Minimum Message Length Criterion for Second-Order Polynomial Model Selection Applied to Tropical Cyclone Intensity Forecasting
ADVANCES IN INTELLIGENT DATA ANALYSIS V(2003)
摘要
This paper outlines a body of work that tries to merge polynomial model selection research and tropical cyclone forecasting research. The contributions of the work are four-fold. First, a new criterion based on the Minimum Message Length principle specifically formulated for the task of polynomial model selection up to the second order is presented. Second, a programmed optimisation search algorithm for second-order polynomial models that can be used in conjunction with any model selection. criterion is developed. Third, critical examinations of the differences in performance of the various criteria when applied to artificial vis-a-vis to real tropical cyclone data are conducted. Fourth, a novel strategy which uses a synergy between the new criterion built based on the Minimum Message Length principle and other model selection criteria namely, Minimum Description Length, Corrected Akaike's Information Criterion, Structured Risk Minimization and Stochastic Complexity is proposed. The forecasting model developed using this new automated strategy has better performance than the benchmark models SHIFOR (Statistical HurrIcane FORcasting) [4] and SHIFOR94 [8] which are being used in operation in the Atlantic basin.
更多查看译文
关键词
search algorithm,minimum message length,second order,structural risk minimization,tropical cyclone,model selection,minimum description length
AI 理解论文
溯源树
样例
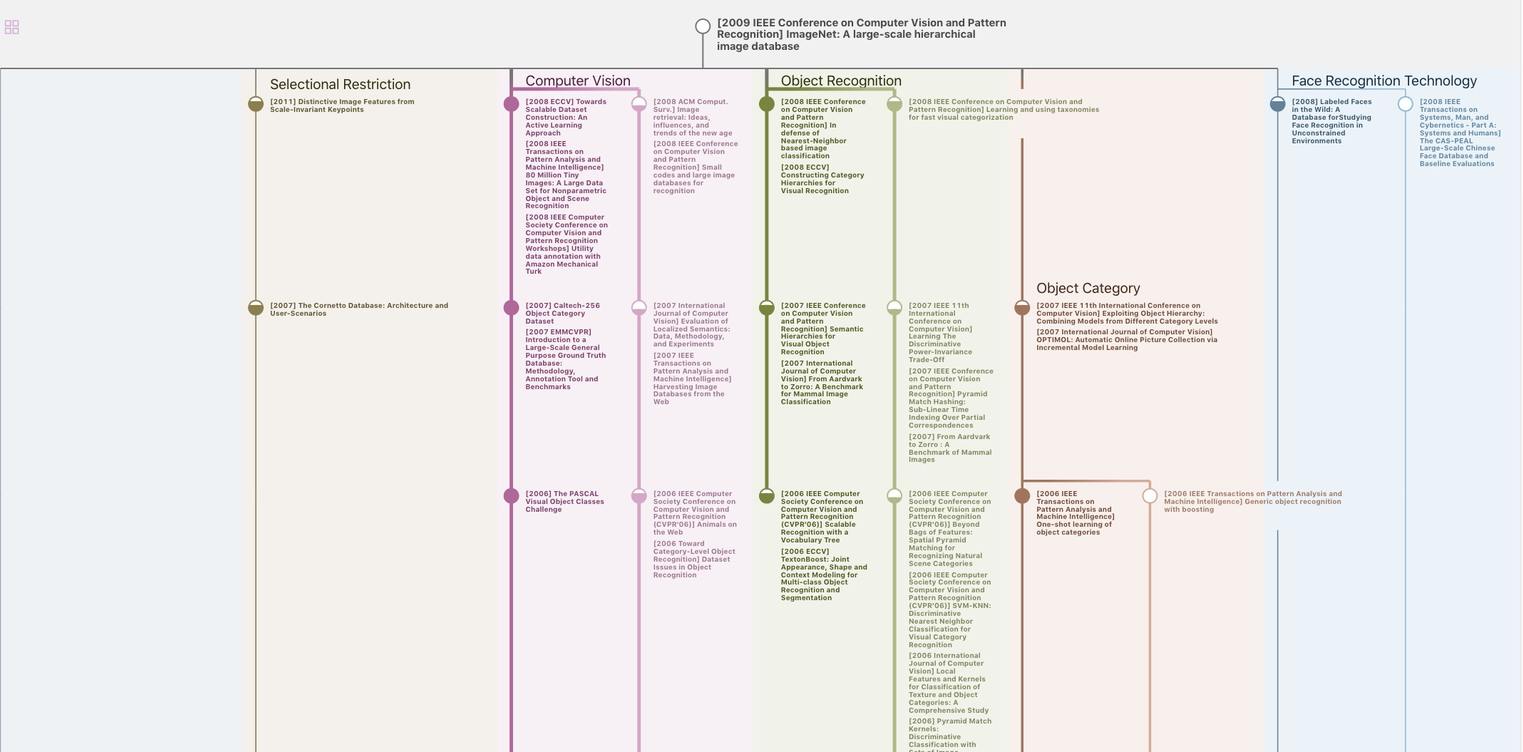
生成溯源树,研究论文发展脉络
Chat Paper
正在生成论文摘要