Selective presentation of peer commentary on Web objects through a modeling of user similarity and reputability: reducing information overload in social networks.
International Conference on User Modeling, Adaptation, and Personalization(2012)
摘要
In this paper, we present an approach aimed at enabling users to enrich their experience with web-based objects (texts or videos). In particular, we consider a social network of users offering commentary on the web objects they have experi- enced together with opinions on the value of this commen- tary being registered by peers. Within this framework, we in- tegrate a reasoner that personalizes the presentation of these annotations to each new user, selectively limiting what is displayed to promote the commentary that will lead to the most effective knowledge gains, based on a modeling of the reputability of the annotator and the similarity of peers who have found this commentary to be useful. We demonstrate the effectiveness of our approach for selective presentation of these web document annotations by constructing a simu- lation of knowledge gains achieved by users. Our method is shown to approach the ideal knowledge gains achieved by an optimal algorithm, far outpacing a system where a random selection of commentary is offered (as might match what users would experience if employing self-directed limiting of browsing behaviour). As a result, we offer an effective method for enhancing the experiences of users in contexts with potentially massive amounts of peer commentary. Indeed, when a social network of peers is involved, a user does eventually need to make decisions about which peers to listen to. In our framework, we allow those peers who have not been helpful in the past to be redeemed and em- braced anew within the social network (through a provision of exploring as well as exploiting the collection of peer com- mentary); in addition, we do not blindly accept the advice that is offered by each respected peer, separately evaluating its worth towards the learning gains to be achieved. As such, we are advocating the use of user models of peers as part of the reasoning of the user who is processing web content. Permission to make digital or hard copies of all or part of this work for personal or classroom use is granted without fee provided that copies are not made or distributed for profit or commercial advantage and that copies bear this notice and the full citation on the first page. To copy otherwise, or republish, to post on servers or to redistribute to lists, requires prior specific permission and/or a fee. CHI 2009, April 4 9, 2009, Boston, Massachusetts, USA. Copyright 2009 ACM 978-1-60558-246-7/09/04...$5.00. In contrast with peer-based intelligent tutoring researchers, we are able to leverage past experiences rather than requir- ing peers to be assisting in realtime with the learning that is achieved. As such, our users are free to browse and view the accompanying annotations on their web documents and videos, at their leisure. This is accomplished by attaching an interaction history (knowledge benefit acquired) from each user to each learning object and continuously selecting those web objects that are poised to deliver the most value the cur- rent user (based on modeling similarity to other peers). From here, the commentary that is selected are the most beneficial ones attached to this particular web object. We are also care- ful to avoid possibly harmful content offered by peers, thus continuing to allow free expression within the social net- work, but achieving a valuable limiting of what is directed to each new user.
更多查看译文
关键词
user similarity,peer commentary,social networks,information overload,web objects
AI 理解论文
溯源树
样例
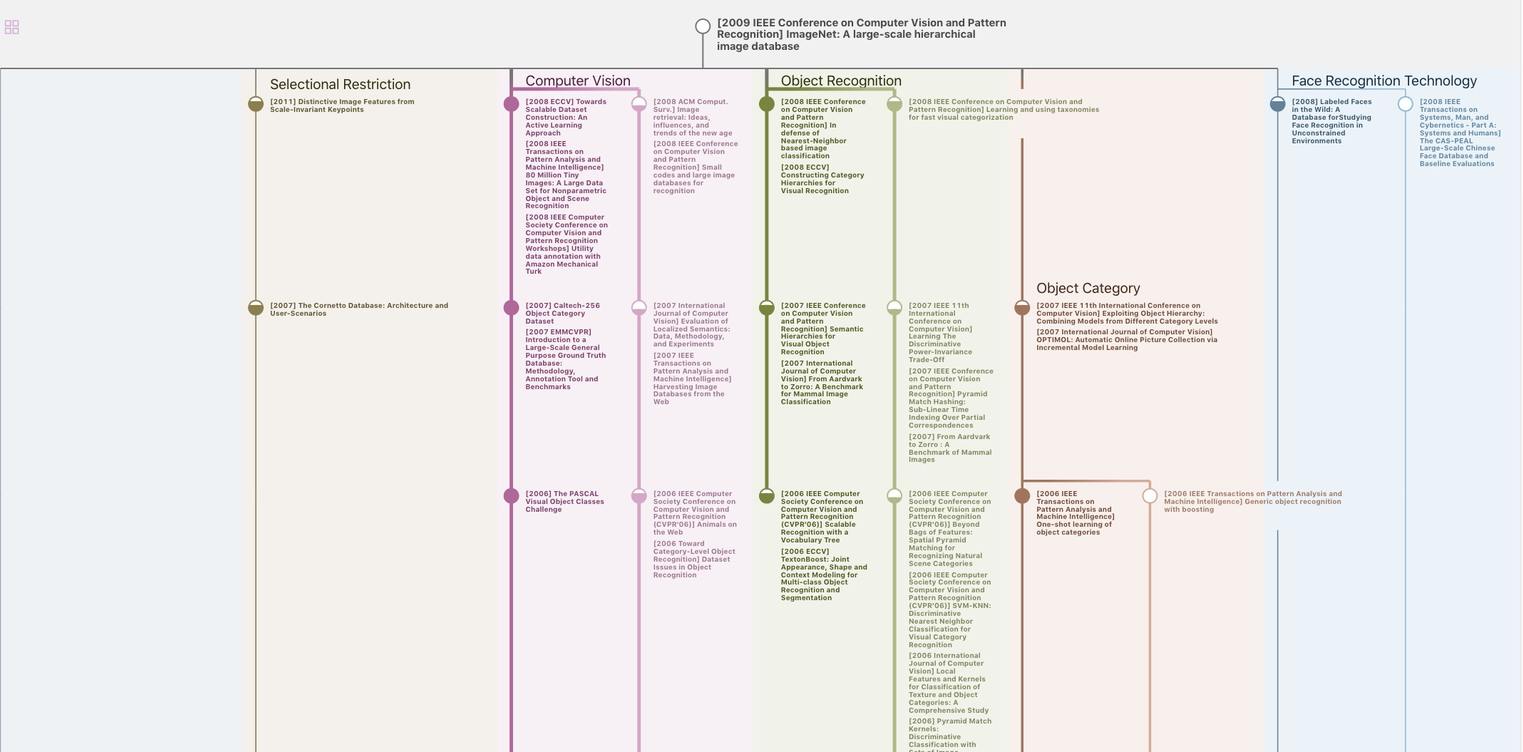
生成溯源树,研究论文发展脉络
Chat Paper
正在生成论文摘要