (in a non-strict sense) represented as the second-degree polynomial kernel induced features of the vectors formed by concatenating the two input vectors constituting a trial. This equivalence relationship paves the way for the speaker recognition problem to be viewed as a two-class support vector machine (SVM) training problem where higher degree polynomial kernels can give better discriminative power. To alleviate the large scale SVM training problem, we propose a kernel evaluation trick that greatly simplifies the kernel evaluation operations. In our experiments, a combination of multiple second degree polynomial kernel SVMs performed comparably to a state-of-the-art PLDA system. For the analysed test case, SVMs trained with third degree polynomial kernel reduced the EERs on average by 10% relative to that of the SVMs trained with second degree polynomial kernel.
更多查看译文
关键词
speaker recognition, large scale SVMs, PLDA
AI 理解论文
溯源树
样例
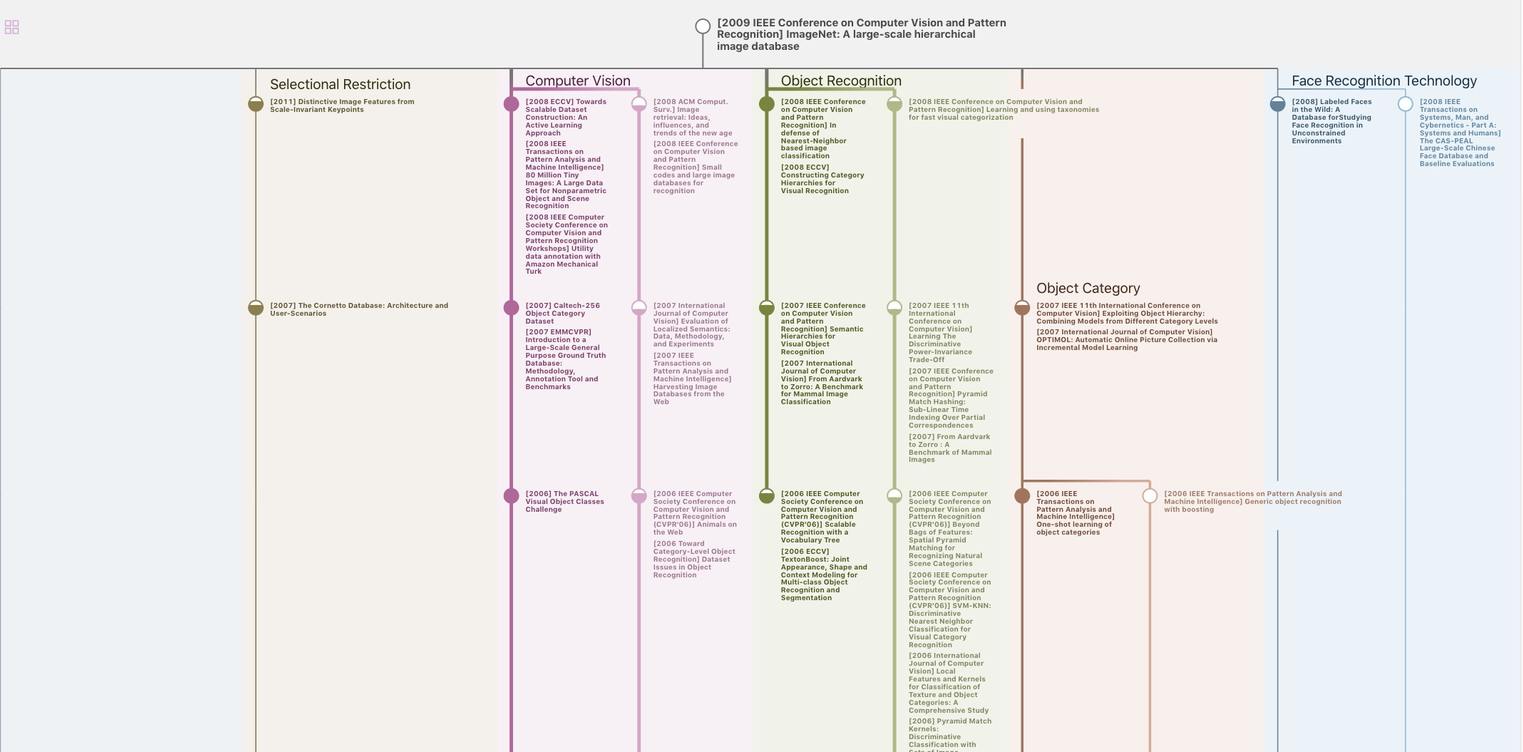
生成溯源树,研究论文发展脉络
Chat Paper
正在生成论文摘要